Natural Language Processing Previous Year Question Papers Jntu
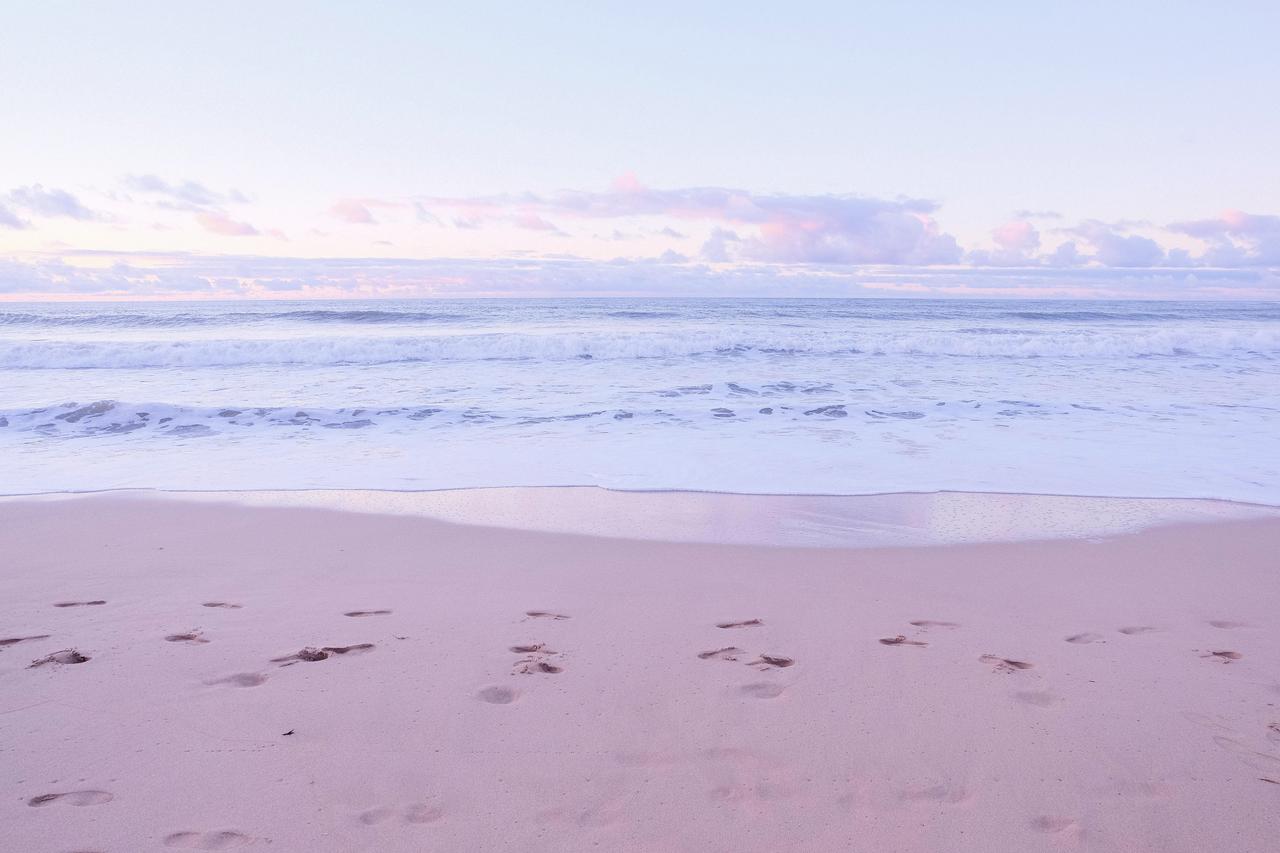
Natural Language Processing (NLP) is a critical field within artificial intelligence that focuses on the interaction between computers and human language. For students pursuing studies in NLP, particularly those at Jawaharlal Nehru Technological University (JNTU), reviewing past examination materials can be incredibly beneficial. The “natural language processing previous year question papers JNTU” serve as a valuable resource for understanding the types of questions typically asked, the structure of the exams, and the key topics covered in the course.
These previous year question papers provide insights into various aspects of NLP, such as syntax and semantic analysis, machine translation, speech recognition, and text mining. By analyzing these papers, students can identify recurring themes and areas of focus that are frequently tested. This allows them to tailor their study efforts more effectively and gain a deeper understanding of core concepts.
Additionally, the question papers often include both theoretical and practical questions, which are crucial for grasping the application of NLP techniques in real-world scenarios. For instance, students might encounter questions related to algorithms for part-of-speech tagging, named entity recognition, or sentiment analysis. Understanding these questions and the expected answers can guide students in developing a comprehensive approach to problem-solving in NLP.
Moreover, reviewing the “natural language processing previous year question papers JNTU” can also help students familiarize themselves with the examination format, such as the types of questions (e.g., multiple-choice, short answer, or problem-solving) and the distribution of marks. This knowledge is essential for effective time management during exams.
In summary, accessing and studying the “natural language processing previous year question papers JNTU” provides a practical way for students to prepare for their exams, offering them a clearer picture of the subject’s examination framework and content.
Natural Language Processing (NLP) is a field of artificial intelligence that focuses on the interaction between computers and human language. It aims to enable computers to understand, interpret, and generate human language in a way that is both meaningful and useful. NLP is integral to various applications such as speech recognition, machine translation, and sentiment analysis.
Natural Language Processing Applications
Speech Recognition Systems
Speech recognition technology converts spoken language into text. This application of NLP is widely used in virtual assistants, transcription services, and voice-controlled systems. It involves complex algorithms to process and understand human speech patterns and nuances.
Machine Translation Technologies
Machine translation refers to the automatic translation of text from one language to another. NLP techniques are employed to handle syntactic and semantic differences between languages, ensuring accurate translations. This technology underpins services like Google Translate and DeepL.
Sentiment Analysis Methods
Sentiment analysis involves determining the emotional tone behind a series of words. It is commonly used in social media monitoring and customer feedback analysis. By analyzing text data, NLP algorithms can classify sentiments as positive, negative, or neutral, providing insights into public opinion and customer satisfaction.
“Natural Language Processing enables computers to interact with human language in ways that were previously thought impossible, revolutionizing how we communicate with technology.”
Advanced NLP Techniques
Named Entity Recognition (NER)
Named Entity Recognition is an NLP technique used to identify and classify entities in text, such as names of people, organizations, or locations. This process is crucial for information extraction and helps in organizing and structuring data from unstructured sources.
Part-of-Speech Tagging (POS)
Part-of-Speech Tagging assigns grammatical categories to words in a sentence, such as nouns, verbs, adjectives, etc. This technique aids in understanding the syntactic structure of sentences and is foundational for more complex NLP tasks like parsing and machine translation.
Dependency Parsing
Dependency parsing involves analyzing the grammatical structure of a sentence to establish relationships between words. This technique helps in understanding the context and meaning of sentences, improving the accuracy of various NLP applications.
NLP and Machine Learning
Recent advancements in NLP have been driven by machine learning techniques, particularly deep learning. Models such as transformers and BERT have significantly improved the performance of NLP systems, enabling more accurate and contextually aware language processing.
By leveraging these advanced techniques and understanding the applications of NLP, researchers and developers can create more sophisticated and effective language processing tools.
Excited by What You've Read?
There's more where that came from! Sign up now to receive personalized financial insights tailored to your interests.
Stay ahead of the curve - effortlessly.