Machine Learning And Reinforcement Learning In Finance Specialization
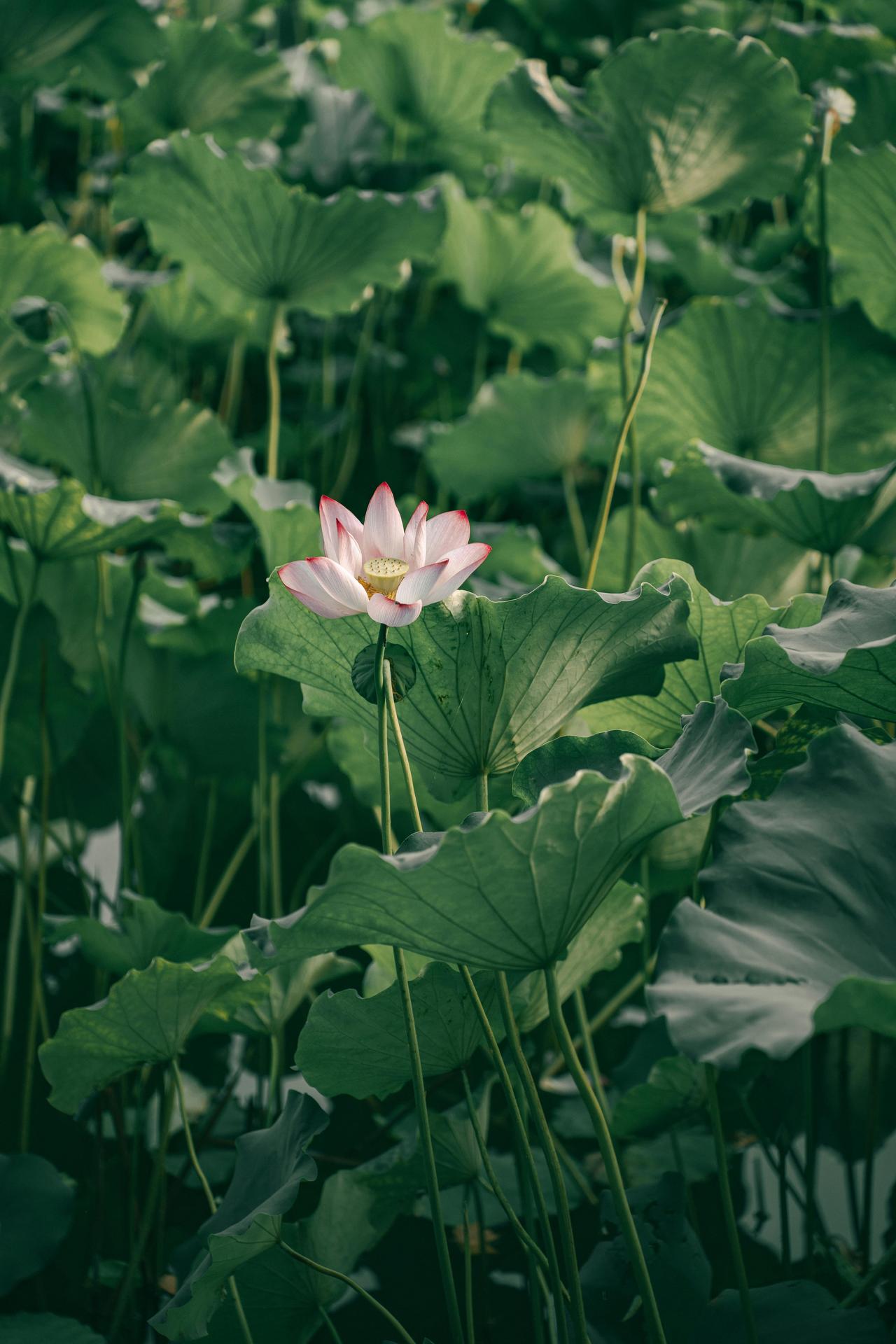
The integration of machine learning and reinforcement learning in finance specialization represents a significant advancement in how financial markets are analyzed and managed. In recent years, the application of these advanced computational techniques has transformed various aspects of finance, from risk management to algorithmic trading. Machine learning, with its ability to analyze vast amounts of data and identify patterns that may be invisible to traditional models, is particularly valuable in predicting market trends and making data-driven investment decisions.
Machine learning algorithms, such as supervised and unsupervised learning, are employed to develop predictive models that can forecast stock prices, assess credit risk, and optimize portfolio allocation. These models utilize historical data and various financial indicators to generate insights that aid in decision-making processes. For example, machine learning techniques can enhance the accuracy of credit scoring models by incorporating a broader range of variables and learning from new data patterns over time.
Reinforcement learning, on the other hand, is a subset of machine learning where algorithms learn optimal strategies through trial and error, receiving rewards or penalties based on their actions. In the context of finance, reinforcement learning can be used to develop sophisticated trading strategies that adapt to changing market conditions. By continually learning from market interactions, these models can optimize trading decisions, manage risk, and maximize returns in a dynamic environment.
The field of finance specialization that focuses on machine learning and reinforcement learning integrates these approaches to create robust financial models and strategies. This specialization involves a deep understanding of both financial theory and advanced computational techniques. Practitioners in this area leverage machine learning algorithms to analyze complex financial datasets and use reinforcement learning to refine decision-making processes and trading strategies.
Overall, machine learning and reinforcement learning in finance specialization enable more accurate and adaptive financial modeling, providing significant advantages in risk management, investment strategy development, and market analysis.
Machine learning has revolutionized finance by enabling advanced predictive analytics and optimization strategies. In finance, machine learning models analyze vast amounts of data to identify patterns, forecast market trends, and optimize investment strategies. Reinforcement learning (RL), a subset of machine learning, is particularly notable for its application in finance due to its ability to make decisions based on evolving data and rewards.
Machine Learning Applications in Finance
Predictive Analytics and Forecasting
Machine learning enhances predictive analytics in finance by leveraging algorithms that process historical data to predict future market trends. Techniques such as supervised learning are used to forecast stock prices, interest rates, and other financial indicators with high accuracy. These models improve decision-making by identifying patterns and correlations that traditional methods might miss.
Risk Management and Fraud Detection
In risk management, machine learning models analyze credit scores, transaction histories, and other variables to assess the risk associated with financial products or investments. Additionally, anomaly detection algorithms are employed to identify unusual transactions, helping to prevent fraud and ensure financial security. These applications enhance the robustness and efficiency of financial risk management.
Reinforcement Learning in Finance
Optimization of Trading Strategies
Reinforcement learning is used to optimize trading strategies by continuously learning and adapting to market conditions. RL algorithms, such as Q-learning and deep reinforcement learning, train trading agents to make decisions that maximize returns while minimizing risks. This adaptive approach allows for more sophisticated and responsive trading strategies.
Portfolio Management and Asset Allocation
Reinforcement learning also plays a significant role in portfolio management and asset allocation. RL models help in dynamically adjusting asset allocations based on market conditions and performance metrics. This results in more effective management of investment portfolios, aligning asset distribution with risk tolerance and investment goals.
Techniques and Tools
Supervised vs. Unsupervised Learning
Machine learning techniques in finance can be broadly categorized into supervised and unsupervised learning. Supervised learning, involving algorithms like regression and classification, relies on labeled data to make predictions. Unsupervised learning, including clustering and dimensionality reduction, is used to discover hidden patterns and relationships within data without predefined labels.
Deep Learning and Neural Networks
Deep learning, a subset of machine learning, employs neural networks to model complex financial systems. Techniques such as convolutional neural networks (CNNs) and recurrent neural networks (RNNs) are used to analyze time-series data and financial texts, providing deeper insights and more accurate forecasts.
Future Trends
Integration of AI and Big Data
The integration of artificial intelligence (AI) with big data is expected to further advance machine learning applications in finance. AI-driven models will increasingly utilize large datasets to improve predictions, enhance decision-making, and uncover new opportunities. This trend promises to enhance the precision and efficiency of financial analysis.
Ethical Considerations and Regulation
As machine learning becomes more prevalent in finance, ethical considerations and regulatory frameworks will play a critical role. Ensuring transparency, fairness, and accountability in AI-driven financial systems will be essential to maintaining trust and compliance. Ongoing research and dialogue will help address these challenges and shape the future of machine learning in finance.
Key References
- “Machine Learning for Asset Management” – This resource explores various machine learning techniques and their applications in asset management.
- “Reinforcement Learning in Finance” – A comprehensive study of how reinforcement learning is applied to financial decision-making and strategy optimization.
Excited by What You've Read?
There's more where that came from! Sign up now to receive personalized financial insights tailored to your interests.
Stay ahead of the curve - effortlessly.