Stochastic Volatility Modeling By Lorenzo Bergomi
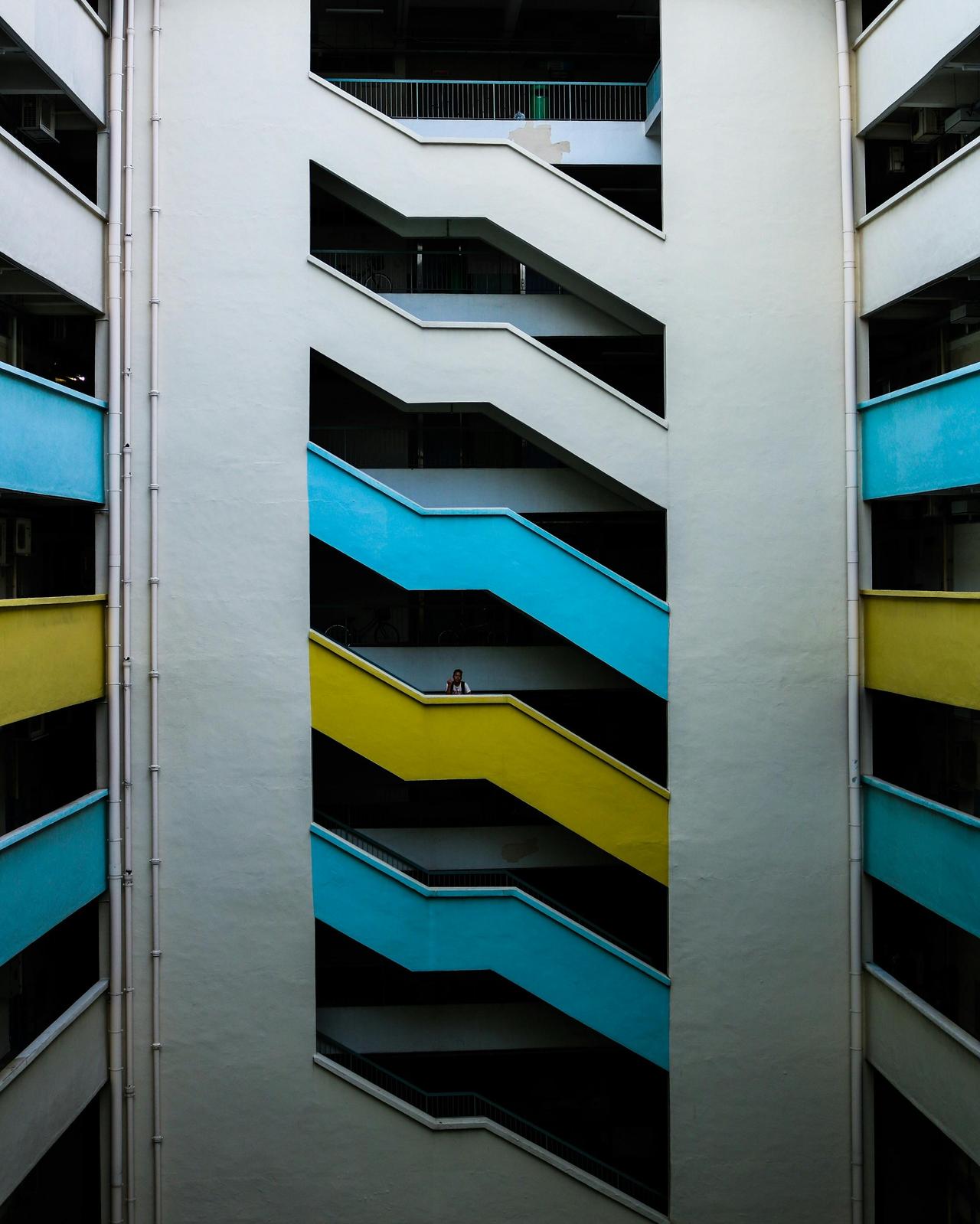
Stochastic volatility models are crucial in financial mathematics for capturing the dynamic behavior of volatility in financial markets, which is not constant but varies over time. A significant contribution to this field is “stochastic volatility modeling by Lorenzo Bergomi,” which has provided deep insights into how volatility can be modeled more accurately in complex financial environments. Bergomi’s work, particularly through his book “Smile Dynamics,” elaborates on advanced stochastic volatility models and their applications in pricing and hedging options.
Bergomi’s approach to stochastic volatility modeling focuses on improving the realism of volatility surfaces and the behavior of financial instruments under different market conditions. His models incorporate stochastic processes that allow volatility to evolve randomly over time, reflecting the real-world phenomena of volatility clustering and sudden jumps in financial markets. This modeling approach helps in better pricing derivatives and managing risk by accounting for the variable nature of market volatility.
The stochastic volatility models developed by Bergomi often use a combination of mathematical frameworks and empirical observations to enhance the accuracy of volatility forecasts and option pricing. These models are particularly valuable for traders and risk managers who need to understand and anticipate changes in market conditions. They provide tools for adjusting strategies in response to shifting volatility, leading to more informed decision-making and improved financial outcomes.
In summary, “stochastic volatility modeling by Lorenzo Bergomi” represents a significant advancement in the field of financial modeling, offering a sophisticated approach to understanding and managing the complexities of market volatility. His contributions have been instrumental in refining models that better align with observed market behaviors and improve the practical application of stochastic volatility concepts in finance.
Stochastic volatility models are essential tools in financial mathematics for pricing derivatives and managing risk. Unlike models that assume constant volatility, stochastic volatility models allow volatility to fluctuate over time, reflecting real market conditions more accurately. These models help in capturing the dynamic behavior of financial markets where volatility is not static but varies with time and market events.
Stochastic Volatility Modeling Insights
Overview of Stochastic Models
Stochastic volatility models account for the randomness in volatility, providing a framework for more realistic option pricing and risk management. These models are characterized by their ability to incorporate the randomness of volatility, which impacts the pricing of financial derivatives. The Heston model, for example, is a widely-used stochastic volatility model that captures the empirical features of financial markets, such as volatility clustering and mean reversion.
Key Model: Heston Model
The Heston model is popular in stochastic volatility modeling due to its ability to fit various market conditions. It describes the evolution of volatility as a mean-reverting process, which means that volatility tends to revert to a long-term average over time. This feature helps in capturing the observed behavior of volatility in financial markets.
Risk Management with Stochastic Volatility
Applications in Derivatives Pricing
Stochastic volatility models are extensively used in derivatives pricing, particularly for options. These models help in estimating the fair value of options by accounting for the varying levels of volatility. For instance, the Heston model can be used to price European options and other complex derivatives by incorporating both the stochastic nature of volatility and the underlying asset price dynamics.
Practical Considerations
In practice, implementing stochastic volatility models involves calibrating the model parameters to fit market data. This process requires advanced numerical techniques and software tools to ensure accurate model predictions. The choice of model and its parameters can significantly impact the accuracy of pricing and risk management decisions.
Key Takeaways
Understanding Volatility Dynamics
Stochastic volatility models provide a more comprehensive view of market behavior by allowing volatility to vary over time. This approach helps in improving the accuracy of option pricing and risk management strategies compared to models that assume constant volatility.
Effective Model Calibration
Accurate calibration of stochastic volatility models is crucial for effective derivatives pricing and risk management. By fitting the model parameters to market data, traders and analysts can enhance their ability to forecast market movements and manage financial risks more effectively.
Excited by What You've Read?
There's more where that came from! Sign up now to receive personalized financial insights tailored to your interests.
Stay ahead of the curve - effortlessly.