Machine Learning Market Manipulation And Collusion On Capital Markets Why The Black Box Matters
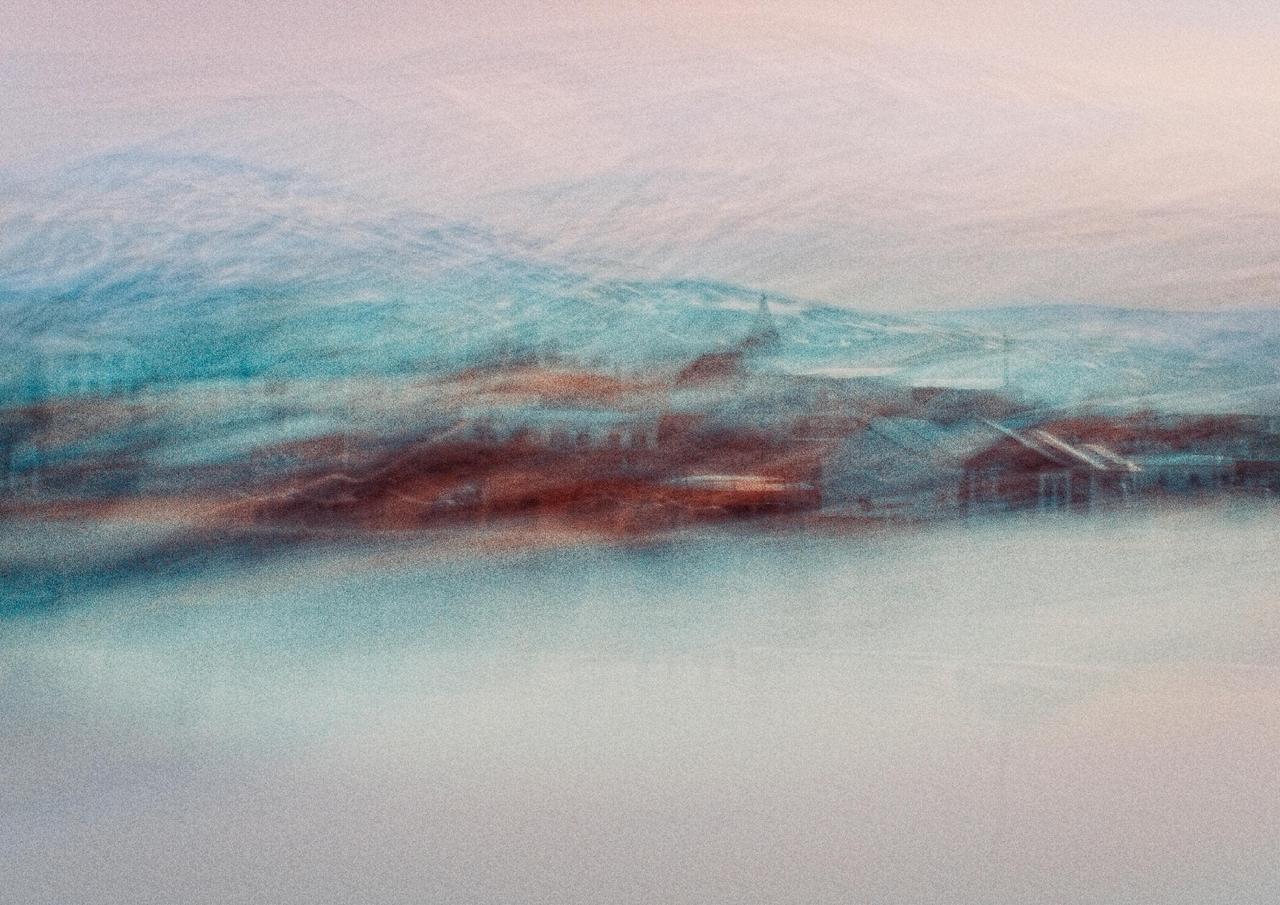
Machine learning techniques have introduced complexities in market manipulation and collusion within capital markets, primarily due to the “black box” nature of these algorithms. In market manipulation, machine learning models can exploit patterns and anomalies in trading data to create or exacerbate price distortions. These models, which often operate without transparency, can obscure the intentions behind trading strategies, making it challenging to detect and address manipulative behavior. The lack of transparency in machine learning algorithms means that their decision-making processes are not easily understood, which complicates the enforcement of regulatory measures and the identification of collusive practices. This opacity can allow unethical practices to persist, affecting market integrity and investor trust.
Black Box Implications
Issue | Description |
---|---|
Transparency | Machine learning algorithms are often opaque, hindering oversight. |
Detection Difficulty | Hard to identify manipulative strategies due to complex algorithms. |
Regulatory Challenges | Difficult to enforce regulations and detect collusion. |
Market Integrity | Risk of eroded trust and increased market volatility. |
Key Insight
“The ‘black box’ nature of machine learning in financial markets complicates the detection and regulation of market manipulation and collusion.”
Introduction to Market Manipulation
Definition and Types of Market Manipulation
Market manipulation refers to activities designed to deceive or mislead investors by artificially affecting the supply or demand for securities, thereby distorting market prices. Common forms of market manipulation include:
- Pump and Dump: Promoting a stock to inflate its price and then selling off shares at the higher price.
- Spoofing: Placing large orders to create a false impression of demand or supply, only to cancel them before execution. Historical examples of market manipulation demonstrate its pervasive and damaging impact on market integrity.
Overview of Capital Markets
Capital markets encompass the venues where savings and investments are channeled between suppliers (investors) and those in need (companies, governments). They include stock markets, bond markets, and other financial exchanges. Key participants include individual investors, institutional investors, brokers, and market makers. Capital markets are vital for economic growth, providing liquidity and enabling capital formation.
Role of Technology in Capital Markets
Technological advancements have significantly transformed capital markets, particularly through the rise of algorithmic trading and high-frequency trading (HFT). These technologies have enhanced market efficiency and liquidity but have also introduced new challenges, such as increased volatility and the potential for sophisticated market manipulation.
Understanding Machine Learning in Capital Markets
Basics of Machine Learning
Machine learning (ML) is a subset of artificial intelligence (AI) that enables systems to learn from data and improve over time without explicit programming. Key concepts include:
- Supervised Learning: Learning from labeled data to make predictions.
- Unsupervised Learning: Identifying patterns in unlabeled data.
- Reinforcement Learning: Learning through trial and error to maximize rewards. In finance, ML is used for predictive analytics, risk management, and developing trading strategies.
Machine Learning Models in Trading
ML models are employed to predict price movements, optimize trading strategies, and execute trades at high speeds. High-frequency trading leverages these models to capitalize on market inefficiencies. While ML models offer significant advantages, such as improved accuracy and speed, they also come with limitations, including model complexity and the risk of overfitting.
The Black Box Problem
The black box problem refers to the lack of transparency and interpretability in ML models. These models often operate as opaque systems, making it challenging to understand how decisions are made. This opacity can obscure manipulative behaviors and complicate regulatory oversight, posing a significant risk to market integrity.
Machine Learning and Market Manipulation
Potential for Market Manipulation
ML can be exploited for market manipulation through techniques such as spreading fake news or manipulating sentiment analysis algorithms to influence stock prices. Case studies reveal instances where ML-driven strategies have been used to manipulate markets, highlighting the sophistication of these schemes.
Detection and Prevention
Detecting market manipulation involves analyzing trading patterns and using surveillance systems equipped with ML algorithms. Regulatory bodies like the SEC and FINRA play a crucial role in monitoring and preventing manipulative activities. However, the complexity and speed of ML-driven manipulation present significant challenges for detection and enforcement.
Ethical and Legal Implications
The ethical use of ML in capital markets necessitates adherence to principles of fairness, transparency, and accountability. Legal frameworks must evolve to address the challenges posed by ML, ensuring compliance and protecting market participants. Financial institutions have a responsibility to implement robust controls and ensure ethical practices in deploying ML technologies.
Machine Learning and Collusion
Understanding Collusion in Capital Markets
Collusion involves secret agreements between market participants to manipulate markets for mutual benefit, such as price fixing or information sharing. Historical examples, such as the LIBOR scandal, underscore the severe impact of collusion on market fairness and integrity.
Role of Machine Learning in Facilitating Collusion
ML can facilitate collusion through algorithms that enable coordinated trading behaviors or information sharing without explicit communication. Detection of such collusive activities requires sophisticated surveillance tools capable of identifying subtle patterns indicative of collusion.
Regulatory and Surveillance Challenges
Identifying collusion is inherently challenging due to its covert nature. Current regulatory frameworks often lack the tools to detect and prove collusion effectively. Innovations in surveillance technology, including the use of AI and ML, are essential to enhance detection capabilities and prevent collusion.
Importance of Transparency and Accountability
The Need for Transparency in Machine Learning Models
Transparent ML models enhance trust and accountability, enabling regulators and stakeholders to understand decision-making processes. Techniques such as explainable AI (XAI) aim to increase model interpretability, providing insights into how models arrive at their predictions.
Accountability in the Use of Machine Learning
Establishing accountability mechanisms involves implementing internal controls, regular audits, and adherence to ethical standards. Financial institutions must adopt best practices to ensure responsible use of ML, fostering a culture of integrity and compliance.
Future Directions and Recommendations
Advancements in explainable AI and collaborative efforts between regulators and industry stakeholders are crucial for addressing the challenges posed by ML. Long-term strategies should focus on developing robust regulatory frameworks, fostering innovation in surveillance technology, and promoting ethical standards in ML applications.
Ensuring Market Integrity in the Age of Machine Learning
Summary of Key Points
Machine learning (ML) offers transformative potential for enhancing trading strategies and improving market efficiency. However, its application also introduces significant risks of market manipulation and collusion, exacerbated by the opaque nature of ML models, known as the black box problem. Addressing these challenges requires a focus on transparency, accountability, and proactive regulatory measures.
Final Thoughts
The rapid advancement of technology in capital markets necessitates continuous adaptation and vigilance. Ensuring market integrity amidst the rise of ML involves concerted efforts from regulators, financial institutions, and technology developers to create a secure, fair, and transparent trading environment.
Additional Resources
For further exploration of machine learning and market regulation:
- Recommended Readings: Books and articles on AI in finance, regulatory frameworks, and ethical considerations.
- Online Courses: Educational platforms offering courses on machine learning, financial regulation, and market ethics.
- Key Reports and Publications: Insights from financial regulatory bodies such as the SEC, FINRA, and international organizations like the Financial Stability Board.
Excited by What You've Read?
There's more where that came from! Sign up now to receive personalized financial insights tailored to your interests.
Stay ahead of the curve - effortlessly.