What Is Covariance And Why Is It Important In Portfolio Theory
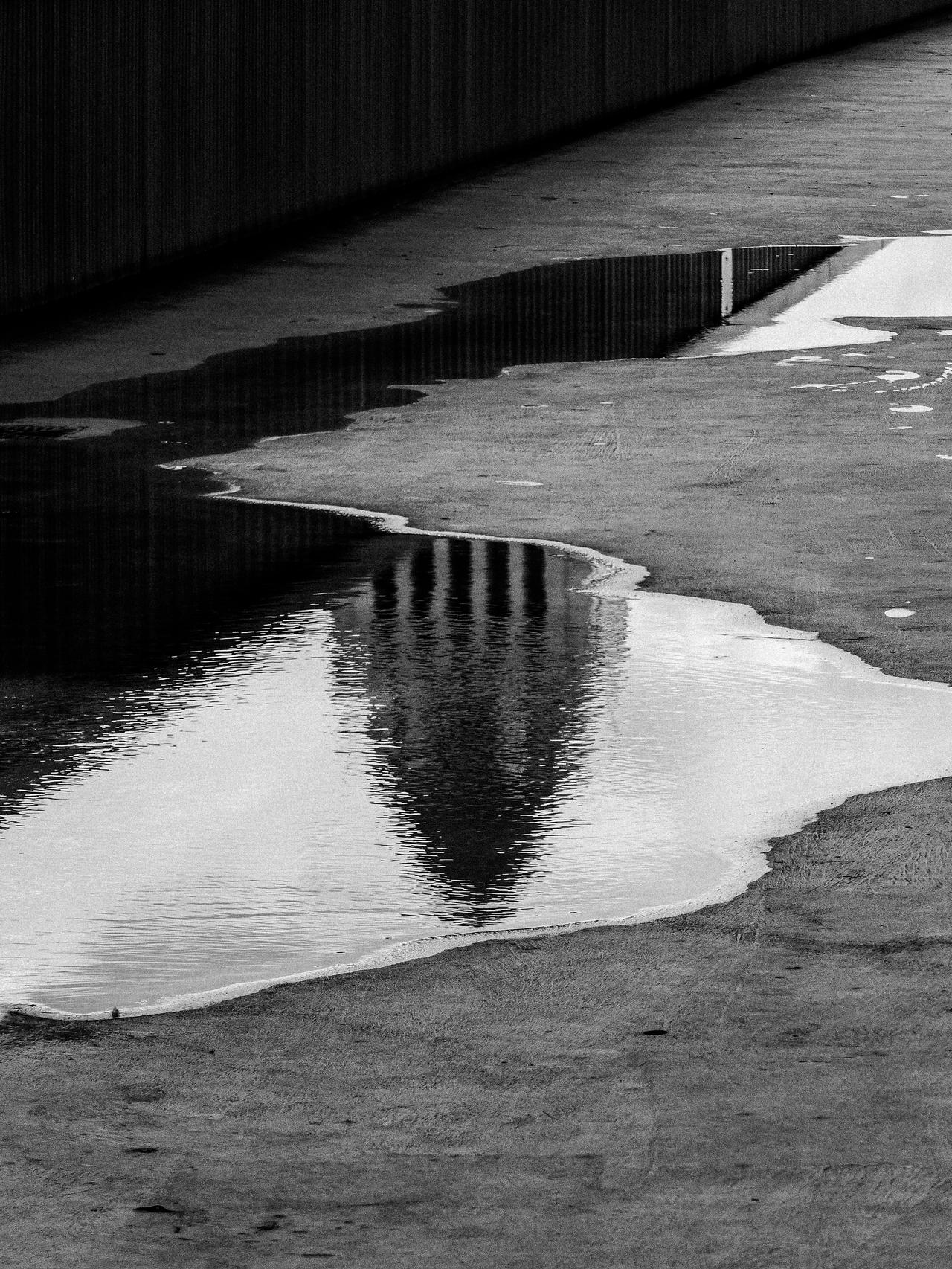
Portfolio Theory is a cornerstone of modern financial management that focuses on optimizing investment portfolios to achieve the best possible returns for a given level of risk. A critical component of this theory is understanding how different assets interact with each other, which is where the concept of covariance comes into play. Covariance measures how two asset returns move in relation to each other. Specifically, it indicates whether the returns on two assets tend to rise and fall together or move in opposite directions.
What is Covariance and Why is It Important in Portfolio Theory? Covariance helps investors understand the degree to which the returns of two assets are correlated. This information is vital for constructing a diversified portfolio that minimizes risk. By combining assets with different covariances, investors can reduce the overall risk of the portfolio, as some assets may offset the risks of others. Thus, covariance is essential for effective risk management and portfolio optimization.
Covariance and Diversification
In portfolio theory, diversification is a key strategy for reducing risk without sacrificing returns. Covariance plays a central role in diversification by enabling investors to select assets that do not move in perfect correlation with one another. When assets with low or negative covariance are combined in a portfolio, the overall risk is reduced because the likelihood of simultaneous negative returns across the portfolio decreases. This is the foundation of modern portfolio theory, which suggests that the risk of a portfolio is not merely the sum of its individual risks but is also influenced by the covariance between the assets.
Covariance Calculation and Portfolio Impact
Covariance between two assets is calculated using the formula:
\[ \text{Cov}(X, Y) = \frac{1}{n-1} \sum_{i=1}^{n} (X_i - \bar{X})(Y_i - \bar{Y}) \]Where:
- \( X_i \) and \( Y_i \) are the individual returns of the assets \( X \) and \( Y \) at time \( i \)
- \( \bar{X} \) and \( \bar{Y} \) are the mean returns of assets \( X \) and \( Y \)
- \( n \) is the number of observations
This calculation helps in understanding how the assets in a portfolio interact with one another, which is essential for minimizing risk through diversification.
Portfolio Variance and Covariance Matrix
The importance of covariance is further emphasized when calculating the variance of a portfolio, which depends on the covariance between all pairs of assets. The variance of a portfolio composed of multiple assets can be expressed as:
\[ \text{Portfolio Variance} = \sum_{i=1}^{n} \sum_{j=1}^{n} w_i w_j \text{Cov}(X_i, X_j) \]Where:
- \( w_i \) and \( w_j \) are the weights of the assets in the portfolio
- \( \text{Cov}(X_i, X_j) \) represents the covariance between assets \( X_i \) and \( X_j \)
This equation highlights that the total risk of a portfolio is a function not just of the variances of the individual assets but also of their covariances.
Importance of Covariance in Risk Management
Covariance Value | Interpretation | Impact on Portfolio |
---|---|---|
Positive Covariance | Assets move in the same direction | Higher risk if both assets decline |
Negative Covariance | Assets move in opposite directions | Potential risk reduction |
Zero Covariance | No consistent relationship between assets | Neutral effect on risk |
Portfolio Risk Reduction Strategy
“Covariance is a critical factor in portfolio theory because it allows investors to understand the interrelationships between assets, enabling the construction of a diversified portfolio that minimizes risk while maintaining potential returns.”
This perspective underscores the role of covariance in achieving effective portfolio diversification, highlighting its importance in the broader context of portfolio theory and risk management.
Understanding Covariance in Portfolio Theory
Defining Covariance
Concept of Covariance
Covariance is a statistical measure that assesses the degree to which two variables move in relation to each other. In finance, it is used to determine how the returns of two assets move together.
- Mathematical Definition: Covariance is calculated as the average of the product of the deviations of two variables from their respective means. Mathematically, it is represented as:
where \(X_i\) and \(Y_i\) are the individual returns of assets X and Y, and \(\mu_X\) and \(\mu_Y\) are the mean returns of assets X and Y, respectively.
Calculation Method: To calculate covariance, you first determine the mean returns of the assets, then find the deviation of each return from its mean, multiply the deviations of the two assets, and finally take the average of these products.
Relationship with Variance: Variance is a special case of covariance where the two variables are the same. It measures the dispersion of a single asset’s returns, while covariance measures the joint variability of two assets.
Covariance in Statistics
Covariance plays a critical role in statistical analysis, especially in understanding the relationship between variables.
Role in Statistical Analysis: Covariance helps to understand whether an increase in one variable corresponds to an increase or decrease in another. It is a foundational concept in multivariate statistics.
Comparison with Correlation: While covariance indicates the direction of the relationship between two variables, correlation standardizes this measure, providing a dimensionless value between -1 and 1, which shows both the strength and direction of the relationship.
Applications in Data Analysis: Covariance is widely used in various fields to analyze relationships between data sets, particularly in finance for assessing the co-movement of asset returns.
Types of Covariance
Positive vs. Negative Covariance: Positive covariance indicates that the returns of two assets move in the same direction, while negative covariance indicates that they move in opposite directions.
Zero Covariance: If the covariance is zero, it suggests that the returns of the two assets are independent of each other.
Implications for Investment Analysis: Understanding the type of covariance between assets is crucial for portfolio diversification and risk management.
Importance of Covariance in Portfolio Theory
Portfolio Diversification
Covariance is essential in portfolio theory because it informs how combining different assets can reduce risk.
Role in Risk Reduction: By combining assets with low or negative covariance, investors can reduce the overall risk of the portfolio, as the poor performance of one asset may be offset by the better performance of another.
Impact on Portfolio Volatility: The overall volatility of a portfolio is influenced by the covariances between its constituent assets. Lower covariance between assets generally results in lower portfolio volatility.
Optimizing Asset Allocation: Covariance is a key factor in determining the optimal mix of assets that minimizes risk for a given level of expected return.
Covariance and Correlation
Understanding the relationship between covariance and correlation is crucial for portfolio construction.
Comparison with Correlation Coefficient: While covariance gives the direction of the relationship, correlation provides both direction and magnitude, making it a more interpretable measure for assessing how closely two assets move together.
Interrelationship with Asset Returns: The correlation coefficient is derived from covariance and provides a clearer view of how asset returns relate, which is critical in constructing a diversified portfolio.
Influence on Portfolio Construction: By using correlation (which is derived from covariance), investors can select assets that complement each other, reducing overall portfolio risk.
Mathematical Foundation
Covariance is mathematically integral to portfolio models, particularly in modern portfolio theory.
Formula for Covariance: The covariance between two assets is calculated using the formula mentioned earlier. It is a core input in the calculation of portfolio variance.
Integration into Portfolio Models: Covariance is used to construct the covariance matrix, which is essential in optimizing portfolios using models like the Markowitz model.
Application in Modern Portfolio Theory: In Harry Markowitz’s Modern Portfolio Theory (MPT), covariance is used to calculate the efficient frontier, which represents the set of optimal portfolios that offer the highest expected return for a given level of risk.
Application of Covariance in Portfolio Management
Portfolio Construction
Impact on Asset Selection
Covariance guides investors in selecting assets that work well together to create a balanced portfolio.
Choosing Assets Based on Covariance: Assets with low or negative covariance are ideal for diversification, as they can help reduce the overall risk of the portfolio.
Balancing Risk and Return: Covariance helps in creating portfolios that strike a balance between risk and return, ensuring that investors achieve their financial goals with an acceptable level of risk.
Creating Efficient Frontiers: By using covariance to optimize the asset mix, investors can identify portfolios on the efficient frontier, which offer the best possible return for a given level of risk.
Risk Management
Using Covariance to Predict Risk: Covariance allows investors to estimate how different asset combinations will behave in various market conditions, helping to predict potential risks.
Evaluating Risk of Asset Combinations: By analyzing the covariance between assets, investors can assess how the inclusion of a new asset will affect the overall risk profile of the portfolio.
Adjusting Portfolio for Desired Risk Levels: Covariance analysis helps in adjusting the portfolio to meet the investor’s risk tolerance by selecting assets that complement each other.
Performance Evaluation
Assessing Portfolio Performance Metrics: Covariance is integral in evaluating performance metrics such as the Sharpe ratio, which measures risk-adjusted return.
Impact on Sharpe Ratio: A portfolio constructed using covariance analysis is likely to have a better Sharpe ratio, as the risks are well-managed through diversification.
Analyzing Portfolio Efficiency: Covariance helps in determining whether a portfolio is operating efficiently or if adjustments are needed to improve performance.
Practical Examples
Real-World Asset Combinations
Understanding how covariance applies to real-world asset combinations can provide practical insights into portfolio management.
Case Studies of Diverse Portfolios: Historical case studies demonstrate how portfolios with assets exhibiting low or negative covariance have outperformed less diversified portfolios in terms of risk-adjusted returns.
Historical Data Analysis: Analyzing historical data helps investors understand how covariance has affected portfolio performance over time, guiding future investment decisions.
Simulations and Scenarios: Using simulations to analyze different covariance scenarios allows investors to prepare for various market conditions and optimize their portfolios accordingly.
Covariance in Different Markets
Stock Markets: In stock markets, covariance is used to assess how different stocks move in relation to each other, guiding diversification strategies.
Bond Markets: Covariance is also crucial in bond markets, helping investors combine bonds with varying risk profiles to create a stable income stream.
Alternative Investments: For alternative investments, covariance analysis is used to integrate these assets into traditional portfolios, enhancing diversification and potentially improving returns.
Portfolio Optimization Techniques
Markowitz Model: The Markowitz model relies heavily on covariance to create the efficient frontier, guiding investors in constructing optimal portfolios.
Black-Litterman Model: This model incorporates covariance and investor views to create more tailored portfolio strategies, addressing some of the limitations of the Markowitz model.
Factor Models: Factor models use covariance to assess how different factors (such as market, size, and value factors) affect asset returns, allowing for more nuanced portfolio construction.
Theoretical Underpinnings and Models
Modern Portfolio Theory (MPT)
Foundations of MPT
Harry Markowitz’s Contributions: Markowitz’s introduction of MPT revolutionized portfolio management by highlighting the importance of diversification and covariance in minimizing risk.
Efficient Frontier Concept: The efficient frontier is a key concept in MPT, representing the set of portfolios that offer the highest return for a given level of risk, which is calculated using covariance.
Risk-Return Trade-Off: MPT emphasizes the trade-off between risk and return, with covariance playing a central role in determining the optimal balance.
Integration of Covariance in MPT
Covariance Matrix Construction: The covariance matrix is a fundamental component of MPT, used to calculate the variance of portfolio returns and identify optimal asset combinations.
Impact on Optimal Portfolio Weights: Covariance influences the weights assigned to different assets in the portfolio, guiding the construction of a diversified portfolio that minimizes risk.
Utilization in Asset Allocation: Covariance is used in asset allocation models to determine the best mix of assets that aligns with the investor’s risk tolerance and financial goals.
Advancements in Portfolio Theory
Evolution of MPT: Since its introduction, MPT has evolved to incorporate new models and techniques, many of which still rely on covariance as a key input.
Incorporation of Covariance in New Models: New portfolio models, such as the Black-Litterman model, build on MPT by integrating covariance with additional factors, offering more flexible and tailored investment strategies.
Comparative Analysis with Alternative Theories: While alternative theories have emerged, such as behavioral finance, covariance remains a critical component in most quantitative approaches to portfolio management.
Advanced Portfolio Models
Factor Models
Understanding Factor Exposures: Factor models use covariance to assess how different macroeconomic factors impact asset returns, helping investors manage risk more effectively.
Role of Covariance in Factor Models: Covariance is used
to calculate the sensitivity of assets to different factors, guiding the construction of factor-based portfolios.
- Applications in Risk Management: Factor models are widely used in risk management, with covariance playing a central role in identifying and mitigating risk exposures.
Black-Litterman Model
Incorporation of Investor Views: The Black-Litterman model combines investor views with covariance data to create more tailored and robust portfolio strategies.
Covariance in Model Calibration: Covariance is used to calibrate the Black-Litterman model, ensuring that the portfolio reflects both market data and investor insights.
Enhanced Portfolio Optimization: By integrating covariance with investor views, the Black-Litterman model offers a more flexible approach to portfolio optimization, addressing some of the limitations of traditional MPT.
Dynamic Portfolio Management
Adjusting for Market Changes: Dynamic portfolio management uses time-varying covariance to adjust portfolios in response to changing market conditions.
Time-Varying Covariance: This approach recognizes that covariance is not static and can change over time, requiring continuous monitoring and adjustment.
Adaptation Strategies: Investors can use time-varying covariance to develop adaptive strategies that respond to market shifts, enhancing portfolio resilience.
Practical Considerations in Portfolio Management
Data and Computation
Covariance Matrix Estimation
Data Requirements: Estimating the covariance matrix requires accurate and comprehensive data on asset returns, making data quality a critical factor in portfolio analysis.
Estimation Techniques: Various techniques, such as historical estimation, shrinkage methods, and factor models, are used to estimate the covariance matrix.
Challenges in Computation: Estimating the covariance matrix can be computationally intensive, particularly for large portfolios, and requires sophisticated tools and software.
Software and Tools
Financial Modeling Software: Tools like MATLAB, R, and Python are widely used for covariance analysis and portfolio optimization.
Covariance Analysis Tools: Specialized software, such as portfolio management platforms, offer built-in tools for covariance analysis, making it easier for investors to manage complex portfolios.
Visualization and Interpretation: Advanced visualization tools help investors interpret covariance data and make informed decisions based on the analysis.
Limitations and Challenges
Data Accuracy: The accuracy of the covariance matrix depends on the quality of the input data, making it crucial to use reliable and up-to-date information.
Model Assumptions: Covariance analysis relies on several assumptions, such as the linear relationship between asset returns, which may not always hold true in the real world.
Potential Pitfalls in Analysis: Over-reliance on historical data and the assumption of constant covariance can lead to suboptimal investment decisions, highlighting the need for robust risk management practices.
Strategic Applications
Tailoring Portfolios to Investor Goals
Risk Tolerance Adjustments: Covariance analysis allows investors to adjust their portfolios based on their risk tolerance, ensuring that the portfolio aligns with their financial objectives.
Long-Term vs. Short-Term Goals: By understanding the covariance between assets, investors can design portfolios that meet both long-term growth and short-term liquidity needs.
Customizing Asset Mix: Covariance analysis enables investors to customize their asset mix, balancing risk and return to achieve their financial goals.
Behavioral Considerations
Investor Behavior and Covariance: Behavioral factors, such as loss aversion, can influence how investors perceive and respond to covariance, affecting their portfolio choices.
Psychological Factors in Portfolio Choices: Understanding the psychological impact of risk and uncertainty can help investors make more informed decisions, avoiding common biases.
Mitigating Behavioral Biases: By relying on data-driven analysis and objective metrics like covariance, investors can mitigate the impact of behavioral biases on their investment decisions.
Regulatory and Compliance Issues
Adhering to Investment Guidelines: Covariance analysis helps investors adhere to regulatory guidelines by ensuring that portfolios are diversified and aligned with risk management standards.
Disclosure Requirements: Investors may be required to disclose how covariance and other risk metrics are used in portfolio management, ensuring transparency and accountability.
Ethical Considerations: Ethical considerations, such as ensuring fair treatment of investors and avoiding conflicts of interest, are important in portfolio management, particularly when using advanced techniques like covariance analysis.
Unlocking the Value of Covariance in Portfolio Theory
Essential Role in Investment Strategies
Guiding Asset Allocation: Understanding covariance is crucial for optimizing asset allocation. It helps investors to strategically combine assets to minimize risk and enhance returns by revealing how asset returns move together.
Risk Mitigation Insights: Covariance is key to effective risk management. By analyzing how assets interact, investors can construct portfolios that are better equipped to handle market volatility and achieve stable performance.
Foundational Theory and Practical Application: The concept of covariance is deeply embedded in portfolio theory and practical investment strategies. It provides essential insights for creating diversified portfolios and enhancing overall investment outcomes.
Future Directions and Innovations
Evolving Methodologies: Ongoing research and advancements in covariance analysis will likely lead to more sophisticated methods and models, improving how portfolios are constructed and managed.
Integration with New Technologies: Emerging tools and technologies will enhance covariance estimation and application, offering more precise and dynamic ways to optimize investment strategies.
Expanding Applications: Future developments may uncover new uses for covariance in various investment contexts, potentially transforming how investors approach portfolio management and risk assessment.
Understanding “what is covariance and why it is important in portfolio theory” not only clarifies its fundamental role but also underscores its impact on crafting resilient and efficient investment strategies.
Summary of Key Points
Recap of Covariance Importance
Role in Portfolio Theory: Covariance is a fundamental concept in portfolio theory, guiding asset selection, risk management, and portfolio optimization.
Impact on Investment Decisions: By understanding covariance, investors can make more informed decisions that balance risk and return, leading to more effective portfolio management.
Theoretical and Practical Insights: Covariance analysis provides both theoretical insights and practical tools for managing portfolios, making it an essential component of modern investment strategies.
Implications for Investors
Strategic Applications: Investors can use covariance to optimize their portfolios, reduce risk, and achieve their financial goals.
Risk Management Practices: Covariance analysis is critical for managing risk, helping investors create portfolios that are resilient to market fluctuations.
Future Trends in Portfolio Theory: As portfolio theory evolves, covariance will continue to play a central role in new models and techniques, guiding the development of more sophisticated investment strategies.
Future Research Directions
Emerging Topics in Covariance Analysis: Future research may explore new ways to estimate and use covariance, particularly in dynamic and uncertain environments.
Advancements in Portfolio Optimization: Continued innovation in portfolio optimization techniques will likely build on the foundation of covariance analysis, leading to more effective and adaptable strategies.
Potential Areas for Innovation: As technology and data analytics advance, new tools and methods for covariance analysis will emerge, offering investors more precise and powerful ways to manage their portfolios.
Excited by What You've Read?
There's more where that came from! Sign up now to receive personalized financial insights tailored to your interests.
Stay ahead of the curve - effortlessly.