Robo Advisors Quantitative Methods Inside The Robots
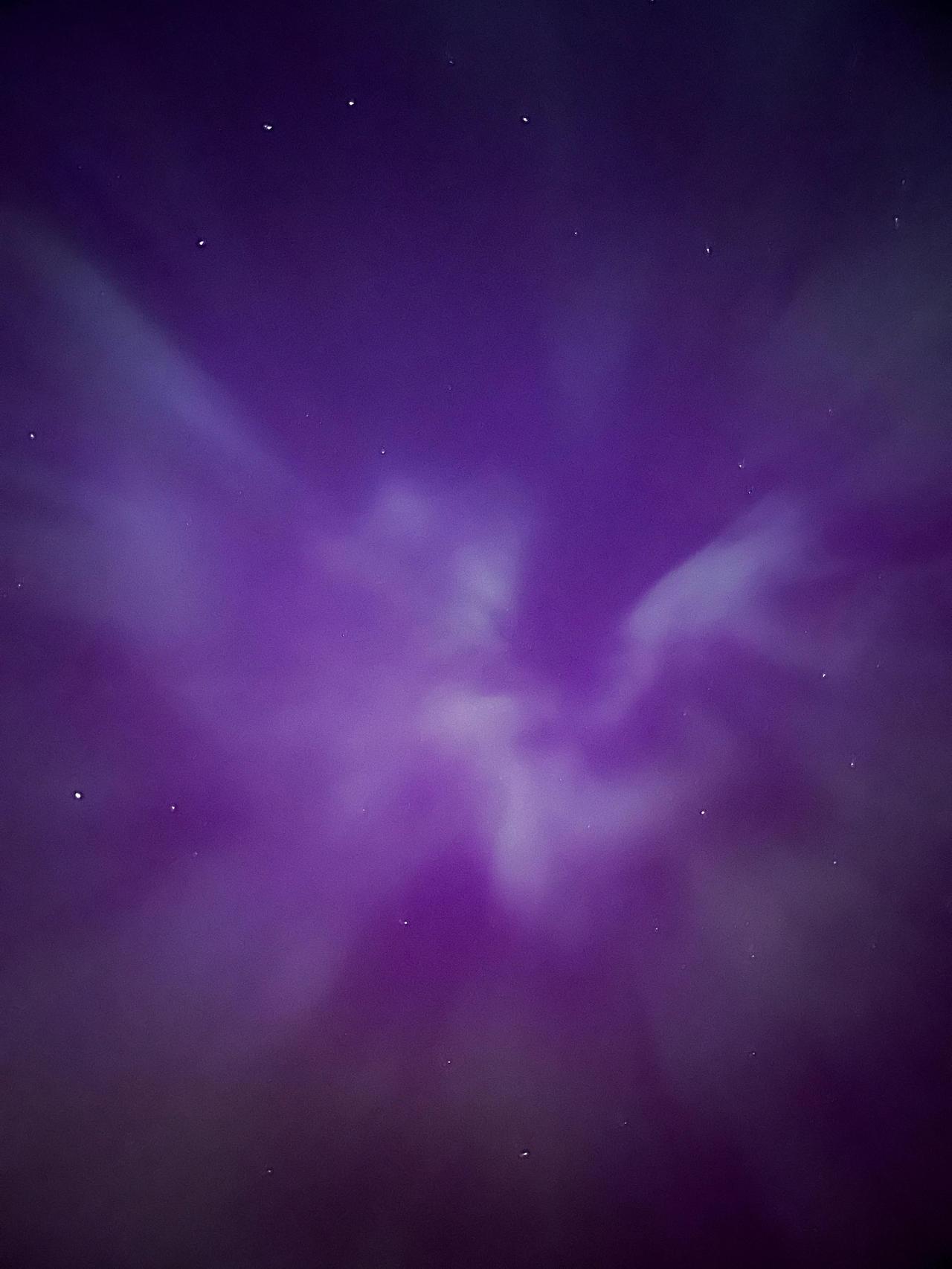
Robo-advisors represent a technological evolution in financial advisory services, leveraging advanced algorithms and automation to manage investment portfolios. A critical aspect of these platforms is the use of “robo advisors quantitative methods inside the robots,” which underpins their operational efficiency and effectiveness. These quantitative methods encompass various mathematical models and statistical techniques that enable robo-advisors to make informed investment decisions and optimize portfolio performance.
At the core of these quantitative methods is data analysis, which involves the collection and processing of vast amounts of financial data. Robo-advisors utilize algorithms to analyze historical market data, current economic conditions, and individual investor profiles to provide personalized investment recommendations. Techniques such as mean-variance optimization, which helps in constructing efficient portfolios by balancing risk and return, and Monte Carlo simulations, which assess the impact of different variables on investment outcomes, are commonly employed.
Additionally, machine learning models are increasingly integrated into robo-advisors to enhance their predictive accuracy. These models learn from historical data and identify patterns or trends that might not be immediately apparent through traditional analysis. For instance, predictive algorithms can forecast market movements or detect anomalies, enabling robo-advisors to adjust investment strategies proactively.
The incorporation of quantitative methods also extends to risk management and performance evaluation. By employing techniques such as Value at Risk (VaR) and stress testing, robo-advisors can gauge the potential risk of investment portfolios and adjust strategies accordingly to mitigate potential losses. Furthermore, performance metrics like Sharpe ratios and alpha measurements help in assessing the effectiveness of investment strategies over time.
Overall, the “robo advisors quantitative methods inside the robots” highlight the sophistication of these platforms, which combine data science, statistical analysis, and machine learning to provide high-quality financial advisory services. This integration of advanced quantitative techniques ensures that robo-advisors can deliver personalized, efficient, and dynamic investment management solutions to users, making them a valuable tool in modern financial planning.
Robo-advisors are digital platforms that provide automated financial services, using advanced algorithms to manage and optimize investment portfolios. These systems leverage quantitative methods to analyze vast amounts of financial data, making investment decisions and adjustments based on real-time market conditions and individual user profiles. The goal of robo-advisors is to offer cost-effective, efficient, and personalized investment management without the need for human intervention.
Quantitative Models in Robo-Advisors
Robo-advisors employ quantitative models to create and manage investment strategies. These models use statistical techniques and mathematical algorithms to predict market trends and optimize asset allocation. Techniques such as mean-variance optimization, factor models, and machine learning algorithms are commonly used to develop robust investment strategies that align with user goals and risk tolerance.
Portfolio Construction Algorithms
Portfolio construction is a critical aspect of robo-advisory services. Algorithms are used to construct diversified portfolios that minimize risk and maximize returns based on historical data and user inputs. By applying techniques like Modern Portfolio Theory (MPT) and optimization algorithms, robo-advisors can build portfolios that efficiently balance risk and reward.
Example of a Portfolio Optimization Model
In portfolio optimization, the goal is often to maximize the Sharpe ratio, which is calculated as:
\[ \text{Sharpe Ratio} = \frac{R_p - R_f}{\sigma_p} \]where:
- \( R_p \) is the expected portfolio return,
- \( R_f \) is the risk-free rate, and
- \( \sigma_p \) is the portfolio standard deviation.
Algorithms use this ratio to select the optimal mix of assets.
Risk Assessment and Management
Risk assessment is another crucial function of robo-advisors. These platforms use quantitative methods to evaluate and manage risk by analyzing market volatility, asset correlations, and historical performance. Risk management strategies include dynamic rebalancing, which adjusts the portfolio in response to changes in market conditions, and diversification to spread risk across different assets.
Machine Learning Enhancements
Machine learning techniques enhance the capabilities of robo-advisors by enabling them to learn from vast datasets and improve predictions over time. Algorithms can identify patterns, adapt to market changes, and refine investment strategies based on new data. Machine learning models contribute to more accurate forecasting and personalized financial advice.
“Quantitative methods and machine learning enhance robo-advisors’ ability to manage investments efficiently, providing users with tailored financial solutions.”
Evaluating Robo-Advisor Performance
Evaluating the performance of a robo-advisor involves analyzing key metrics such as returns, risk-adjusted returns, and adherence to investment goals. Performance reports provide insights into how well the robo-advisor’s algorithms are performing and help users make informed decisions about their investments.
By leveraging quantitative methods and advanced algorithms, robo-advisors offer sophisticated investment management solutions that are accessible and cost-effective, helping users achieve their financial goals with increased efficiency and precision.
Excited by What You've Read?
There's more where that came from! Sign up now to receive personalized financial insights tailored to your interests.
Stay ahead of the curve - effortlessly.