When Forecasting Using A Linear Trend Model One Should Be Wary Of Too Far Into The Future
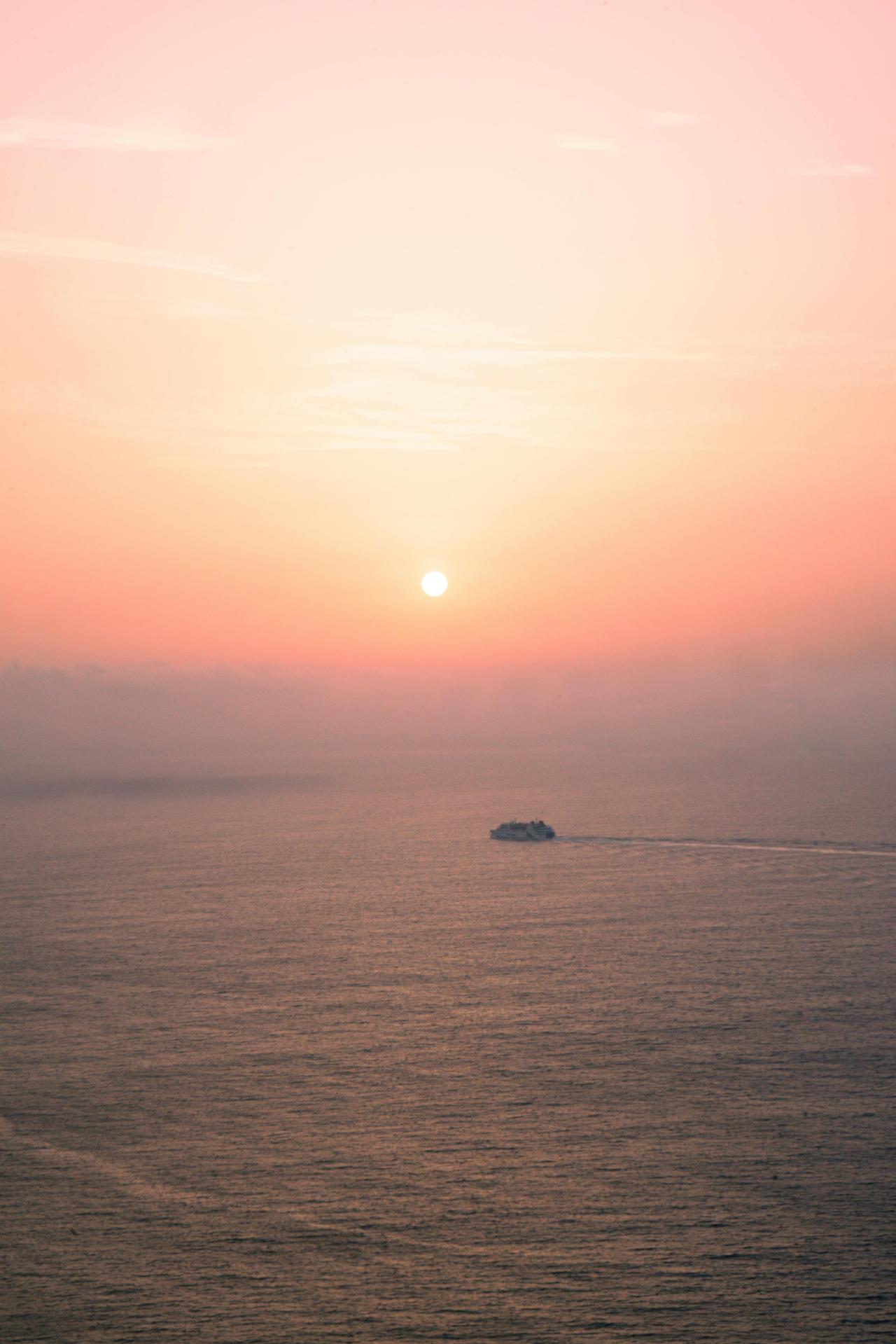
Forecasting using a linear trend model involves extrapolating historical data into the future based on a linear relationship. However, caution is needed when projecting too far into the future, as the model’s simplicity may not account for potential changes in underlying patterns or unforeseen variables. Linear models assume that trends will continue in a straight line, but real-world data can exhibit non-linear behavior or structural changes over time. This can lead to increasingly inaccurate forecasts if the projection horizon extends too far beyond the range of the historical data.
Limitations of Linear Trend Models
Issue | Description |
---|---|
Assumption of Linearity | Assumes future trends will continue in a straight line. |
Ignoring Non-linear Patterns | May not account for changes in trend direction or variability. |
Impact of External Factors | Unpredictable events or changes may impact future values. |
“Be cautious of relying too heavily on linear trends for long-term forecasts, as they may not capture the complexity of future data.”
Mathematical Representation
The general form of a linear trend model is:
\[ \text{Forecast} = \alpha + \beta t \]where \( \alpha \) is the intercept, \( \beta \) is the slope, and \( t \) is the time period.
This model is simple but may become less accurate as \( t \) increases significantly beyond the data range.
Understanding Linear Trend Models
Definition and Basics
What Is a Linear Trend Model? A linear trend model is a statistical tool used in forecasting to predict future values based on past data. It operates on the principle that data follows a linear pattern over time, represented by the equation \( y = mx + b \), where \( m \) is the slope, \( b \) is the intercept, and \( y \) is the predicted value.
How Linear Trend Models Work Linear trend models fit a straight line to historical data points. The method involves calculating the best-fit line that minimizes the distance between the actual data points and the predicted line, often using the least squares method.
Applications of Linear Trend Models These models are widely used in various fields, including business, economics, and environmental science, to predict future trends based on historical data. For instance, businesses use them for sales forecasting, while economists might use them to predict economic indicators.
Limitations of Linear Trend Models
Short-Term vs. Long-Term Forecasting
Accuracy in the Short Term Linear trend models tend to be more accurate in the short term because they assume that historical patterns will continue unchanged. Factors like stable market conditions and consistent consumer behavior contribute to their reliability over shorter periods.
Challenges with Long-Term Predictions Over longer periods, the assumptions of a linear trend model may not hold due to changing trends and external factors. Predicting far into the future can lead to significant inaccuracies, as the linear model does not account for non-linear changes or unexpected disruptions.
Examples of Misleading Predictions Historical cases, such as overly optimistic sales forecasts during economic booms or underestimating market crashes, highlight the risks of relying solely on linear trends for long-term predictions. These examples emphasize the need for caution and additional methods.
External Influences and Variability
Economic and Market Changes Shifts in the economy, such as recessions or booms, can drastically alter trends. A linear model might fail to predict these shifts accurately, leading to substantial forecasting errors.
Technological and Social Changes Technological advancements and social changes can significantly impact trends. For instance, the rapid adoption of smartphones or social media trends can render previous linear forecasts obsolete.
Seasonal and Cyclical Patterns Linear models often overlook seasonal and cyclical variations. Incorporating methods to adjust for these patterns is crucial for improving long-term forecast accuracy.
Best Practices for Using Linear Trend Models
Data Quality and Selection
Importance of Accurate Data The accuracy of a linear trend model heavily depends on the quality of the input data. Ensuring data is clean, accurate, and relevant is essential for reliable forecasts.
Choosing Relevant Data Points Selecting appropriate data points that represent the trend accurately is critical. Excluding outliers and ensuring data consistency helps in creating a more reliable model.
Updating Data Regularly Regularly updating the model with new data ensures it reflects the most current trends and conditions, improving its accuracy over time.
Incorporating Additional Factors
Combining Models Using linear trend models alongside other forecasting methods, such as time series analysis or machine learning, can provide a more comprehensive view and enhance accuracy.
Adjusting for External Variables Incorporating external variables like economic indicators, technological trends, and social factors can adjust the model to reflect real-world complexities.
Scenario Analysis Scenario analysis involves exploring different future possibilities by varying key assumptions and conditions. This approach can help in understanding potential outcomes and preparing for uncertainties.
Tools and Techniques for Improved Forecasting
Advanced Forecasting Models
Polynomial and Non-Linear Models Polynomial and non-linear models can capture more complex trends that linear models might miss. These are particularly useful when the data shows curvature rather than a straight line.
Time Series Analysis Time series models, such as ARIMA, consider the temporal order of data points and can capture patterns like seasonality and trends more effectively than linear models.
Machine Learning Approaches Machine learning techniques, including neural networks and ensemble models, can analyze vast amounts of data and identify complex patterns, often outperforming traditional linear models in accuracy.
Software and Tools
Forecasting Software There are numerous forecasting tools available, such as Excel, R, Python, and specialized software like SAS and SPSS, which offer robust functionalities for building and analyzing forecasting models.
Data Visualization Tools Effective data visualization helps in interpreting forecast results and communicating findings. Tools like Tableau, Power BI, and Google Data Studio are invaluable for creating clear and informative visualizations.
Integration with Other Systems Integrating forecasting tools with business systems ensures seamless data flow and facilitates real-time forecasting and decision-making.
Evaluating Forecasting Accuracy
Monitoring and Adjusting Forecasts
Regular Review of Predictions Continuously reviewing and updating forecasts with new data helps in maintaining accuracy and relevance.
Error Analysis Analyzing forecasting errors helps in identifying weaknesses in the model and areas for improvement. Metrics like Mean Absolute Error (MAE) and Root Mean Squared Error (RMSE) are commonly used for this purpose.
Feedback Loops Implementing feedback mechanisms allows for continuous improvement of forecasting models by incorporating learnings from past errors and adjusting accordingly.
Communicating Forecasts
Presenting Forecast Results Clear and concise presentation of forecast results is crucial for stakeholder understanding and decision-making. Visual aids and executive summaries can enhance clarity.
Explaining Uncertainties Communicating the uncertainties and limitations of forecasts helps manage expectations and allows for more informed decision-making.
Documenting Forecasting Processes Thorough documentation of the forecasting process ensures transparency and provides a reference for future improvements.
Navigating the Future with Linear Trend Models
Understanding Limitations and Best Practices Linear trend models offer a straightforward approach to forecasting but come with significant limitations, particularly when predicting far into the future. While they are useful for short-term predictions where trends are stable, their accuracy diminishes over extended periods due to the inherent assumption that historical patterns will continue unchanged. This can be problematic as it fails to account for potential economic shifts, technological advancements, and other external influences.
Incorporating Advanced Techniques To enhance the reliability of forecasts, it is crucial to supplement linear trend models with additional methods and data. Techniques such as time series analysis, machine learning, and scenario planning can provide a more nuanced understanding of future trends. These methods can better accommodate non-linear patterns, seasonal variations, and sudden market changes, thereby improving forecast accuracy.
Maintaining Data Quality and Continuous Monitoring Ensuring the accuracy and relevance of input data is foundational for effective forecasting. Regular updates and continuous monitoring of the model against actual outcomes are necessary to maintain its validity. Employing robust data visualization tools and error analysis techniques, such as Mean Absolute Error (MAE) and Root Mean Squared Error (RMSE), helps in identifying discrepancies and refining the model.
Communicating Forecast Results Effectively Clear communication of forecast results, including their uncertainties and limitations, is vital for informed decision-making. Visual aids and comprehensive documentation enhance stakeholder understanding and ensure transparency in the forecasting process.
Future Outlook While linear trend models remain a valuable tool in the forecaster’s toolkit, relying solely on them for long-term predictions is risky. A balanced approach that integrates various forecasting methods and continuously evolves with new data and technologies will lead to more robust and reliable predictions, helping organizations navigate future uncertainties more effectively.
Excited by What You've Read?
There's more where that came from! Sign up now to receive personalized financial insights tailored to your interests.
Stay ahead of the curve - effortlessly.