Time Series Analysis List Out All The Values Hands-On Fresco Play
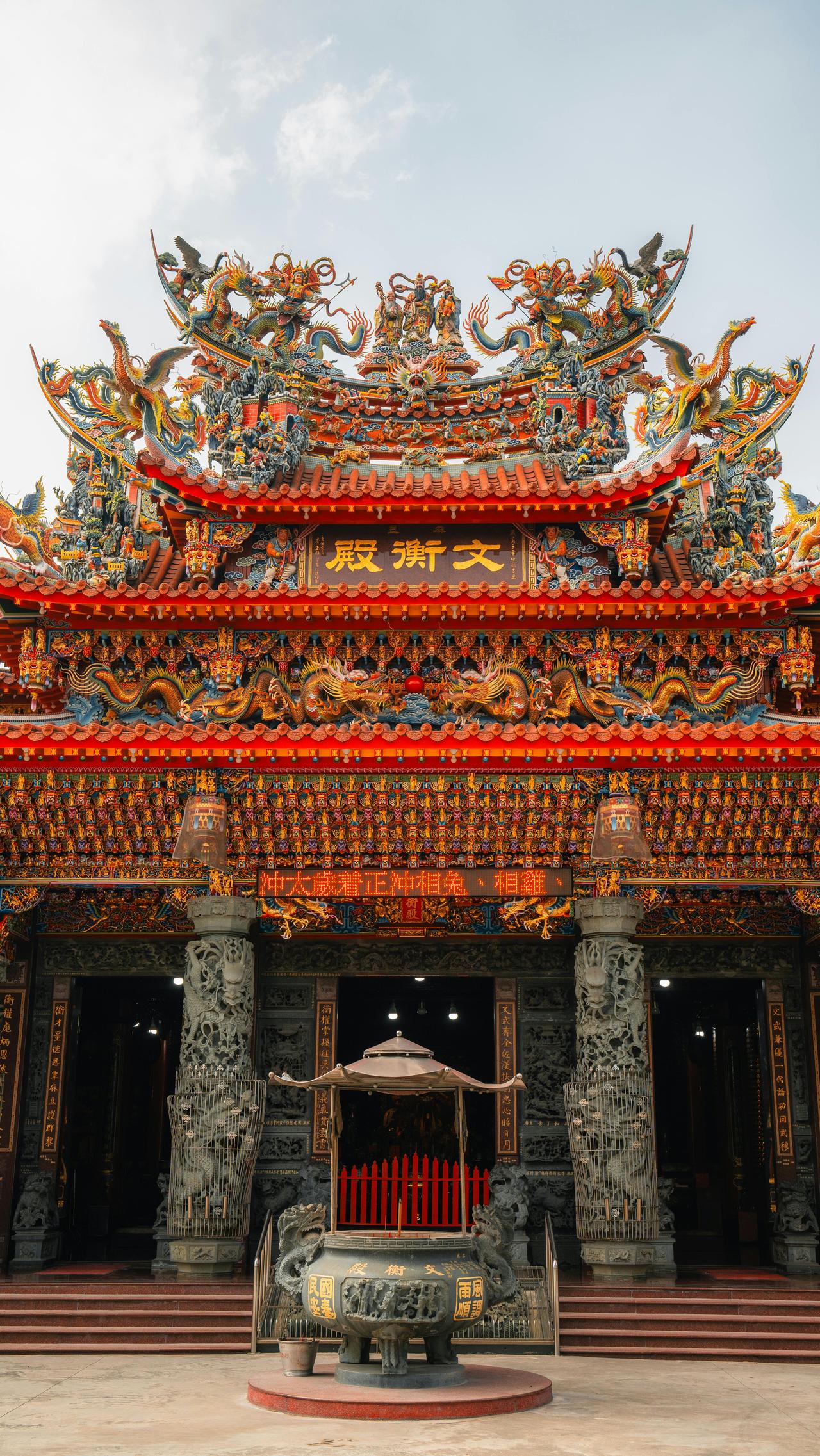
Time series analysis is a powerful statistical tool used to understand and predict patterns within data collected over time. It involves various methods and techniques to analyze temporal data, such as detecting trends, seasonality, and cyclical patterns. The search term “time series analysis list out all the values hands-on fresco play” points towards a practical application of time series methods in analyzing and interpreting data from a specific context, such as a game or application.
In the context of time series analysis, listing out all the values hands-on involves collecting and organizing data points sequentially to observe how they change over time. For instance, in a practical application like a game or a software application, this might involve recording various metrics or performance indicators at different time intervals. Analyzing these values can reveal underlying patterns and help in understanding how different variables influence the system over time.
By using time series analysis techniques, one can perform tasks such as plotting data points on a graph to visualize trends, calculating moving averages to smooth out fluctuations, and employing more advanced methods like autoregressive integrated moving average (ARIMA) models to forecast future values based on historical data. In the case of “hands-on fresco play,” this might involve listing out the scores or performance metrics over several games to analyze patterns or trends in player behavior and performance.
In summary, “time series analysis list out all the values hands-on fresco play” reflects the process of systematically collecting and analyzing sequential data points to gain insights into patterns and trends within a specific context, using practical, hands-on approaches to apply time series analysis methods effectively.
Time series analysis involves examining data points collected or recorded at specific time intervals to identify patterns, trends, and seasonal variations. This analysis helps in forecasting future values based on historical data, and it is widely used in fields such as finance, economics, and environmental studies. By analyzing time series data, one can gain insights into underlying processes and make informed predictions.
Time Series Analysis Techniques
Various techniques are employed in time series analysis to extract meaningful information from data:
- Trend Analysis: Identifies long-term movements in the data, such as upward or downward trends.
- Seasonal Decomposition: Separates data into seasonal components to understand periodic fluctuations.
- Autoregressive Models (AR): Models that predict future values based on past observations.
- Moving Averages: Smooths out short-term fluctuations to highlight longer-term trends.
Hands-On Fresco Play: Practical Application
“Hands-On Fresco Play” involves using practical tools and techniques for analyzing time series data. This approach emphasizes the application of theoretical concepts through real-world examples and exercises. By working directly with datasets, users can apply various time series methods to uncover insights and improve forecasting accuracy.
Comparison of Time Series Methods
Here is a table comparing some common time series analysis methods:
Method | Description | Advantages | Disadvantages |
---|---|---|---|
Trend Analysis | Identifies long-term trends in data | Highlights overall direction of data | May not account for seasonal variations |
Seasonal Decomposition | Breaks down data into seasonal, trend, and residual components | Useful for understanding periodic patterns | Can be complex to implement and interpret |
Autoregressive Models (AR) | Uses past values to predict future values | Captures relationships within the data | Assumes linearity and stationarity |
Moving Averages | Smooths data to identify trends | Reduces noise and short-term fluctuations | May lag behind actual trends |
Quote: “Effective time series analysis requires a combination of techniques to accurately model and forecast data trends, seasonality, and irregularities.”
Mathematical Foundations of Time Series Models
Understanding the mathematical basis of time series models is crucial for effective analysis. Consider the following formulas:
- Autoregressive Model (AR):
where \(X_t\) is the value at time \(t\), \(\phi_i\) are the parameters, and \(\epsilon_t\) is the error term.
- Moving Average (MA):
where \(MA_t\) is the moving average at time \(t\), and \(X_{t-i}\) represents past values.
By utilizing these methods and understanding their mathematical underpinnings, analysts can better interpret time series data and make more accurate forecasts.
Excited by What You've Read?
There's more where that came from! Sign up now to receive personalized financial insights tailored to your interests.
Stay ahead of the curve - effortlessly.