Innovations in Monte Carlo Simulation Techniques for Risk Assessment
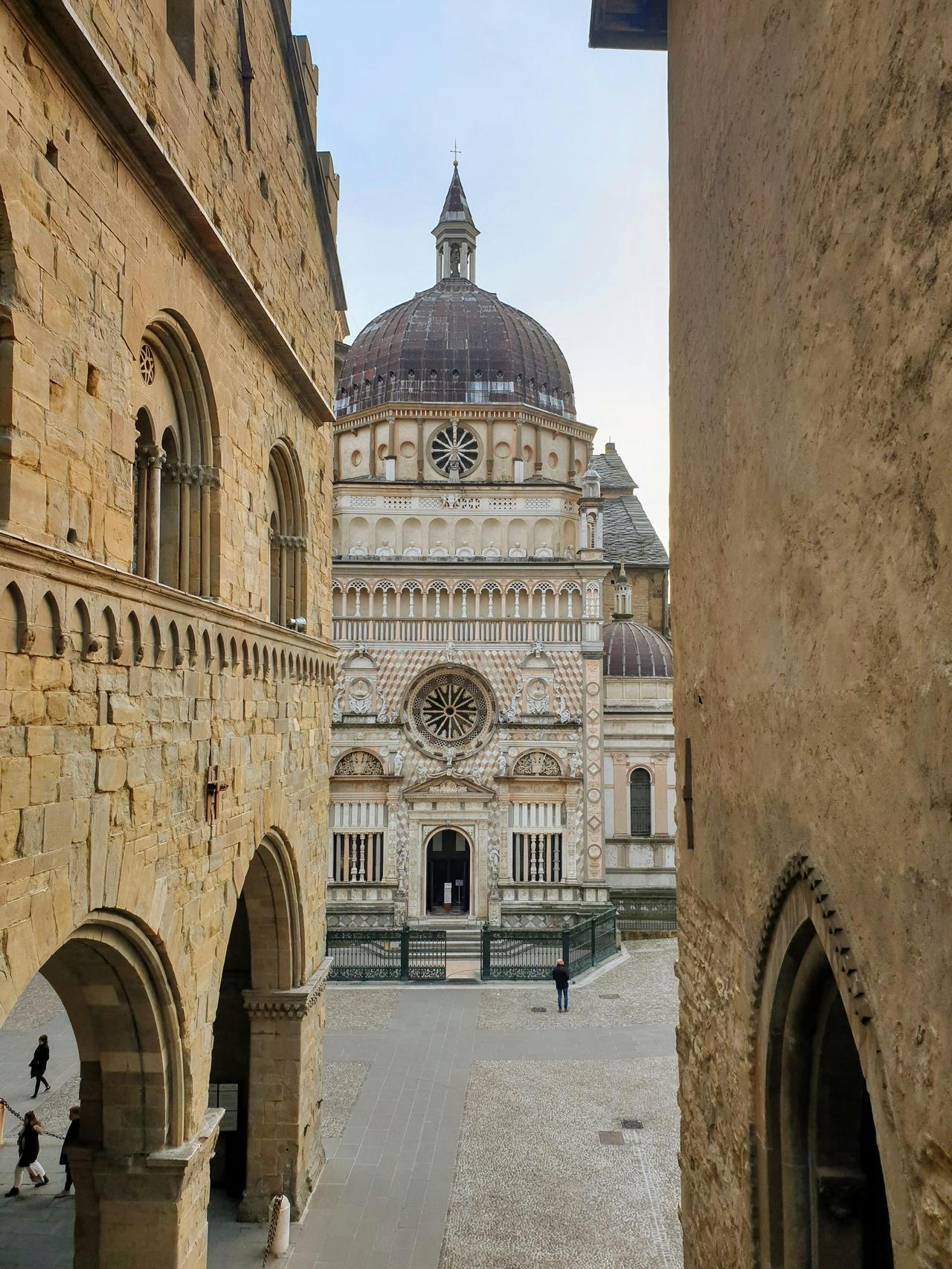
Risk management is an essential aspect of both financial and operational planning in organizations. The use of Monte Carlo simulation techniques has revolutionized the field of risk assessment, offering more accurate and comprehensive insights into potential risks and their impacts. This article delves into the latest innovations in Monte Carlo simulation techniques, exploring how they enhance traditional risk management practices.
The Evolution of Monte Carlo Simulation in Risk Management
Monte Carlo simulations have been a part of risk assessment for decades, providing a way to model the probability of different outcomes in complex, uncertain systems. This technique uses randomness to solve problems that might be deterministic in principle.
Historical Context and Development
Originating in the 1940s, Monte Carlo simulations were first used for scientific and engineering applications. Over time, their application in financial risk management has grown, becoming an indispensable tool for analysts and decision-makers. This evolution mirrors the increasing complexity of the business environment and the need for more sophisticated risk assessment methods.
Key Principles of Monte Carlo Simulation
The fundamental principle of Monte Carlo simulation in risk assessment is its use of random sampling to obtain numerical results. This process involves running simulations many times over, with varying random inputs, to model the probability of different outcomes. This allows for a more nuanced understanding of risk in scenarios where outcomes are uncertain.
Advancements in Computational Techniques
The efficacy of Monte Carlo simulations in risk assessment has greatly benefited from advancements in computational technology. Faster processors and more sophisticated software have made these simulations more accessible and efficient.
Leveraging High-Performance Computing
With the advent of high-performance computing, Monte Carlo simulations can now process large volumes of data at unprecedented speeds. This has significantly reduced the time required for complex risk assessments, enabling real-time risk analysis in some cases.
Integration with Big Data and Analytics
The integration of Monte Carlo simulations with big data analytics has opened new frontiers in risk assessment. This combination allows organizations to analyze vast datasets, uncovering hidden patterns and correlations that inform risk management decisions.
Monte Carlo Simulations in Financial Risk Management
In financial risk management, Monte Carlo simulations have become a cornerstone technique, particularly in the areas of market risk, credit risk, and operational risk assessment.
Application in Market Risk Analysis
Monte Carlo simulations are extensively used in market risk analysis to model and predict changes in market prices and rates. This application is crucial for portfolio management, derivative pricing, and financial planning.
Credit Risk Evaluation
Credit risk evaluation has also benefited from Monte Carlo techniques. These simulations help in assessing the probability of default and the potential impact on an investment portfolio, aiding in more informed decision-making regarding credit issuance and management.
Monte Carlo Simulations in Operational Risk Assessment
Operational risk assessment is another area where Monte Carlo simulations have proved invaluable, especially in sectors like manufacturing, healthcare, and energy.
Modeling Supply Chain Risks
Monte Carlo simulations help model risks in supply chains, such as delays, disruptions, and cost variations. This modeling is essential for strategic planning and contingency management in complex supply chains.
Assessing Risks in Project Management
In project management, these simulations are used to assess risks related to project timelines, costs, and resource allocation, providing project managers with a probabilistic understanding of potential project outcomes.
Future Directions and Challenges
As Monte Carlo simulation techniques continue to evolve, they face both opportunities and challenges in the realm of risk management.
Integration with AI and Machine Learning
The integration of AI and machine learning with Monte Carlo simulations represents a significant advancement, offering the potential for more accurate, adaptive, and intelligent risk assessment models.
Addressing Computational Complexity and Cost
Despite technological advancements, Monte Carlo simulations can still be computationally intensive and costly. Ongoing research and development are focused on making these simulations more efficient and accessible for a wider range of organizations.
In conclusion, Monte Carlo simulation techniques represent a significant innovation in the field of risk management. Their ability to model complex, uncertain systems provides invaluable insights for decision-makers. As computational technologies continue to advance, these techniques will likely become even more integral to effective risk management strategies.
Excited by What You've Read?
There's more where that came from! Sign up now to receive personalized financial insights tailored to your interests.
Stay ahead of the curve - effortlessly.