Stochastic Volatility Modeling Lorenzo Bergomi
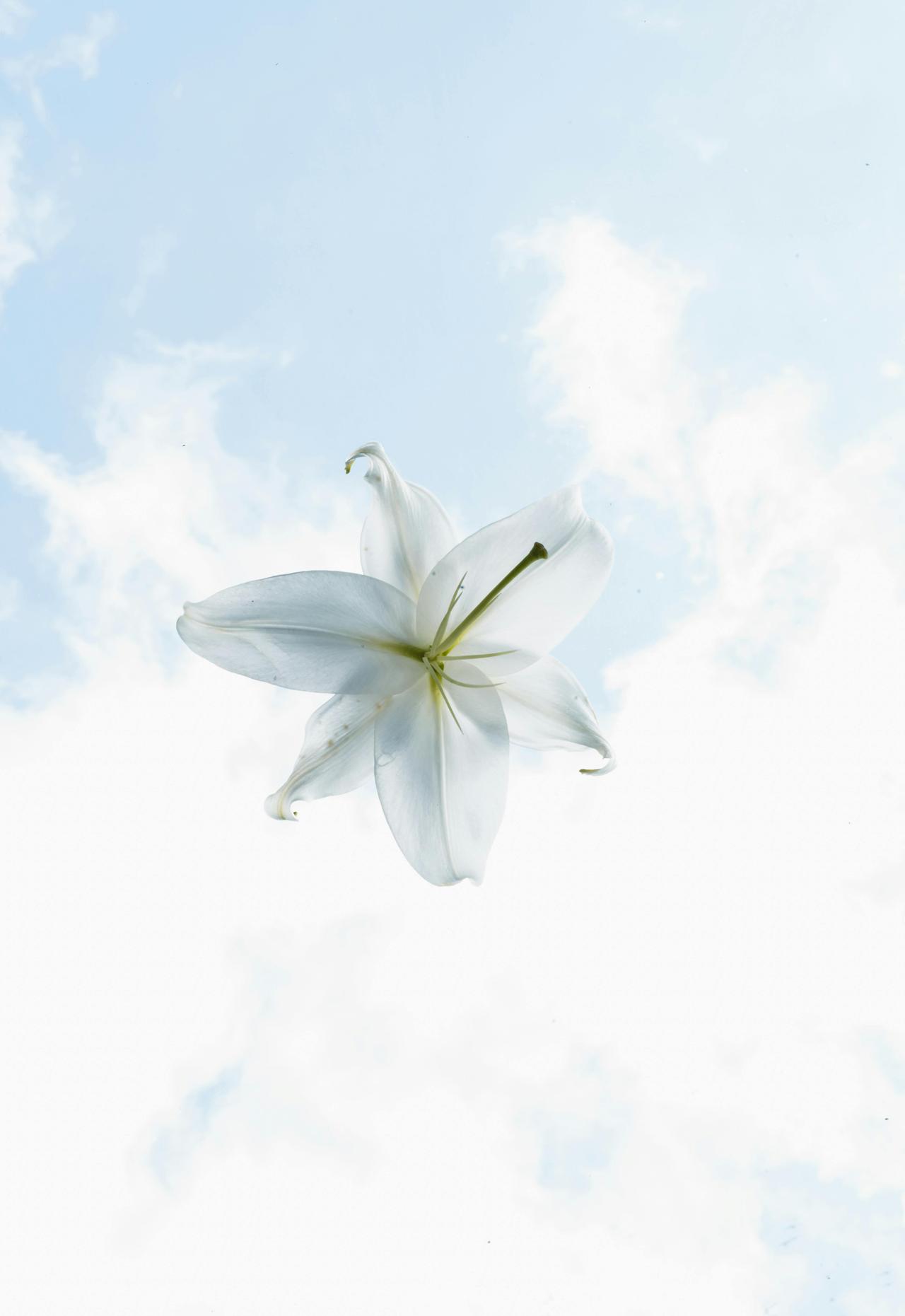
Stochastic volatility modeling is a crucial aspect of financial mathematics, focusing on capturing the dynamic nature of volatility in financial markets. One of the prominent contributors to this field is Lorenzo Bergomi, whose work on stochastic volatility modeling has significantly advanced our understanding of volatility dynamics. Stochastic volatility models, such as those proposed by Bergomi, are designed to address the limitations of simpler models by incorporating the fact that volatility is not constant but rather evolves over time in response to market conditions.
The term “stochastic volatility modeling Lorenzo Bergomi” refers to Bergomi’s approach to modeling volatility using stochastic processes. His work has provided a framework that accounts for the randomness in volatility and allows for a more realistic representation of financial markets. Bergomi’s models often involve complex mathematical techniques and statistical methods to capture the observed behavior of financial instruments. For instance, his contributions to the SABR (Stochastic Alpha Beta Rho) model have been particularly influential. The SABR model is used for pricing options and managing risks by incorporating stochastic volatility and correlating factors, which help in accurately pricing derivatives and understanding market movements.
Bergomi’s research also delves into the calibration of stochastic volatility models, which involves adjusting model parameters to fit real market data. This calibration process is essential for ensuring that the model accurately reflects market conditions and can be used for practical applications in trading and risk management. By improving the accuracy of volatility forecasts and options pricing, Bergomi’s work has provided valuable tools for both practitioners and researchers in the field of financial mathematics.
In summary, “stochastic volatility modeling Lorenzo Bergomi” encompasses Bergomi’s significant contributions to the development and application of models that account for the dynamic and stochastic nature of volatility. His work has played a pivotal role in enhancing our ability to model, analyze, and manage financial risk in increasingly complex markets.
Volatility modeling is crucial for financial markets, where it helps to estimate the variability of asset prices and manage risks effectively. Traditional models, such as the Black-Scholes model, assume constant volatility, which often does not hold true in real markets. To address this limitation, more advanced models have been developed to capture the dynamic nature of volatility. These models are essential for pricing derivatives, managing portfolios, and conducting risk assessments.
Stochastic Volatility Models
Understanding Stochastic Volatility
Introduction to Stochastic Volatility
Stochastic volatility models address the issue of changing volatility over time. Unlike static models, stochastic volatility models assume that volatility is driven by a random process. This approach provides a more realistic representation of market behavior, as it accounts for the fact that volatility can fluctuate in response to market conditions and economic events.
Benefits of Stochastic Volatility
- Realistic Market Behavior: Captures the dynamic nature of volatility better than constant volatility models.
- Improved Pricing Accuracy: Enhances the accuracy of derivative pricing by incorporating variable volatility.
- Risk Management: Provides better tools for managing risk by reflecting the uncertainty in volatility.
Key Stochastic Volatility Models
Heston Model Overview
The Heston model, developed by Steven Heston, is one of the most popular stochastic volatility models. It assumes that volatility follows a mean-reverting square-root process. The model is used to price options and other derivatives by accounting for the changing nature of volatility.
Model Formulation
In the Heston model, the volatility \( v(t) \) evolves according to:
\[ dv(t) = \kappa (\theta - v(t)) \, dt + \sigma_v \sqrt{v(t)} \, dW_v(t) \]where:
- \( \kappa \) is the rate of mean reversion,
- \( \theta \) is the long-term volatility variance,
- \( \sigma_v \) is the volatility of volatility,
- \( W_v(t) \) is a Brownian motion.
Advantages and Applications
- Accurate Derivative Pricing: Suitable for pricing options with varying volatility.
- Flexibility: Can be adapted to different market conditions and volatility structures.
Implementation and Analysis
Practical Considerations
- Parameter Estimation: Estimating the parameters of stochastic volatility models can be complex and requires historical data and sophisticated techniques.
- Computational Challenges: These models often involve complex calculations, which can be computationally intensive.
Example Use Case
In practice, stochastic volatility models are used to price equity options, interest rate derivatives, and other financial instruments. For example, traders and portfolio managers use the Heston model to assess the value of options and hedge against volatility risks.
Case Study: Financial Crisis
During periods of financial crisis, the ability of stochastic volatility models to adapt to sudden changes in market conditions becomes particularly valuable. These models can provide insights into extreme market events and help in developing strategies to mitigate potential losses.
By incorporating stochastic volatility into financial modeling, market participants gain a deeper understanding of price dynamics and improve their ability to make informed decisions in volatile environments.
Excited by What You've Read?
There's more where that came from! Sign up now to receive personalized financial insights tailored to your interests.
Stay ahead of the curve - effortlessly.