Resource Allocation For Irs Assisted Sgf Noma Transmission A Madrl Approach
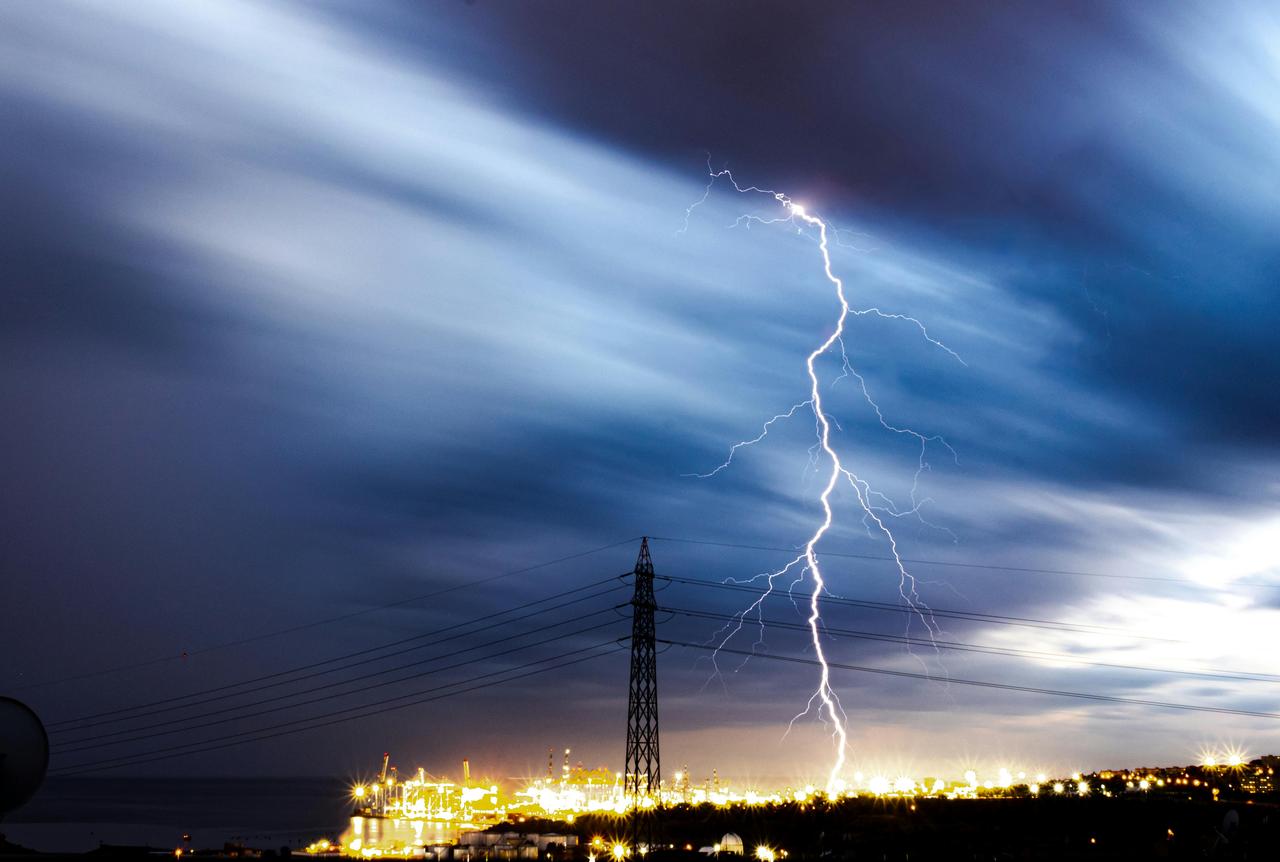
Resource allocation for IRS-assisted SGF NOMA transmission involves sophisticated methods to optimize the use of available resources in wireless communication systems, and a MADRL (Multi-Agent Deep Reinforcement Learning) approach is increasingly being explored to address these needs. In IRS-assisted (Intelligent Reflecting Surface) systems, the IRS is used to enhance signal transmission by reflecting signals in a controlled manner to improve coverage and capacity. When combined with SGF (Spectral Graph Filtering) and NOMA (Non-Orthogonal Multiple Access), these systems can significantly increase the efficiency of resource use.
SGF NOMA transmission aims to improve spectral efficiency by allowing multiple users to share the same frequency band simultaneously, with the help of advanced signal processing techniques. However, efficiently managing this shared resource requires effective allocation strategies to ensure that all users experience optimal performance. This is where the MADRL approach comes into play.
The MADRL approach involves training multiple agents using deep reinforcement learning techniques to make resource allocation decisions in a dynamic and distributed manner. Each agent represents a decision-making entity that interacts with its environment and learns to optimize the allocation of resources based on feedback from the system. In the context of IRS-assisted SGF NOMA transmission, MADRL can be used to adaptively adjust resource allocation in real-time, considering the varying conditions and requirements of different users.
By leveraging the MADRL approach, the system can achieve more efficient resource allocation by learning optimal strategies for handling interference, user demand, and channel conditions. This results in improved overall system performance, including better signal quality, reduced latency, and increased throughput. The ability to dynamically adapt to changing conditions and user needs is crucial for maintaining high performance in IRS-assisted SGF NOMA systems.
Thus, resource allocation for IRS-assisted SGF NOMA transmission, when approached with MADRL, offers a powerful method for optimizing resource use in advanced wireless networks, addressing the complex challenges of modern communication systems.
Resource allocation in IRS-assisted SGF NOMA transmission is a sophisticated area of study that addresses the efficient distribution of resources in advanced communication systems. IRS (Intelligent Reflecting Surface) technology enhances signal quality by dynamically adapting the environment to improve transmission. SGF (Super-Granular Frequency) and NOMA (Non-Orthogonal Multiple Access) further complicate the allocation process due to their unique requirements.
IRS-Assisted SGF NOMA Resource Allocation
IRS-Based Resource Management
IRS technology leverages programmable surfaces to optimize the transmission environment, enhancing the signal strength and coverage. In the context of SGF NOMA, IRS can be used to adjust the channel conditions dynamically. This approach helps to manage the interference and maximize the overall system capacity. The key to effective IRS-based resource management is to use real-time feedback from the network to adjust the reflecting elements and balance the signal distribution among users.
SGF NOMA Transmission Strategies
SGF NOMA combines super-granular frequency allocation with non-orthogonal access techniques. This strategy enables multiple users to share the same frequency band but at different power levels. The allocation process involves determining the optimal power distribution for each user and efficiently managing the frequency resources to reduce interference. The flexibility of SGF NOMA allows for high data rates and improved spectral efficiency.
MADRL Approach for Allocation
The Multi-Agent Deep Reinforcement Learning (MADRL) approach is employed to optimize resource allocation in complex scenarios like IRS-assisted SGF NOMA. MADRL algorithms enable agents to learn and adapt to dynamic network conditions by interacting with the environment and receiving feedback. This method allows for the development of sophisticated strategies that can handle varying user demands and channel conditions.
Key Challenges and Solutions
Challenge | Solution |
---|---|
Dynamic Channel Conditions | Adaptive IRS adjustments based on real-time data |
Interference Management | Optimized power control and frequency allocation |
Efficient Learning in Complex Scenarios | Advanced MADRL algorithms with high learning efficiency |
Advanced Techniques and Future Directions
Adaptive IRS Techniques
To further improve resource allocation, future research may focus on advanced adaptive techniques for IRS technology. These techniques would involve more precise control of the reflecting elements and better integration with network management systems.
Enhancing MADRL Algorithms
The development of more efficient MADRL algorithms can significantly enhance resource allocation. Research may focus on improving the learning speed and accuracy of these algorithms, allowing them to handle increasingly complex scenarios.
Integration with Emerging Technologies
Integrating IRS-assisted SGF NOMA with emerging technologies such as 5G and beyond could provide new opportunities for resource allocation. This integration would require adapting current techniques to new standards and capabilities.
Conclusion
Effective resource allocation for IRS-assisted SGF NOMA transmission involves a combination of advanced technologies and methods. By employing IRS for adaptive signal management, utilizing SGF NOMA for efficient frequency use, and leveraging MADRL for intelligent decision-making, these systems can achieve high performance and capacity. Future advancements in these areas will continue to drive improvements in communication efficiency and user experience.
Excited by What You've Read?
There's more where that came from! Sign up now to receive personalized financial insights tailored to your interests.
Stay ahead of the curve - effortlessly.