Predictive Modeling Of Hamstring Strain Injuries In Elite Australian Footballers
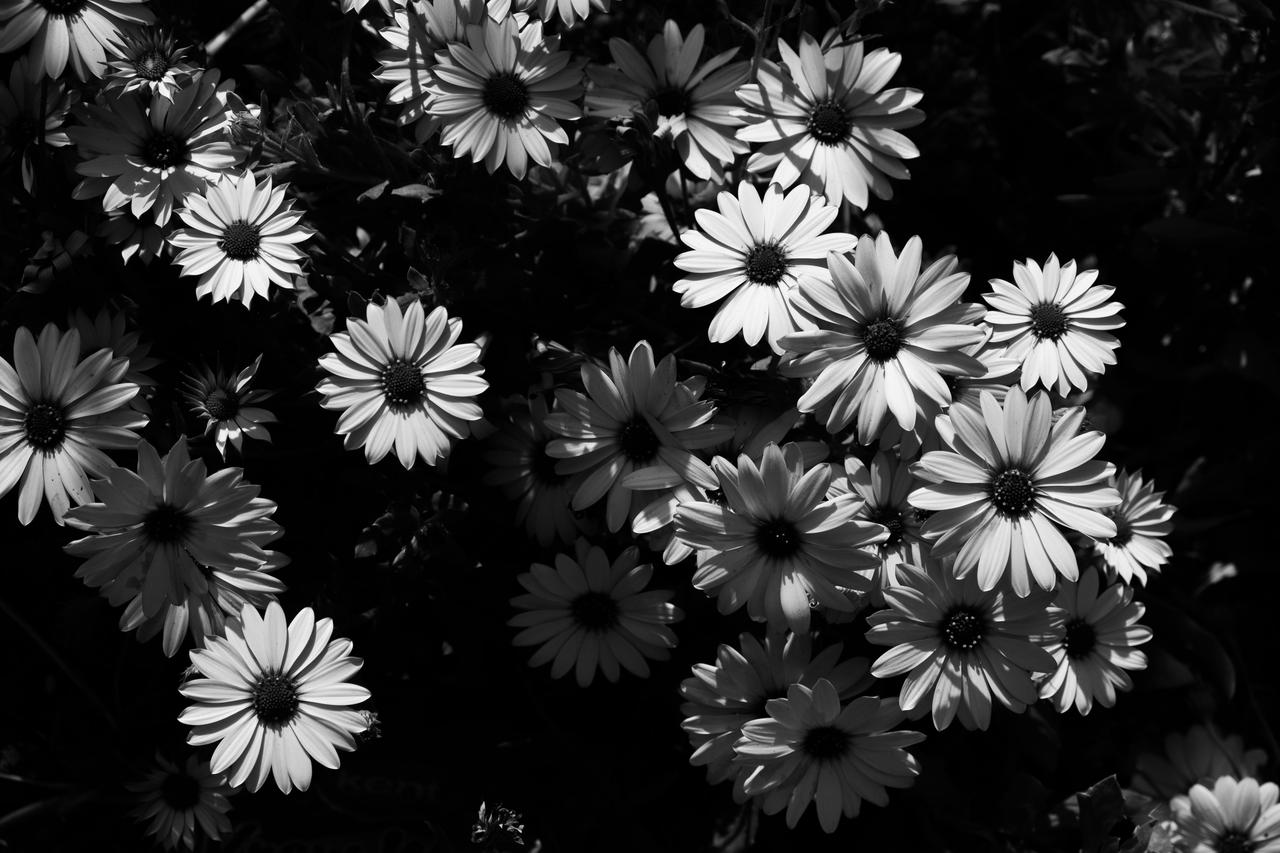
Predictive modeling in the context of sports science can offer valuable insights into injury prevention and management. A notable application of this technique is in the field of sports medicine, particularly regarding the predictive modeling of hamstring strain injuries in elite Australian footballers. This specific use of predictive modeling involves analyzing various data points to forecast the likelihood of hamstring strains, which are a common and debilitating injury in football. By utilizing historical injury data, player performance metrics, training loads, and biomechanical assessments, researchers and practitioners can develop models that predict the risk of such injuries.
The process begins with collecting and integrating data from different sources, such as match play, training sessions, and medical records. Predictive algorithms then analyze this data to identify patterns and factors associated with an increased risk of hamstring strains. For instance, these models may consider variables such as the intensity of physical exertion, previous injury history, muscle strength, flexibility, and fatigue levels. Advanced statistical methods and machine learning techniques are often employed to enhance the accuracy of these predictions.
The goal of predictive modeling of hamstring strain injuries in elite Australian footballers is not only to forecast potential injuries but also to inform preventive strategies and interventions. By accurately predicting when and which players are at higher risk, teams can adjust training programs, optimize recovery protocols, and implement targeted exercises to strengthen vulnerable areas. This approach helps in reducing the incidence of injuries, improving player performance, and extending careers in professional football.
Predictive modeling in liquidity involves using statistical and machine learning techniques to forecast the availability and distribution of financial liquidity within markets. This process is essential for understanding how liquidity fluctuates over time and for managing risks associated with liquidity shortages. Models are designed to predict liquidity conditions based on historical data, market variables, and economic indicators, allowing institutions to better prepare for and respond to changes in liquidity.
Liquidity Forecasting Models and Techniques
Statistical Methods for Predicting Liquidity
Statistical methods play a crucial role in predictive modeling for liquidity. Common techniques include time series analysis, regression models, and volatility forecasting. Time series models, such as ARIMA (AutoRegressive Integrated Moving Average), are used to analyze historical liquidity data and forecast future conditions based on observed patterns.
Table: Common Statistical Models for Liquidity Prediction
Model | Description | Use Case |
---|---|---|
ARIMA | Time series forecasting with trend and seasonality | Short-term liquidity forecasting |
GARCH | Generalized Autoregressive Conditional Heteroskedasticity | Volatility estimation |
VAR | Vector Autoregression | Multivariate time series analysis |
Machine Learning Approaches to Liquidity
Machine Learning Techniques for Enhanced Forecasting
Machine learning algorithms can enhance predictive accuracy by capturing complex patterns in liquidity data. Techniques such as random forests, support vector machines, and neural networks are applied to model non-linear relationships and interactions between different variables influencing liquidity. These methods often improve prediction performance compared to traditional statistical approaches.
Quote on Machine Learning in Liquidity Modeling
“Machine learning techniques offer the potential to uncover complex patterns in liquidity data, improving forecasting accuracy beyond traditional methods.”
Example: Neural Network Model for Liquidity Prediction
An example of a neural network model used in liquidity prediction is the Long Short-Term Memory (LSTM) network, which is particularly effective for sequential data and time series forecasting. The model learns from historical liquidity data to make predictions about future liquidity conditions.
Integration of Predictive Models in Risk Management
Application of Models in Financial Risk Management
Predictive models for liquidity are integral to financial risk management strategies. By forecasting liquidity needs and potential shortages, financial institutions can make informed decisions about capital allocation, risk mitigation, and liquidity management. These models help institutions anticipate market conditions and adjust their strategies accordingly.
Mathematical Formulation of Liquidity Prediction
To illustrate a predictive model for liquidity, consider the following simplified linear regression formula:
\[ L_t = \beta_0 + \beta_1 X_t + \beta_2 Z_t + \epsilon_t \]where:
- \( L_t \) represents liquidity at time \( t \),
- \( X_t \) and \( Z_t \) are predictors of liquidity,
- \( \beta_0, \beta_1, \beta_2 \) are coefficients,
- \( \epsilon_t \) is the error term.
This formula helps in estimating liquidity based on various influencing factors and historical data.
Predictive modeling in liquidity provides valuable insights for financial institutions, allowing them to anticipate and manage liquidity risks effectively. By employing both statistical and machine learning techniques, institutions can enhance their forecasting capabilities and improve their overall liquidity management strategies.
Excited by What You've Read?
There's more where that came from! Sign up now to receive personalized financial insights tailored to your interests.
Stay ahead of the curve - effortlessly.