Portfolio Rebalancing Experiments Using The Quantum Alternating Operator Ansatz
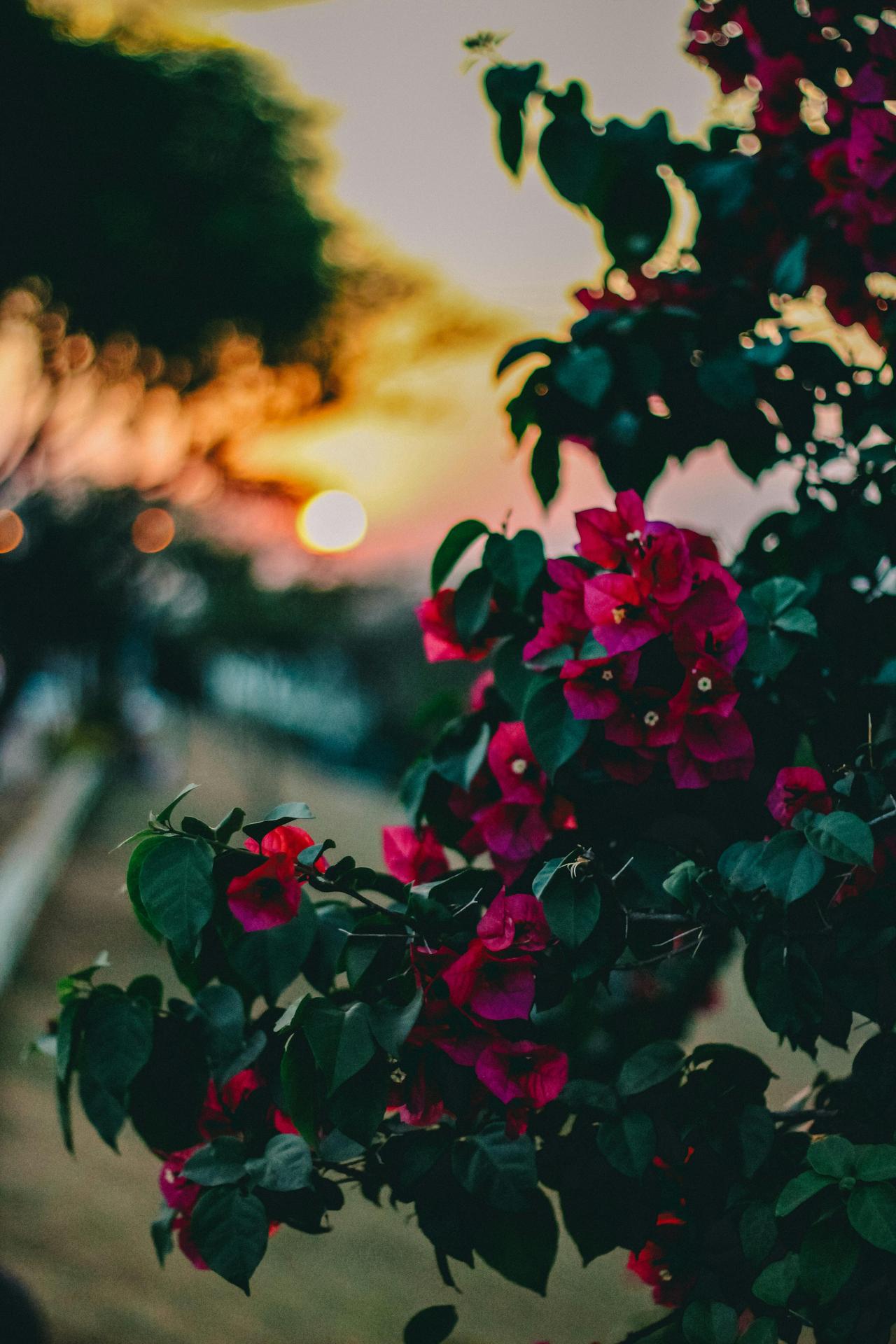
Portfolio rebalancing is a crucial process in investment management, ensuring that a portfolio maintains its intended asset allocation and risk profile over time. Recently, the application of advanced quantum computing techniques to financial optimization problems has gained attention, particularly in portfolio rebalancing. One area of research involves portfolio rebalancing experiments using the quantum alternating operator ansatz. This approach leverages the principles of quantum mechanics to address the complexities involved in optimizing portfolio allocations.
The quantum alternating operator ansatz is a method used within quantum computing to solve optimization problems. It involves constructing a quantum circuit that iteratively applies a series of operators to find an optimal solution. In the context of portfolio rebalancing, this technique is used to explore and optimize various asset allocations by encoding the problem into a quantum system. The quantum alternating operator ansatz allows for the exploration of a vast solution space more efficiently than classical methods by leveraging quantum parallelism.
Portfolio rebalancing experiments using the quantum alternating operator ansatz typically involve formulating the portfolio optimization problem in terms of quantum operations. This includes defining the cost function, which often reflects the portfolio’s risk and return characteristics, and then implementing the ansatz to minimize or maximize this function. The quantum algorithm iteratively refines the solution by adjusting the quantum state, aiming to find the optimal asset weights that achieve the desired balance of risk and return.
These experiments demonstrate the potential of quantum computing to handle complex and high-dimensional optimization problems more efficiently than classical techniques. By applying the quantum alternating operator ansatz to portfolio rebalancing, researchers aim to enhance the precision and speed of finding optimal investment strategies. This approach represents a significant advancement in financial optimization, combining quantum computational techniques with traditional portfolio management practices to potentially revolutionize how portfolios are managed and adjusted in response to market changes.
Portfolio rebalancing is a strategic process used to maintain an investment portfolio’s desired asset allocation over time. This process involves periodically adjusting the portfolio to realign with the investor’s risk tolerance, investment goals, and market conditions. Effective rebalancing ensures that the portfolio remains consistent with the investor’s objectives and helps manage risk by preventing any single asset class from dominating the portfolio.
Quantum Alternating Operator Ansatz (QAOA) for Portfolio Rebalancing
The Quantum Alternating Operator Ansatz (QAOA) has emerged as a novel approach for optimizing portfolio rebalancing. QAOA leverages quantum computing to solve complex optimization problems, such as those involved in rebalancing a portfolio. By using quantum algorithms, investors can potentially achieve more efficient and precise portfolio adjustments compared to classical methods. This approach models the portfolio optimization problem as a quantum circuit, where the goal is to find the optimal allocation of assets that maximizes returns while minimizing risk.
Experimental Applications of QAOA in Rebalancing
Recent experiments have demonstrated the effectiveness of QAOA in portfolio rebalancing. These experiments involve creating quantum circuits that represent different portfolio configurations and using quantum algorithms to evaluate and optimize these configurations. By exploring various quantum states, the QAOA approach can identify optimal rebalancing strategies that might be difficult to find using traditional methods. These advancements highlight the potential for quantum computing to revolutionize portfolio management and improve investment outcomes.
Benefits of Quantum Approaches in Rebalancing
Using quantum computing for portfolio rebalancing offers several potential benefits:
- Enhanced Efficiency: Quantum algorithms can process complex calculations faster than classical methods, leading to quicker rebalancing decisions.
- Increased Accuracy: Quantum approaches can handle larger datasets and more intricate optimization problems, potentially providing more accurate solutions.
- Innovative Strategies: Quantum computing introduces new methodologies for solving optimization problems, leading to innovative investment strategies and techniques.
Future Directions and Challenges
Despite the promising results, there are challenges to overcome before quantum approaches become mainstream in portfolio management. These include:
- Quantum Hardware Limitations: Current quantum computers are limited in their capacity and reliability, which may affect the practicality of QAOA in real-world scenarios.
- Algorithm Development: Continued development of quantum algorithms and techniques is necessary to fully realize the potential of QAOA in portfolio rebalancing.
- Integration with Classical Systems: Combining quantum and classical methods effectively requires advancements in hybrid computing approaches and system integration.
Summary
Portfolio rebalancing is essential for maintaining an optimal asset allocation. The integration of quantum computing, particularly through the QAOA approach, represents a significant advancement in optimizing this process. While experimental applications show promise, further development and overcoming technical challenges are crucial for broader adoption.
Tables and Quotes
Portfolio Rebalancing Strategies
Approach | Description | Benefits |
---|---|---|
Classical Methods | Traditional optimization techniques for rebalancing | Well-established and widely used |
Quantum Alternating Ansatz (QAOA) | Quantum computing approach for optimization | Enhanced efficiency and accuracy |
Notable Quotes
“The potential of quantum computing to transform portfolio management is immense, offering new ways to optimize rebalancing strategies.” — Financial Innovation Journal
Mathematical Representation
For a simplified quantum circuit optimization problem, the goal is to maximize the objective function \( f(x) \), subject to constraints:
\[ \text{maximize } f(x) \text{ subject to } g_i(x) \leq 0 \]Where:
- \( f(x) \) = Objective function representing portfolio returns
- \( g_i(x) \) = Constraints related to risk and asset allocation
Exploring these advanced techniques can offer valuable insights and improvements in portfolio management strategies.
Excited by What You've Read?
There's more where that came from! Sign up now to receive personalized financial insights tailored to your interests.
Stay ahead of the curve - effortlessly.