Nonparametric Methods For Changepoint Problems
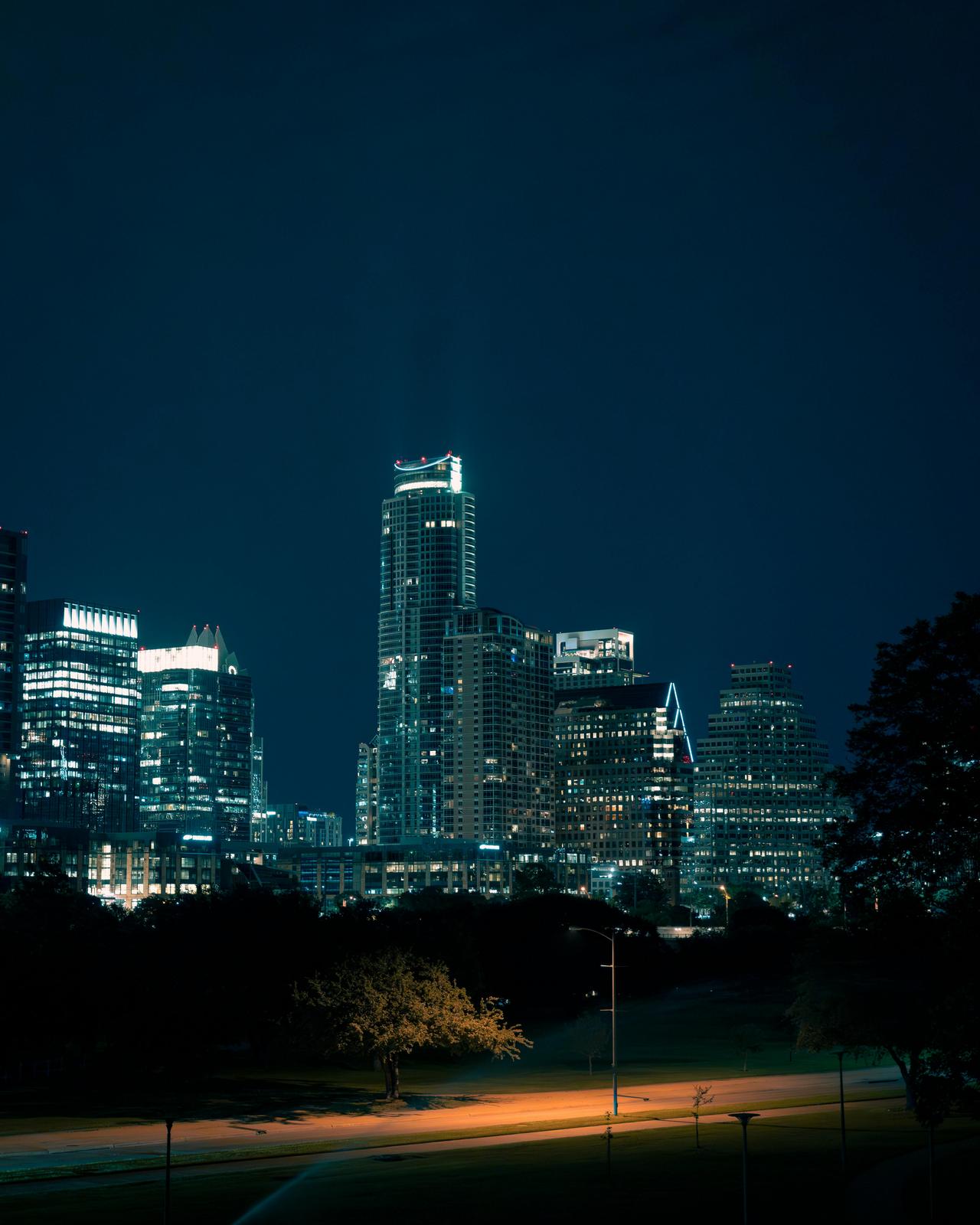
In the realm of statistical analysis, “nonparametric methods for changepoint problems” play a crucial role in identifying and addressing shifts in data patterns without assuming a specific parametric model. These methods are particularly valuable when dealing with changepoint problems, where the objective is to detect points in time where the statistical properties of a process change significantly. Nonparametric approaches are advantageous because they do not rely on predefined distributional assumptions about the data, making them flexible and robust in various scenarios.
Nonparametric methods for changepoint problems include techniques that can adapt to the data’s inherent characteristics without imposing strict parametric constraints. One common approach is the use of kernel-based methods, which involve smoothing techniques to estimate the underlying distribution of the data. By examining changes in these estimates, it is possible to identify potential changepoints where significant shifts occur. Another popular method is the use of resampling techniques such as bootstrapping, which helps in assessing the stability of the changepoint estimates by generating multiple simulated samples from the observed data.
Additionally, nonparametric methods such as the CUSUM (Cumulative Sum) technique and the use of rank-based statistics offer valuable tools for detecting changepoints. The CUSUM method monitors cumulative changes in the data to identify shifts, while rank-based methods assess changes in the order or ranking of data points, providing a robust alternative when traditional parametric tests may not be applicable.
These nonparametric techniques are particularly useful in practical applications where data does not conform to standard distributional assumptions or where the underlying process is complex and multifaceted. By utilizing nonparametric methods for changepoint problems, analysts can gain insights into structural changes in data with greater flexibility and accuracy, accommodating a wide range of real-world scenarios.
Non-parametric methods offer a flexible approach to statistical analysis, especially when the underlying data does not conform to traditional parametric assumptions. These methods are particularly useful in scenarios where the distribution of data is unknown or does not fit known distributions. They rely on fewer assumptions and can provide robust results even with limited data.
Non-parametric Changepoint Detection
Detecting Changepoints
Changepoint Analysis
Non-parametric methods for changepoint detection are crucial when identifying points in time where the statistical properties of a time series change. These methods do not assume a specific distribution and can adapt to various types of data. For instance, the CUSUM (Cumulative Sum) method is widely used for its simplicity and effectiveness in detecting shifts in mean.
Techniques Used
- CUSUM Test: This technique monitors the cumulative sum of deviations from a target value to detect shifts.
- Kernel-Based Methods: Kernel methods smooth the data to identify changes in distribution more robustly.
Practical Applications
Financial Market Analysis
In financial markets, non-parametric methods can be employed to detect changes in volatility or mean returns, which are crucial for risk management and trading strategies.
Quality Control
Manufacturing industries use these methods to detect changes in production processes, ensuring quality control and reducing defects.
Non-parametric Test Examples
Kolmogorov-Smirnov Test
The Kolmogorov-Smirnov test compares the distribution of a sample with a reference distribution or another sample to detect deviations. This test is non-parametric and does not assume any specific distribution.
Mann-Whitney U Test
The Mann-Whitney U test is used to compare differences between two independent samples. It is particularly useful when the data does not meet the assumptions required for parametric tests.
Mathematical Formulation
Non-parametric Changepoint Model
In a changepoint model, let \( X_1, X_2, \ldots, X_n \) be the observed data. The objective is to identify a changepoint \( \tau \) that maximizes the likelihood of observing the data split into two segments with different distributions.
The likelihood function for a changepoint model can be expressed as:
\[ L(\tau) = P(X_1, \ldots, X_\tau) \times P(X_{\tau+1}, \ldots, X_n) \]where \( P(\cdot) \) represents the probability distribution of the data segments before and after the changepoint \( \tau \).
Example Calculation
For a time series data set, applying a non-parametric test like the CUSUM involves calculating cumulative sums and analyzing changes to detect potential changepoints.
Example Visualizations
Detection Chart
A typical visualization for changepoint detection might include a plot of the cumulative sum of deviations from the mean. Sudden jumps in the plot indicate potential changepoints.
Kernel Density Estimate
A kernel density estimate plot can show changes in the distribution of the data, highlighting where shifts occur.
By leveraging non-parametric methods, analysts and researchers can effectively identify changepoints and make informed decisions based on the behavior of their data, free from the constraints of parametric assumptions.
Excited by What You've Read?
There's more where that came from! Sign up now to receive personalized financial insights tailored to your interests.
Stay ahead of the curve - effortlessly.