Integrating AI and Machine Learning in Credit Scoring Models
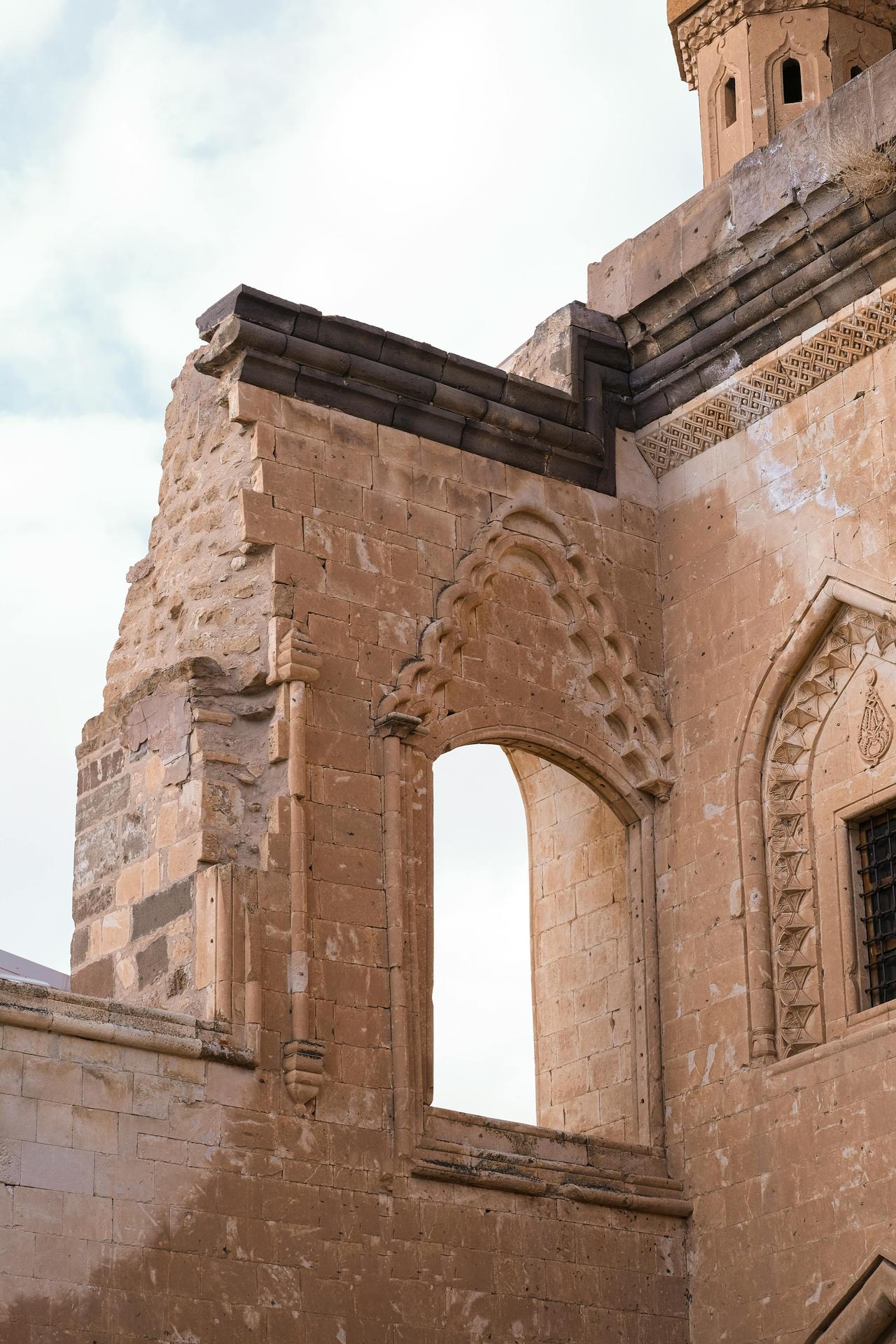
The integration of Artificial Intelligence (AI) and Machine Learning (ML) in credit scoring models represents a significant advancement in the field of financial risk management. These technologies offer new ways to analyze data, predict outcomes, and make more informed decisions. In this article, we explore how AI and ML are revolutionizing credit scoring models, discussing their benefits, methodologies, applications, challenges, and future prospects.
Revolutionizing Credit Scoring with AI and ML
AI and ML technologies have transformed traditional credit scoring methods by introducing advanced data processing capabilities and predictive analytics. This evolution has significant implications for risk assessment in the financial sector.
Enhancing Traditional Credit Scoring Models
Traditional credit scoring models often rely on historical financial data, such as credit history, income, and existing debts. AI and ML can enhance these models by incorporating a wider range of data points and identifying complex patterns that may not be evident through traditional methods.
Improving Risk Assessment Accuracy
AI and ML algorithms can process large volumes of data to make more accurate predictions about creditworthiness. By analyzing diverse data sources, these technologies can provide a more holistic view of a borrower’s financial behavior, leading to improved risk assessment accuracy.
Methodologies in AI and ML for Credit Scoring
AI and ML methodologies in credit scoring involve various techniques and algorithms that are designed to improve the prediction accuracy and efficiency of credit risk assessments.
Machine Learning Algorithms
Machine learning algorithms, such as decision trees, random forests, and neural networks, are used to analyze credit data. These algorithms learn from historical data to identify patterns and relationships that are indicative of credit risk.
Data Processing and Analysis
AI and ML technologies are adept at processing and analyzing both structured and unstructured data. This includes traditional credit data, as well as alternative data sources like social media activity, online behavior, and even psychometric assessments.
Applications in the Financial Industry
The application of AI and ML in credit scoring is not limited to traditional banking. It extends to various areas in the financial industry, offering innovative solutions for credit risk management.
Expanding Access to Credit
AI and ML models can assess creditworthiness more accurately for individuals with limited credit history. This can expand access to credit for underserved populations, including small businesses and individuals in developing economies.
Real-time Credit Decisioning
AI and ML enable real-time credit decisioning, allowing lenders to process loan applications more quickly and efficiently. This speed and efficiency are particularly valuable in online lending platforms and fintech companies.
Challenges in Implementing AI and ML Models
Despite their potential, the integration of AI and ML in credit scoring models faces several challenges that need to be addressed to ensure their effective and ethical use.
Data Privacy and Security
The use of extensive data in AI and ML models raises concerns about data privacy and security. Ensuring the protection of sensitive personal and financial information is crucial to maintaining consumer trust and complying with regulatory requirements.
Bias and Fairness
There is a risk of bias in AI and ML models, especially if the training data is not representative or contains historical biases. Ensuring fairness and eliminating discrimination in credit scoring models is a significant challenge.
Future of AI and ML in Credit Scoring
The future of AI and ML in credit scoring looks promising, with continuous advancements and innovations shaping the field.
Integration with Emerging Technologies
The integration of AI and ML with other emerging technologies, such as blockchain and the Internet of Things (IoT), could further enhance credit scoring models. These technologies offer additional data sources and improved security features.
Evolving Regulatory Landscape
As AI and ML in credit scoring become more prevalent, the regulatory landscape is likely to evolve to address the associated challenges and risks. This will involve developing standards and guidelines to ensure the responsible use of AI and ML in credit assessments.
Conclusion
The integration of AI and Machine Learning in credit scoring models marks a significant step forward in financial risk management. These technologies offer the potential to make credit scoring more accurate, efficient, and inclusive. However, realizing this potential requires overcoming challenges related to data privacy, security, bias, and regulatory compliance. As the technology continues to evolve, it will be crucial for the financial industry to stay abreast of these developments, ensuring that credit scoring models are both effective and ethical. This evolution represents not just a technological advancement, but a fundamental shift in how financial risk is assessed and managed.
Excited by What You've Read?
There's more where that came from! Sign up now to receive personalized financial insights tailored to your interests.
Stay ahead of the curve - effortlessly.