Statistical Arbitrage: A Quantitative Approach to Market Inefficiencies
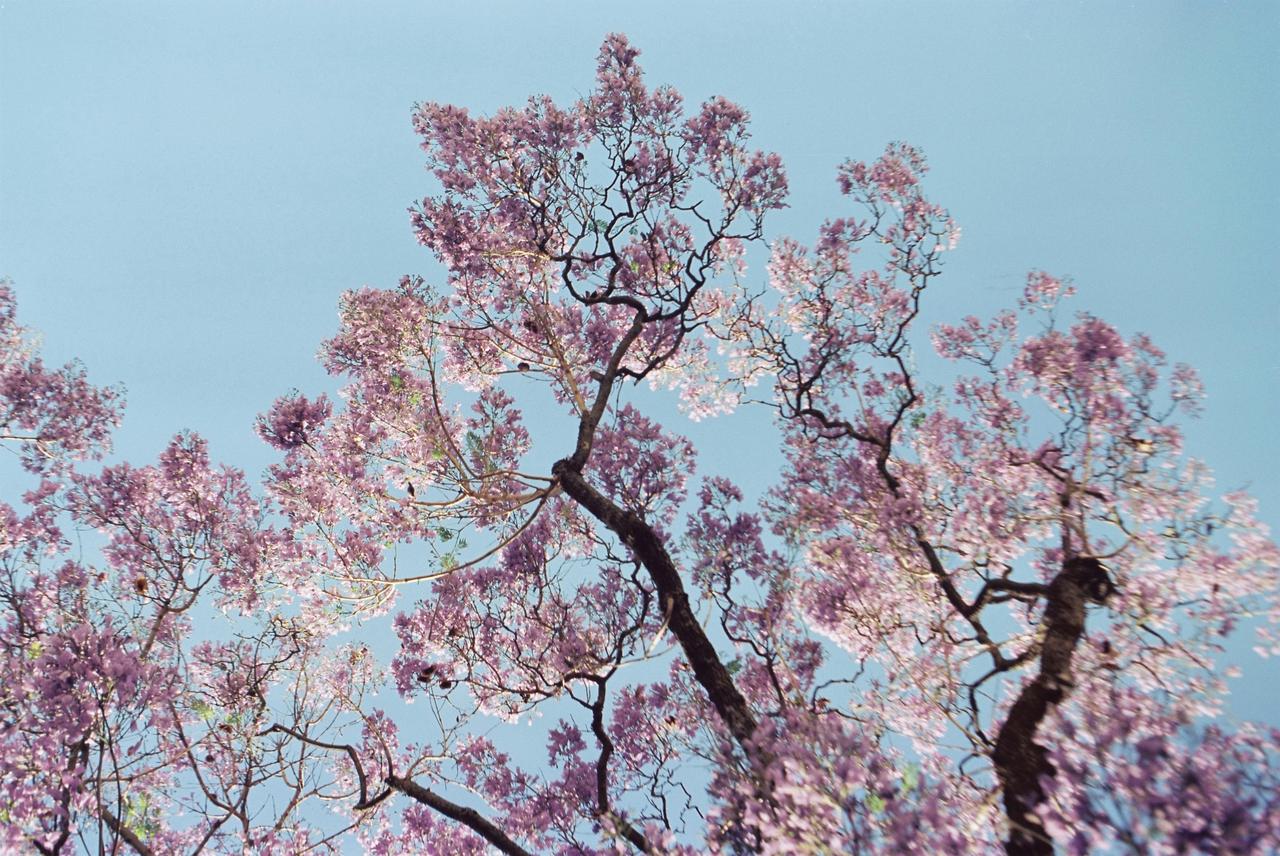
Statistical arbitrage is a sophisticated trading strategy that leverages quantitative methods to identify and exploit market inefficiencies. This approach, often employed by hedge funds and proprietary trading desks, relies on complex mathematical models and high-speed computing systems. In this article, we explore the nuances of statistical arbitrage, including its underlying principles, strategies employed, technological requirements, risks and challenges, and its evolving role in modern financial markets.
Principles of Statistical Arbitrage
Statistical arbitrage is based on the principle that historical price relationships between securities will continue in the future.
Exploiting Price Discrepancies
This strategy involves identifying pairs or groups of securities whose prices have historically moved together and are expected to continue doing so. When a temporary price discrepancy arises, traders buy the undervalued security and sell the overvalued one, expecting their prices to converge eventually.
Mean Reversion Theory
At the heart of statistical arbitrage is the mean reversion theory, which posits that prices and returns eventually move back towards the mean or average. This theory underpins many statistical arbitrage models.
Statistical Arbitrage Strategies
Traders use various strategies under the umbrella of statistical arbitrage, tailored to different market conditions and risk appetites.
Pairs Trading
Pairs trading is a common statistical arbitrage strategy where two co-integrated securities are traded. When the spread between their prices widens beyond a historical norm, traders take opposing positions in these securities.
Basket Trading
Basket trading involves creating a basket of undervalued securities and a basket of overvalued securities. Traders go long on the undervalued basket and short on the overvalued basket, betting on the convergence of their overall values.
Technological Requirements
Statistical arbitrage relies heavily on advanced technology for its execution.
High-Speed Computing and Data Analysis
Traders use high-speed computers capable of processing vast amounts of data to identify arbitrage opportunities. This involves analyzing historical price data, news feeds, and financial reports.
Algorithmic Trading Systems
Algorithms are used to automatically execute trades when certain pre-defined criteria are met. These algorithms are designed to be highly efficient and to execute trades within milliseconds.
Risks and Challenges
Despite its potential profitability, statistical arbitrage is not without its risks and challenges.
Market Risk and Model Failure
The biggest risk in statistical arbitrage is model failure. If the statistical model used to identify opportunities is flawed, it can lead to significant losses. Additionally, market conditions can change rapidly, making historical correlations unreliable.
Competition and Market Evolution
The field of statistical arbitrage is highly competitive, with many players seeking to exploit the same inefficiencies. As more traders enter the market, opportunities for arbitrage can diminish.
Evolution in Financial Markets
Statistical arbitrage has evolved significantly over time, adapting to changes in financial markets and technology.
Impact of Machine Learning and Artificial Intelligence
The integration of machine learning and AI into statistical arbitrage models has allowed for more sophisticated analysis and prediction of price movements.
Adapting to Changing Market Dynamics
As financial markets become more complex, statistical arbitrage strategies are continuously refined to adapt to new market conditions and regulations.
In conclusion, statistical arbitrage represents a cutting-edge approach to trading, leveraging quantitative analysis to capitalize on market inefficiencies. While it offers the potential for substantial profits, it requires sophisticated technological infrastructure, robust statistical models, and a thorough understanding of market dynamics. The field is highly competitive and constantly evolving, driven by advancements in technology and changes in market behavior. As such, it remains a domain largely reserved for institutional investors and highly skilled traders with the resources to develop and maintain complex trading systems.
Excited by What You've Read?
There's more where that came from! Sign up now to receive personalized financial insights tailored to your interests.
Stay ahead of the curve - effortlessly.