Predictive Model Assessment In Pls-Sem Guidelines For Using Plspredict
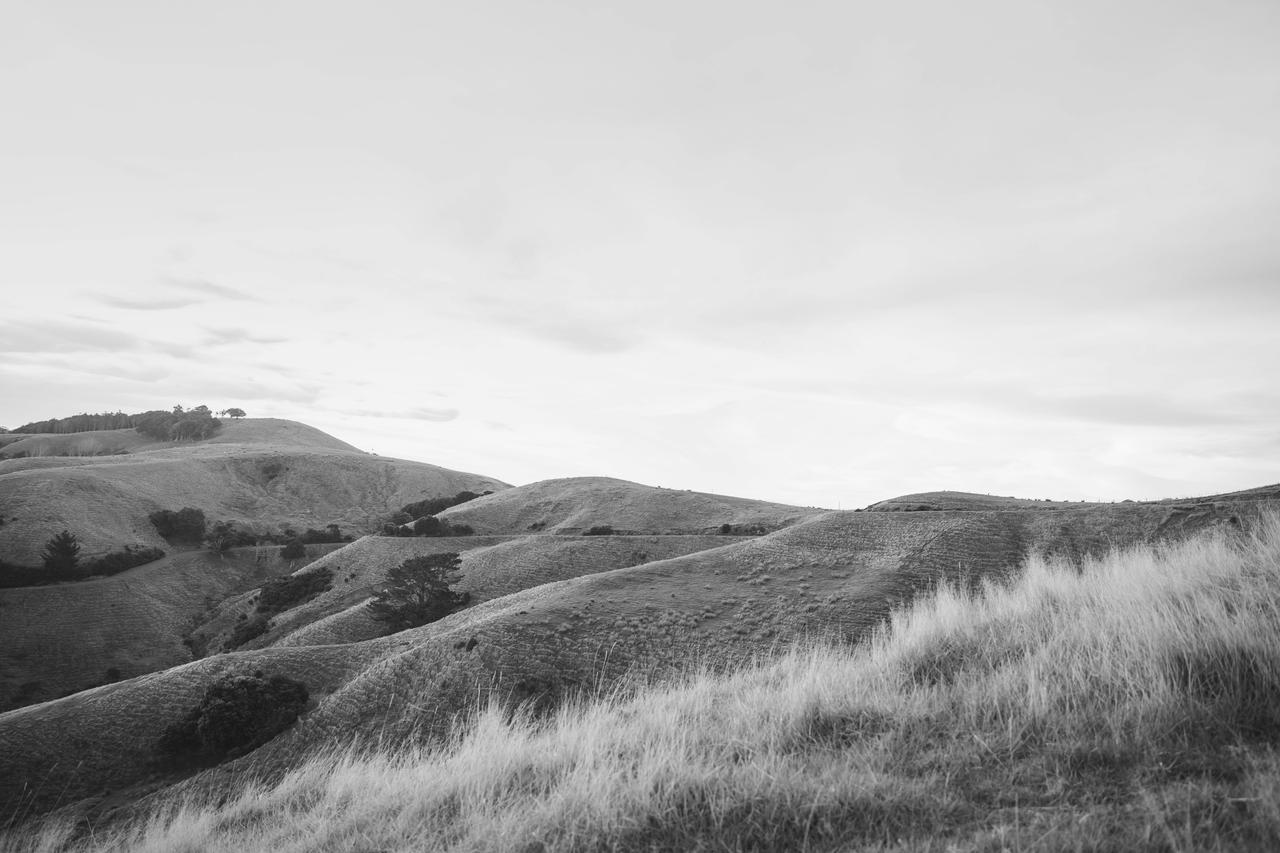
Predictive modeling in liquidity involves forecasting future liquidity needs and behaviors based on historical and current data. In this context, the “predictive model assessment in PLS-SEM guidelines for using plspredict” is a crucial aspect. Partial Least Squares Structural Equation Modeling (PLS-SEM) is a popular technique for modeling complex relationships between variables, and plspredict is a tool designed to assess the predictive performance of models developed using PLS-SEM.
The guidelines for using plspredict focus on evaluating how well a model can predict new or unseen data, which is essential for ensuring the robustness and reliability of predictive models. In PLS-SEM, model assessment typically involves examining both the quality of the structural relationships and the measurement model’s reliability. However, to fully understand a model’s predictive capability, it is important to go beyond traditional validation metrics and employ tools like plspredict.
Plspredict provides guidelines for assessing predictive accuracy by using cross-validation techniques, which involve partitioning the data into training and testing sets. This allows for a more comprehensive evaluation of how well the model performs on new data, which is particularly important for liquidity forecasting where accurate predictions can impact decision-making in financial management.
In practice, applying the “predictive model assessment in PLS-SEM guidelines for using plspredict” involves several steps. First, you would fit your model using PLS-SEM, and then use plspredict to assess its predictive performance. This involves checking metrics such as prediction errors and assessing how well the model generalizes to different data sets. By following these guidelines, you can ensure that the predictive models used in liquidity management are not only theoretically sound but also practically effective in forecasting and decision-making.
Overall, the integration of predictive model assessment in PLS-SEM with tools like plspredict helps enhance the reliability of predictive modeling in liquidity, providing a rigorous framework for evaluating and improving forecasting models.
Predictive modeling in liquidity involves using statistical and machine learning techniques to forecast liquidity conditions and manage financial risks. One key tool in this domain is Partial Least Squares Structural Equation Modeling (PLS-SEM), which allows for the analysis of complex relationships between variables. PLS-SEM is particularly useful for predictive modeling due to its ability to handle small sample sizes and its flexibility in modeling complex constructs.
Model Assessment Guidelines in PLS-SEM
When using PLS-SEM for predictive modeling, assessing the model’s performance is crucial to ensure reliable and actionable insights. The following guidelines help in evaluating predictive models using PLS-SEM:
- PLSPredict Evaluation: This tool provides predictive performance measures for PLS-SEM models, such as RMSE (Root Mean Squared Error) and MAE (Mean Absolute Error), which are essential for assessing the accuracy of predictions.
- Predictive Power Analysis: Evaluating how well the model predicts out-of-sample data, which involves splitting the data into training and test sets to measure predictive accuracy.
Assessing Predictive Accuracy with PLSPredict
PLSPredict provides several metrics for evaluating model accuracy:
- RMSE and MAE: These metrics indicate how close the predicted values are to the actual values, with lower values reflecting better predictive performance.
- Cross-Validation: Using cross-validation techniques to validate the model’s performance on different subsets of data, thereby ensuring that the model generalizes well to new data.
Guidelines for Implementing PLS-SEM Models
When implementing PLS-SEM models for predictive purposes, consider the following:
- Model Specification: Define clear hypotheses and relationships between latent variables and their indicators. Proper specification is crucial for the validity of the model.
- Data Preparation: Ensure that data is preprocessed appropriately, including handling missing values and normalizing data if necessary.
- Performance Metrics: Utilize various performance metrics provided by PLSPredict to evaluate the model comprehensively.
Example of Model Evaluation Metrics
To illustrate, here’s a brief example of how to use PLSPredict metrics:
Root Mean Squared Error (RMSE):
\[ \text{RMSE} = \sqrt{\frac{1}{n} \sum_{i=1}^{n} (y_i - \hat{y}_i)^2} \]where \( y_i \) are the actual values and \( \hat{y}_i \) are the predicted values.
Mean Absolute Error (MAE):
\[ \text{MAE} = \frac{1}{n} \sum_{i=1}^{n} |y_i - \hat{y}_i| \]
Conclusion
Predictive modeling in liquidity using PLS-SEM can offer valuable insights into financial risks and liquidity management. By following the guidelines for model assessment and utilizing tools like PLSPredict, practitioners can enhance the accuracy and reliability of their predictive models, ultimately leading to better decision-making and risk management.
“Accurate predictive modeling in liquidity is crucial for effective financial risk management, and PLS-SEM provides robust tools for assessing and improving model performance.”
Excited by What You've Read?
There's more where that came from! Sign up now to receive personalized financial insights tailored to your interests.
Stay ahead of the curve - effortlessly.