Investment Science And Finance Analytics Lihkg
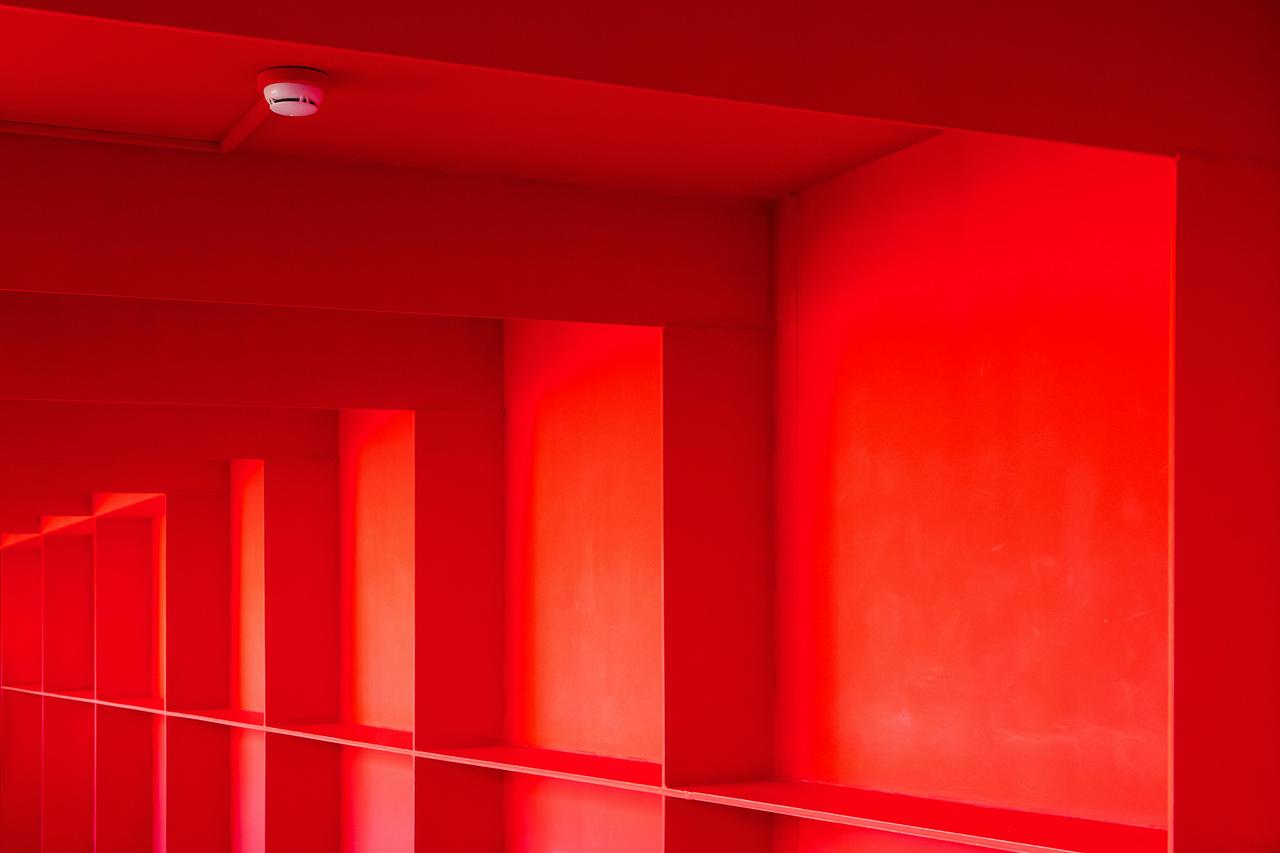
Investment science and finance analytics are crucial fields that leverage quantitative methods and data analysis to guide investment decisions and financial strategies. The term “investment science and finance analytics lihkg” likely pertains to discussions or resources on platforms such as LIHKG, a popular Hong Kong-based online forum, where users engage in detailed discussions about financial and investment topics. Investment science focuses on applying mathematical models, statistical techniques, and computational methods to understand and optimize investment strategies. This includes portfolio management, risk assessment, and asset valuation, all aimed at maximizing returns while managing risk.
Finance analytics, on the other hand, involves using data-driven approaches to analyze financial markets, trends, and individual investments. This encompasses techniques such as financial modeling, algorithmic trading, and predictive analytics to make informed investment decisions. By integrating these analytics into investment science, practitioners can enhance their ability to forecast market movements, evaluate investment opportunities, and implement strategies that align with their financial goals.
In the context of “investment science and finance analytics lihkg,” the focus would be on how these advanced methodologies are discussed and utilized within the community of LIHKG users. This could involve sharing insights, analyzing market data, or discussing various investment techniques and tools that utilize investment science and finance analytics principles. The platform likely serves as a venue for enthusiasts and professionals to exchange knowledge, strategies, and trends related to these fields, reflecting the growing importance of data-driven decision-making in finance and investment.
Investment science combines finance theory with quantitative methods to guide investment decisions. It encompasses various disciplines, including financial mathematics, statistics, and econometrics, to model and predict market behaviors, optimize portfolios, and assess risk. By applying rigorous analytical techniques, investment science helps in making informed decisions and achieving optimal investment outcomes.
Investment Science Applications
Quantitative Finance Models
Quantitative finance involves using mathematical models to analyze financial markets and securities. These models often rely on stochastic processes and statistical methods to forecast market trends, price derivatives, and evaluate investment strategies. The use of quantitative models has become increasingly important in developing algorithmic trading strategies and managing investment portfolios.
Risk Management Techniques
Effective risk management is a cornerstone of investment science. Techniques such as Value at Risk (VaR), Conditional Value at Risk (CVaR), and stress testing are used to evaluate and manage potential financial losses. These techniques help in understanding the potential impact of various risk factors on investment portfolios and in developing strategies to mitigate these risks.
Portfolio Optimization Strategies
Portfolio optimization is a key application of investment science, involving the allocation of assets to achieve the best possible return for a given level of risk. Techniques such as the Markowitz Mean-Variance Optimization and the Black-Litterman model are used to construct efficient portfolios that balance risk and return. These methods rely on historical data and predictive models to make informed investment decisions.
“Investment science applies advanced mathematical and statistical techniques to optimize investment strategies and manage risks effectively.”
Key Investment Science Concepts
Efficient Market Hypothesis
The Efficient Market Hypothesis (EMH) asserts that financial markets are “informationally efficient,” meaning that asset prices reflect all available information. This concept implies that it is impossible to consistently achieve returns that exceed average market returns without assuming additional risk. Understanding EMH is crucial for developing investment strategies and assessing market efficiency.
Behavioral Finance Insights
Behavioral finance explores how psychological factors and cognitive biases influence investor behavior and market outcomes. Insights from behavioral finance challenge the assumptions of traditional finance theories and provide a more nuanced understanding of market anomalies and investor decision-making processes.
Machine Learning in Finance
Machine learning has become an integral part of investment science, enabling the analysis of large datasets and the development of predictive models. Techniques such as supervised learning, unsupervised learning, and reinforcement learning are used to identify patterns, forecast market movements, and optimize trading strategies.
Tools and Resources
Financial Analytics Software
Various financial analytics software tools are available to support investment science. These tools offer functionalities such as data analysis, risk assessment, and portfolio management. Examples include Bloomberg Terminal, MATLAB, and Python libraries like pandas and NumPy.
Academic Research and Publications
Staying updated with academic research and publications in investment science is essential for understanding new developments and methodologies. Journals such as the Journal of Finance and the Review of Financial Studies publish research on various aspects of investment science and finance analytics.
By integrating these advanced techniques and concepts, investment science enhances the ability to make data-driven investment decisions and manage financial risks effectively.
Excited by What You've Read?
There's more where that came from! Sign up now to receive personalized financial insights tailored to your interests.
Stay ahead of the curve - effortlessly.