Why Is Bayesian Statistics Better Than Frequentist
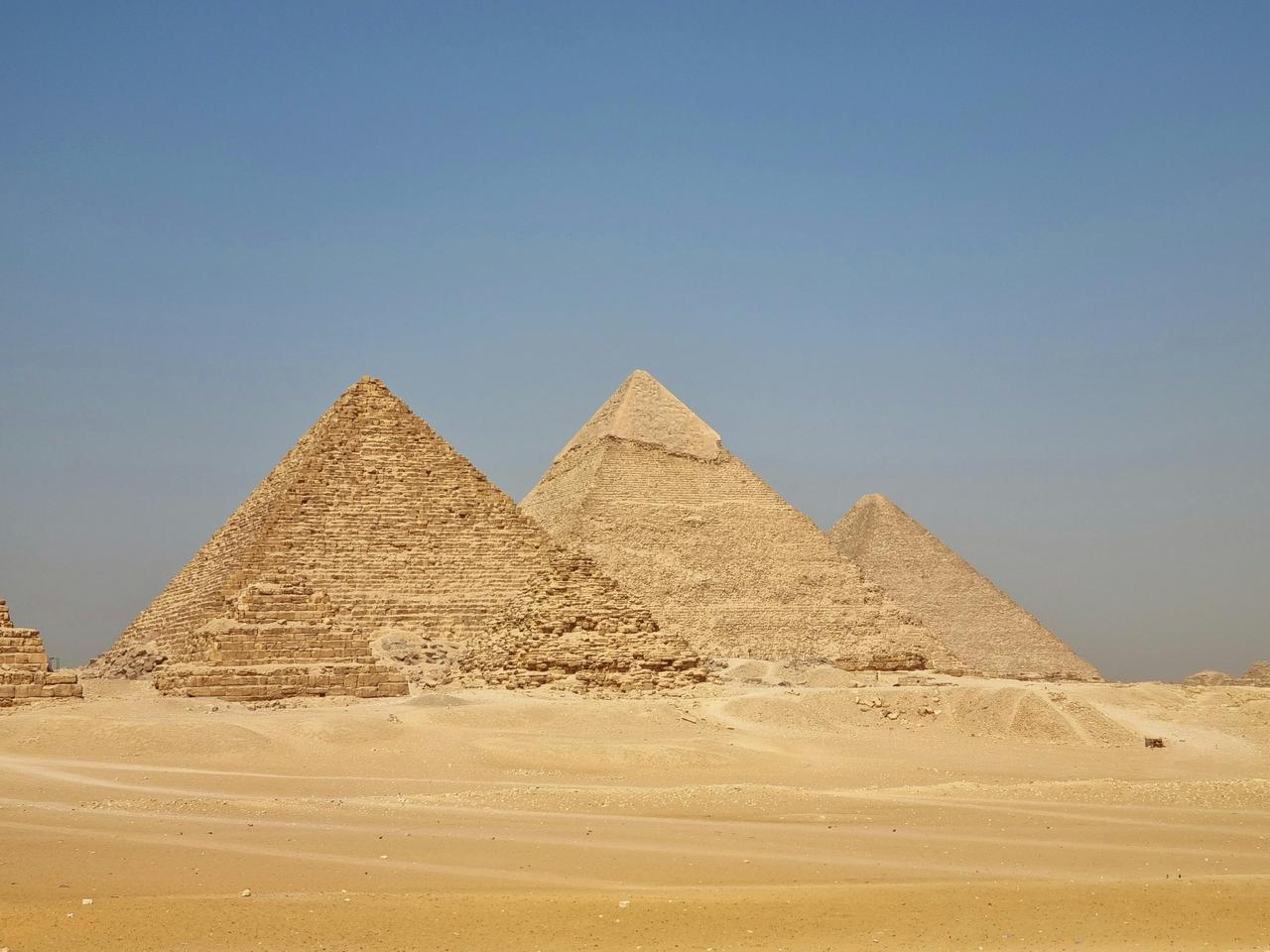
Bayesian Statistics is a powerful approach to data analysis that offers a different perspective compared to traditional methods. Unlike frequentist statistics, which relies on the idea of long-term frequency and objective probability, Bayesian statistics incorporates prior beliefs and updates them as new data becomes available. This iterative process allows for a more flexible and nuanced understanding of data. To delve into the advantages of this method, it’s important to address the question: “Why Is Bayesian Statistics Better Than Frequentist?” Bayesian statistics excels in several areas, such as handling small sample sizes, incorporating prior information, and providing probabilistic interpretations of uncertainty. It allows for the continuous updating of beliefs with each new piece of evidence, making it especially useful in dynamic and uncertain environments. This adaptability and the ability to combine prior knowledge with empirical data give Bayesian statistics a distinctive edge over frequentist approaches, particularly in complex or evolving analytical scenarios.
Incorporating Prior Knowledge
One of the primary advantages of Bayesian statistics is its ability to incorporate prior knowledge or beliefs into the analysis. In Bayesian inference, the prior distribution represents the initial beliefs about a parameter before observing any data. This prior is then updated with the observed data to produce a posterior distribution, which combines the prior information with the new evidence. This process of updating beliefs is mathematically formalized through Bayes’ theorem, which provides a coherent way to revise probabilities as new information becomes available. This feature is especially useful in fields like medicine, where previous studies or expert knowledge can significantly inform the analysis of new data.
Flexibility in Interpretation
Bayesian statistics offers more flexibility in interpretation compared to frequentist methods. For example, Bayesian credible intervals, which represent the range of values within which a parameter lies with a certain probability, are often more straightforward to interpret than frequentist confidence intervals. A 95% credible interval, for instance, directly states that there is a 95% probability that the parameter lies within the specified range, given the data and the prior. In contrast, a frequentist confidence interval only provides a statement about the long-run behavior of the interval under repeated sampling, which can be less intuitive and more difficult to apply in practical decision-making.
Application in Decision-Making
Bayesian methods are particularly well-suited for decision-making processes because they provide a probabilistic framework that reflects uncertainty. In complex problems, Bayesian analysis allows for the continuous updating of models as new data becomes available, leading to more informed and adaptive decisions. This is crucial in dynamic environments, such as finance or clinical trials, where decisions must be made under uncertainty and with evolving information. The Bayesian framework supports a decision-making process that is inherently iterative, allowing for the refinement of decisions as new data emerges.
Key Differences Between Bayesian and Frequentist Approaches
Feature | Bayesian Statistics | Frequentist Statistics |
---|---|---|
Use of Prior Information | Incorporates prior knowledge through priors | Relies solely on data at hand |
Interpretation of Probability | Represents subjective belief or uncertainty | Interprets probability as long-run frequency |
Credible vs. Confidence Intervals | Provides direct probability statements | Based on repeated sampling interpretation |
Flexibility in Decision-Making | Adaptive, iterative approach | More rigid, based on fixed procedures |
Prior Knowledge and Decision Flexibility
“Bayesian statistics excels in incorporating prior knowledge and adapting to new data, making it a superior choice for decision-making in uncertain and dynamic environments compared to the frequentist approach.”
This explanation highlights the strengths of Bayesian statistics, emphasizing its ability to integrate prior information, offer flexible interpretations, and support adaptive decision-making, particularly in fields where uncertainty is a significant factor.
Introduction to Bayesian Statistics
Fundamental Concepts of Bayesian Statistics
Bayesian statistics is a framework for statistical analysis that emphasizes the use of prior knowledge and evidence to update beliefs and make inferences. At the core of Bayesian statistics is Bayes’ Theorem, which provides a mathematical formula to update the probability of a hypothesis based on new data. The key components in Bayesian analysis are the prior distribution (representing initial beliefs), the likelihood (the probability of the observed data given the hypothesis), and the posterior distribution (updated beliefs after considering the new data).
Comparison with Frequentist Approach
In contrast, the frequentist approach to statistics relies on the concept of long-run frequency, interpreting probability as the limiting frequency of an event occurring over repeated trials. Frequentist methods typically involve hypothesis testing, confidence intervals, and point estimates without incorporating prior beliefs or knowledge. This difference leads to distinct approaches in statistical inference, where Bayesian statistics offers a more flexible and adaptive framework, particularly useful in situations where prior information is available or when dealing with complex models.
Historical Development
The origins of Bayesian statistics can be traced back to the 18th century with the work of Reverend Thomas Bayes, who formulated the theorem that bears his name. Over time, Bayesian methods evolved, with significant contributions from statisticians such as Pierre-Simon Laplace, who expanded its application. In recent decades, advancements in computational power and algorithms, like Markov Chain Monte Carlo (MCMC), have revitalized interest in Bayesian methods, making them more accessible for a wide range of applications.
Advantages of Bayesian Statistics
Incorporating Prior Knowledge
One of the most significant advantages of Bayesian statistics is its ability to incorporate prior knowledge into the analysis. This is achieved through the use of prior distributions, which can be informative (based on expert knowledge or previous data) or non-informative (representing a lack of prior information). The prior distribution influences the posterior distribution, allowing for a more nuanced and contextually relevant interpretation of results.
Flexibility in Model Updating
Bayesian statistics excels in its flexibility, particularly in updating models as new data becomes available. This is crucial in fields such as medicine or finance, where new information continuously emerges. Bayesian methods allow for sequential data analysis, where models are dynamically updated, and beliefs are adjusted over time, leading to more accurate and timely inferences.
Handling of Uncertainty
Another key advantage of Bayesian statistics is its robust handling of uncertainty. Unlike frequentist methods, which often rely on fixed confidence intervals, Bayesian methods provide credible intervals that offer a probabilistic interpretation of uncertainty. This approach allows for a more intuitive understanding of the results, making it easier to quantify and manage uncertainty in decision-making processes.
Handling Complex Models and Data
Modeling Flexibility
Bayesian statistics provides significant flexibility in modeling, particularly when dealing with complex or hierarchical models. Bayesian methods can easily accommodate high-dimensional data and intricate dependencies within the data, offering robust and adaptable solutions that are often difficult to achieve with frequentist methods.
Computational Methods and Advances
The advancement of computational methods, such as MCMC and other sampling techniques, has been instrumental in the rise of Bayesian statistics. These methods enable the practical implementation of Bayesian analysis, even for highly complex models. Additionally, a growing array of Bayesian computation tools and software, such as Stan, BUGS, and PyMC, has made Bayesian methods more accessible to researchers and practitioners.
Dealing with Small Sample Sizes
Bayesian statistics is particularly advantageous in scenarios with limited data. By incorporating prior information, Bayesian methods can provide more reliable estimates and inferences even when sample sizes are small. This contrasts with frequentist methods, which often require large samples to produce accurate and robust results.
Practical Applications and Use Cases
Real-World Applications of Bayesian Statistics
Bayesian statistics has found extensive application across various fields:
Medical Research and Clinical Trials: Bayesian models are increasingly used in clinical trials to incorporate prior knowledge and adapt to accumulating data. This approach is beneficial for personalized medicine, where treatments can be tailored based on the evolving understanding of a patient’s condition.
Financial and Economic Forecasting: In finance, Bayesian methods are employed for risk assessment, portfolio optimization, and economic forecasting. The ability to update models as new data becomes available makes Bayesian statistics particularly valuable in the fast-paced financial sector.
Machine Learning and Artificial Intelligence: Bayesian approaches are integral to machine learning, particularly in predictive modeling and decision-making processes. Bayesian inference supports the development of more robust and adaptable AI systems, with applications ranging from natural language processing to robotics.
Challenges and Criticisms
Despite its advantages, Bayesian statistics faces several challenges:
Computational Complexity: Bayesian methods can be computationally intensive, especially for large-scale models. However, advancements in algorithms and computing power continue to mitigate these challenges, making Bayesian methods more feasible for a broader range of applications.
Choice of Priors and Subjectivity: The selection of prior distributions can introduce subjectivity into the analysis, which is a common criticism of Bayesian methods. Transparent and well-justified prior choices are essential to maintaining the objectivity and credibility of Bayesian analysis.
Interpretation and Communication: Communicating Bayesian results can be challenging, particularly to audiences more familiar with frequentist methods. Educating stakeholders on the differences in interpretation and the advantages of Bayesian approaches is crucial for broader acceptance and understanding.
Bayesian Statistics vs. Frequentist Methods
Comparative Strengths and Weaknesses
Bayesian statistics offers several strengths, including flexibility, incorporation of prior knowledge, and robust handling of uncertainty. However, frequentist methods also have their advantages, such as standardization, objectivity, and widespread acceptance in many scientific and regulatory contexts. Each approach has its own strengths and is suited to different types of problems.
Choosing the Appropriate Method
The choice between Bayesian and frequentist methods often depends on the context and specific requirements of the analysis. In some cases, integrating both approaches can provide a more comprehensive understanding of the data. Best practices involve carefully considering the nature of the data, the objectives of the analysis, and the availability of prior knowledge when selecting the appropriate method.
Future Directions and Developments
Advances in Bayesian Methodologies
Bayesian statistics is an area of active research, with ongoing developments in sampling methods, algorithms, and theoretical foundations. These advancements are expanding the scope of Bayesian analysis, making it more applicable to a wider range of complex and interdisciplinary problems.
Expansion of Applications
As Bayesian methods become more accessible and better understood, their application is expected to expand across diverse fields, from environmental science to economics. The growing recognition of the benefits of Bayesian approaches is driving broader adoption and interdisciplinary collaborations.
Educational and Training Resources
To support the continued growth of Bayesian statistics, there is an increasing emphasis on improving education and training in this area. Resources such as online courses, workshops, and textbooks are helping to build expertise in Bayesian methods, ensuring that more researchers and practitioners can effectively apply these powerful tools.
Why Bayesian Statistics Outperforms Frequentist Methods
Bayesian statistics stands out due to its unique advantages in incorporating prior knowledge, updating models with new data, and managing uncertainty. This flexibility allows for a more nuanced analysis, especially in complex scenarios and small sample sizes. Unlike frequentist methods, Bayesian approaches provide a probabilistic interpretation of results, which aids in clearer decision-making and more accurate predictions.
As we advance, Bayesian methods are increasingly relevant due to improvements in computational techniques and their growing application across various fields. Understanding why Bayesian statistics is better than frequentist methods highlights its ability to offer robust, adaptable, and contextually informed insights, making it a powerful tool for contemporary statistical analysis.
Summary of Bayesian Statistics Advantages
Bayesian statistics offers distinct advantages over frequentist methods, particularly in incorporating prior knowledge, handling complex models, and managing uncertainty. These benefits make Bayesian approaches particularly valuable in dynamic and complex real-world applications.
Future Outlook and Continued Relevance
With ongoing advancements and increasing applications, Bayesian statistics is poised to remain a crucial tool in the statistical toolkit. As computational methods continue to improve, the accessibility and relevance of Bayesian methods will only grow.
Final Thoughts and Recommendations
For practitioners and researchers, a strong understanding of both Bayesian and frequentist methods is essential. By choosing the appropriate approach based on the context and integrating the strengths of each method, one can achieve more robust and insightful statistical analyses.
Excited by What You've Read?
There's more where that came from! Sign up now to receive personalized financial insights tailored to your interests.
Stay ahead of the curve - effortlessly.