Time Series Analysis By State Space Methods By James Durbin And Siem Jan Koopman
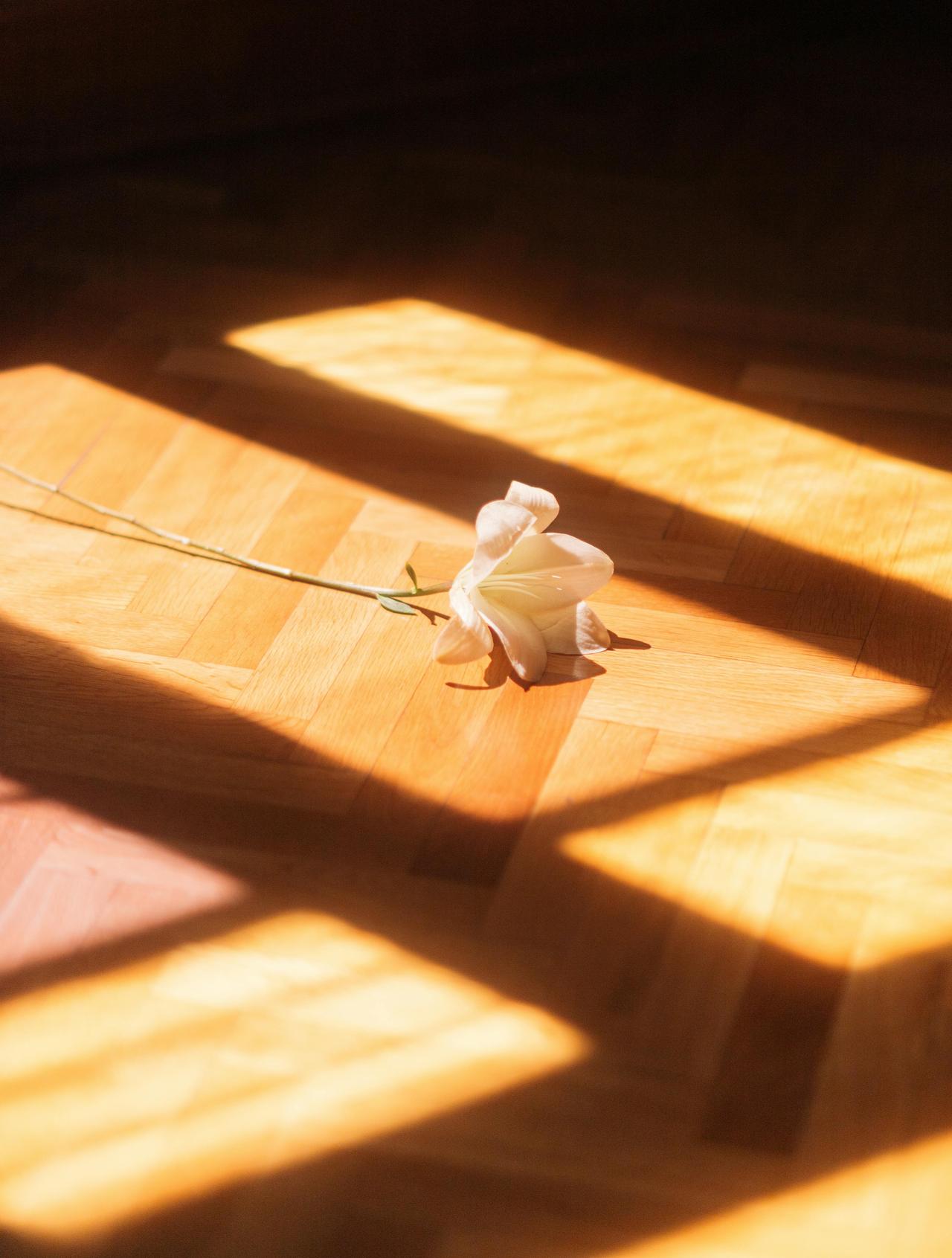
The concept of time series analysis is extensively explored through various methodologies, one of which is the state space approach. This technique is notably detailed in the work “Time Series Analysis by State Space Methods by James Durbin and Siem Jan Koopman.” The exact phrase “time series analysis by state space methods by James Durbin and Siem Jan Koopman” refers to this seminal text that offers a comprehensive examination of state space models, which are essential for analyzing and forecasting time series data.
State space models are a powerful class of models used in time series analysis that allow for the representation of dynamic systems through a set of linear equations. They provide a framework for handling data that evolves over time and is influenced by various internal and external factors. This approach is particularly useful for dealing with unobserved components in time series data, such as trends and seasonality, by modeling the system’s state variables and measurement errors.
In “Time Series Analysis by State Space Methods,” Durbin and Koopman offer an in-depth exploration of these models, detailing their theoretical underpinnings and practical applications. The book covers the formulation of state space models, estimation techniques, and various applications in fields such as economics and finance. It also discusses how to use state space models to manage and interpret time series data with missing values or irregular observations.
The state space methods introduced by Durbin and Koopman are instrumental in addressing the challenges posed by complex time series data. They enable analysts to build robust models that account for different types of variability and structural changes over time. By applying these methods, practitioners can enhance their ability to forecast future values, identify underlying patterns, and make informed decisions based on historical data. The work by Durbin and Koopman thus represents a key contribution to the field of time series analysis, offering valuable insights and tools for researchers and practitioners alike.
Time series analysis is a statistical technique used to analyze time-ordered data points, focusing on extracting meaningful patterns and forecasting future values. One powerful approach in time series analysis is the state space model, which provides a flexible framework for modeling and analyzing time-dependent processes. State space models consist of two main components: the state equation and the observation equation. The state equation models the evolution of the underlying state variables, while the observation equation relates these states to the observed data. This approach allows for the incorporation of various dynamic processes and can handle complex time series data.
State Space Models and Techniques
State Space Framework
The state space framework offers a comprehensive approach to time series modeling. It captures the underlying structure of the data through latent variables, which are not directly observed but inferred from the observed time series. This framework is particularly useful for dealing with irregular or missing data, as it can seamlessly integrate different sources of information. The state space model provides a structured way to analyze and predict time series data, making it a valuable tool in fields such as economics, finance, and engineering.
Filtering and Smoothing Methods
Filtering and smoothing are key techniques within the state space framework. Filtering refers to the process of estimating the current state of a system given past and present observations, while smoothing involves estimating past states given all available data. These techniques are essential for extracting insights from noisy or incomplete time series data. Kalman filtering, for example, is widely used for real-time state estimation in dynamic systems. Smoothing methods, such as the Rauch-Tung-Striebel smoother, provide refined estimates of past states by leveraging all available observations.
Application and Examples
Time Series Forecasting
Time series forecasting is a primary application of state space models. By analyzing historical data and identifying patterns, these models can generate predictions for future values. Techniques such as exponential smoothing and ARIMA (AutoRegressive Integrated Moving Average) models can be incorporated into the state space framework to enhance forecasting accuracy. Forecasting with state space models allows for the accommodation of seasonal effects, trends, and other dynamic elements, providing a robust tool for predicting future outcomes in various domains.
Real-World Case Studies
Real-world applications of state space models span various industries. In finance, these models are used to forecast stock prices and manage risk. In economics, they help analyze economic indicators and make policy decisions. In engineering, state space models are applied to control systems and signal processing. The versatility of state space models makes them suitable for a wide range of practical problems, demonstrating their importance in both theoretical and applied settings.
Advanced Topics in Time Series Analysis
Bayesian State Space Models
Bayesian state space models incorporate prior distributions and Bayesian inference into the state space framework. This approach allows for the incorporation of uncertainty and prior knowledge into the model estimation process. Bayesian methods provide a probabilistic view of the state space model, offering insights into parameter uncertainties and model robustness. This advanced topic enhances the flexibility and interpretability of state space models, making them more applicable to complex and uncertain environments.
Non-Linear State Space Models
Non-linear state space models extend the state space framework to handle non-linear relationships between the state variables and observations. These models are capable of capturing more complex dynamics compared to linear state space models. Techniques such as the extended Kalman filter and particle filter are used to estimate non-linear state space models. These advancements allow for more accurate modeling of systems with non-linear behavior, expanding the applicability of state space methods in time series analysis.
Key References and Further Reading
Essential Texts and Resources
“Time Series Analysis by State Space Methods” by James Durbin and Siem Jan Koopman: This seminal work provides a comprehensive introduction to state space models and their applications in time series analysis. It covers both theoretical foundations and practical implementation, making it a valuable resource for researchers and practitioners.
“State Space Models with Applications” by Paolo Giudici and Stefano G. Gallo: This book explores various applications of state space models, offering insights into real-world problems and advanced techniques in time series analysis.
Exploring these resources will deepen your understanding of state space methods and their applications, providing a solid foundation for advanced time series analysis.
Excited by What You've Read?
There's more where that came from! Sign up now to receive personalized financial insights tailored to your interests.
Stay ahead of the curve - effortlessly.