Regression Analysis An Intuitive Guide For Using And Interpreting Linear Models
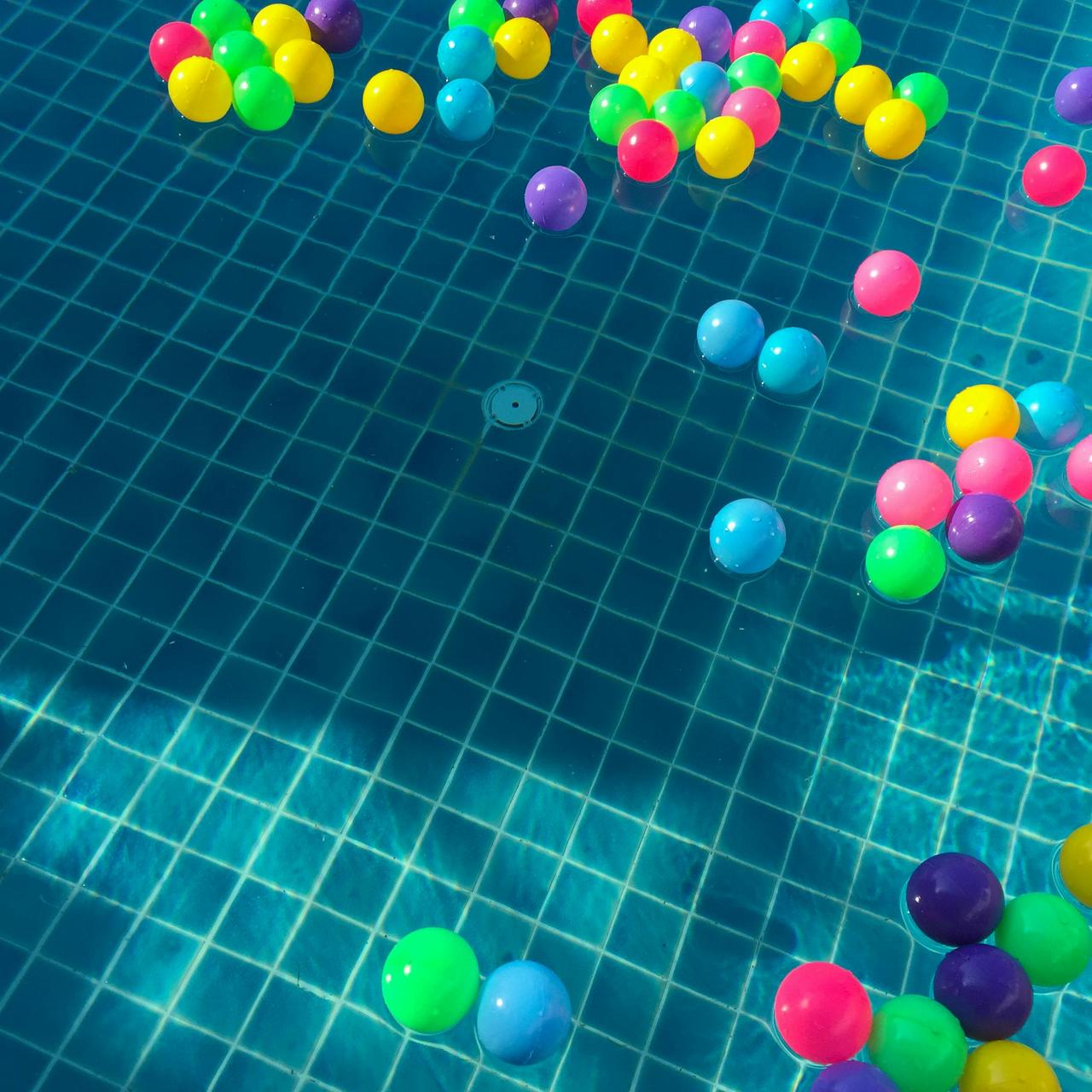
Regression analysis is a powerful statistical tool used to understand the relationship between variables and make predictions based on this understanding. In the context of “regression analysis an intuitive guide for using and interpreting linear models,” the focus is on simplifying the complex concepts of regression to make them more accessible and practical for users. Linear regression, the most basic form of regression analysis, examines how the dependent variable changes with the independent variable(s) and fits a linear equation to the observed data.
The intuitive guide approach to regression analysis involves breaking down the process into manageable steps. It begins with defining the variables and understanding the underlying assumptions of linear models, such as linearity, independence, and homoscedasticity. The guide then explains how to use data to fit a linear model, typically through the least squares method, which minimizes the sum of the squared differences between observed and predicted values.
Interpreting the results is another crucial aspect covered in the guide. This includes understanding key output metrics such as coefficients, which indicate the strength and direction of relationships between variables, and R-squared, which measures the proportion of variance in the dependent variable explained by the independent variables. The guide also addresses how to evaluate the model’s performance and validity through diagnostic checks and statistical tests.
By providing clear explanations and practical examples, “regression analysis an intuitive guide for using and interpreting linear models” helps users grasp how to apply linear regression techniques effectively and interpret the results meaningfully. This approach not only aids in performing regression analysis but also in making informed decisions based on the model’s findings, thereby enhancing both the application and understanding of linear models in various analytical contexts.
Regression analysis is a powerful statistical tool used to understand relationships between variables. It helps in predicting the value of one variable based on the value of another. By modeling these relationships, regression analysis enables us to make informed decisions and predictions. Linear regression, the most common form, fits a straight line to the data, allowing us to explore the linear relationship between the dependent and independent variables.
Interpreting Linear Regression Models
Understanding Model Coefficients
In linear regression, coefficients represent the strength and direction of the relationship between independent variables and the dependent variable. The slope coefficient indicates how much the dependent variable changes with a one-unit change in an independent variable. The intercept represents the value of the dependent variable when all independent variables are zero. These coefficients provide insights into the nature of the relationships modeled by the regression line.
Assessing Model Fit and Significance
To evaluate the effectiveness of a regression model, we use metrics such as R-squared and p-values. R-squared measures how well the model explains the variability in the dependent variable. A higher R-squared value indicates a better fit. P-values help determine the statistical significance of each coefficient. A low p-value suggests that the corresponding variable significantly contributes to the model. Together, these metrics help in assessing whether the model is a good representation of the data.
Visualizing Regression Results
Plotting Regression Lines
Visualizing regression results can enhance our understanding of the model. Plotting the regression line on a scatter plot allows us to see how well the line fits the data points. This visualization helps in identifying patterns, trends, and outliers. By comparing the actual data points with the fitted line, we can visually assess the model’s accuracy and reliability.
Residual Analysis
Residual analysis involves examining the differences between the observed and predicted values of the dependent variable. Plotting residuals can reveal patterns that might suggest model issues, such as non-linearity or heteroscedasticity. Ideally, residuals should be randomly scattered around zero, indicating that the model’s assumptions are satisfied. Residual plots help in diagnosing and improving model performance.
Practical Considerations
Assumptions of Linear Regression
Linear regression models are based on several key assumptions, including linearity, independence, homoscedasticity, and normality of residuals. Violations of these assumptions can affect the validity of the model. It is important to check these assumptions and apply appropriate techniques, such as transformations or alternative models, to address any issues.
Model Diagnostics and Improvements
Model diagnostics involve evaluating the model’s performance and making necessary improvements. Techniques such as cross-validation and stepwise regression can help refine the model and enhance its predictive accuracy. By continually assessing and improving the model, we can ensure that it provides reliable and meaningful insights.
By understanding these aspects of regression analysis, you can effectively use and interpret linear models, leading to better data-driven decisions and predictions.
Excited by What You've Read?
There's more where that came from! Sign up now to receive personalized financial insights tailored to your interests.
Stay ahead of the curve - effortlessly.