Forecasting Volatilities Of Oil And Gas Assets A Comparison Of Gas Garch And Egarch Models
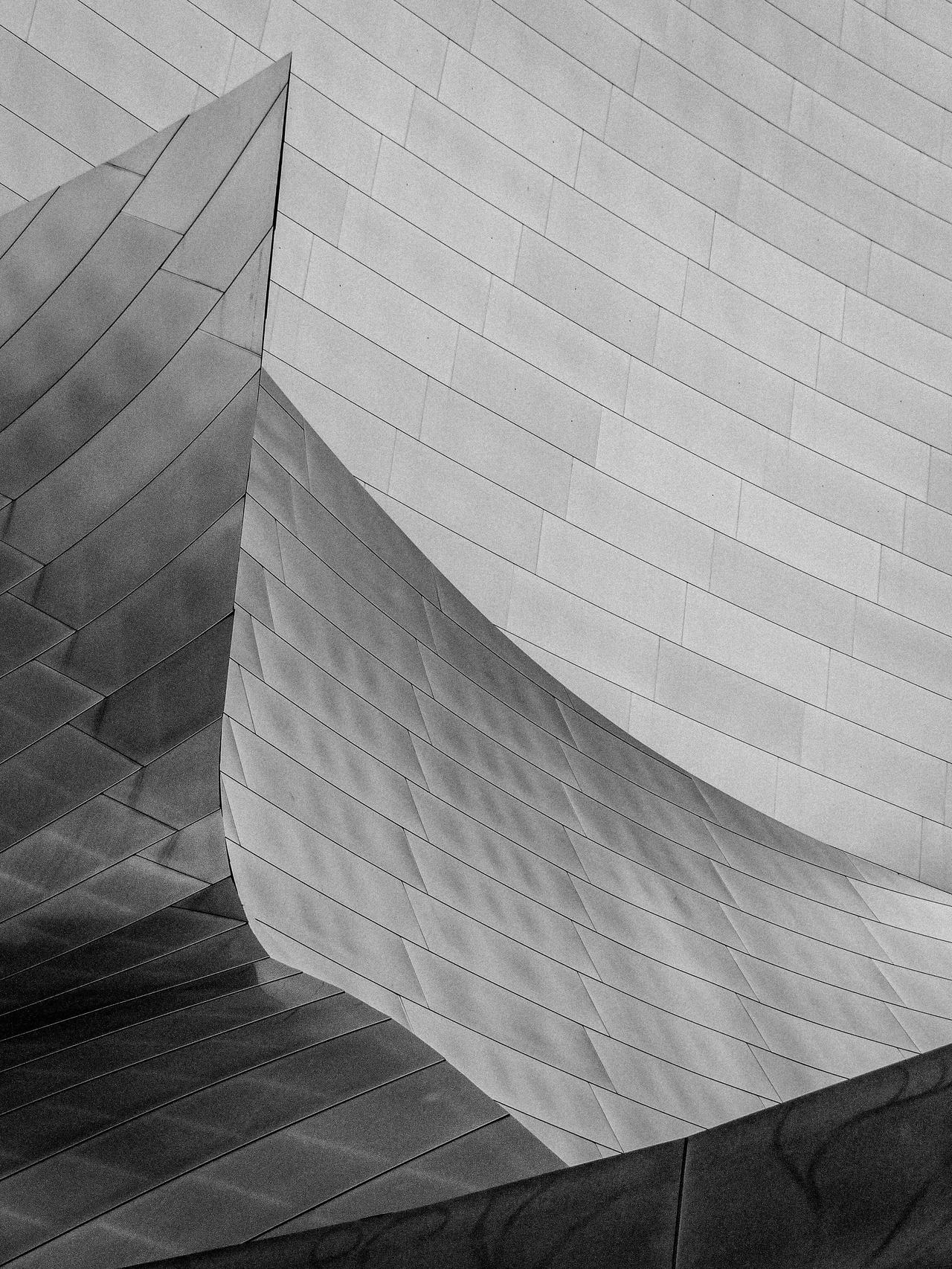
Forecasting the volatilities of oil and gas assets is a crucial aspect of financial and risk management in the energy sector, given the inherent fluctuations in these markets. The exact phrase “forecasting volatilities of oil and gas assets a comparison of GARCH and EGARCH models” refers to an analytical approach used to evaluate and compare different volatility forecasting models. GARCH (Generalized Autoregressive Conditional Heteroskedasticity) and EGARCH (Exponential Generalized Autoregressive Conditional Heteroskedasticity) are two prominent models in this domain, each offering distinct advantages for predicting market volatility.
The GARCH model, developed by Tim Bollerslev, is widely used for its ability to model volatility clustering, a common phenomenon where high-volatility periods are followed by more high-volatility periods and low-volatility periods by low-volatility periods. This model helps in understanding and predicting future volatility by considering past squared returns and past volatility. However, the standard GARCH model has limitations in capturing the asymmetric effects of volatility, where negative shocks might have a different impact compared to positive shocks of the same magnitude.
On the other hand, the EGARCH model, introduced by Tim Bollerslev and Robert Engle, addresses this limitation by incorporating asymmetry into the volatility forecasting process. The EGARCH model allows for a more nuanced understanding of volatility dynamics by modeling how different types of market shocks influence volatility, making it particularly useful for assets with pronounced asymmetric responses to market events. This model uses the natural logarithm of the conditional variance, which ensures that the forecasted volatility remains positive and allows for capturing both the magnitude and direction of volatility changes more accurately.
When comparing the GARCH and EGARCH models for forecasting the volatilities of oil and gas assets, it is essential to consider factors such as the specific characteristics of the asset returns, the presence of asymmetric effects, and the overall model fit. The comparison typically involves assessing the models’ performance through various statistical measures and out-of-sample forecasts to determine which model provides better predictions for the volatile energy markets. Such analysis aids investors and risk managers in making informed decisions based on more accurate volatility forecasts, thereby enhancing their strategies and risk management practices.
Forecasting involves predicting future values based on historical data and statistical models. In the context of financial assets, such as oil and gas, accurate forecasting of volatilities is crucial for risk management and investment strategies. Various models are employed to forecast asset volatilities, each with its strengths and limitations. Among these, the Generalized Autoregressive Conditional Heteroskedasticity (GARCH) models and their extensions, such as Exponential GARCH (EGARCH), are frequently used to estimate and predict volatility.
Comparing GARCH and EGARCH Models
GARCH Model Overview
The Generalized Autoregressive Conditional Heteroskedasticity (GARCH) model, introduced by Tim Bollerslev in 1986, extends the Autoregressive Conditional Heteroskedasticity (ARCH) model by incorporating past conditional variances. It is widely used to model and forecast volatility due to its ability to capture volatility clustering—a phenomenon where high-volatility periods are followed by more high-volatility periods and vice versa.
The GARCH(p, q) model is defined as:
$$ \sigma^2_t = \alpha_0 + \sum_{i=1}^p \alpha_i \epsilon^2_{t-i} + \sum_{j=1}^q \beta_j \sigma^2_{t-j} $$where $\sigma^2_t$ is the conditional variance, $\epsilon_t$ is the error term, and $\alpha$ and $\beta$ are parameters to be estimated.
EGARCH Model Overview
The Exponential GARCH (EGARCH) model, proposed by Daniel Nelson in 1991, improves on the GARCH model by allowing for asymmetric effects of shocks on volatility. This model is particularly useful in capturing the leverage effect, where negative shocks impact volatility more than positive shocks of the same magnitude. The EGARCH(p, q) model is expressed as:
$$ \log(\sigma^2_t) = \alpha_0 + \sum_{i=1}^p \alpha_i \left( \frac{\epsilon_{t-i}}{\sigma_{t-i}} - \mathbb{E} \left[\frac{\epsilon_{t-i}}{\sigma_{t-i}}\right] \right) + \sum_{j=1}^q \beta_j \log(\sigma^2_{t-j}) $$where $\log(\sigma^2_t)$ is the log of the conditional variance, capturing both the size and sign of shocks.
Model Performance Comparison
Forecasting Accuracy
When comparing the forecasting accuracy of GARCH and EGARCH models, empirical studies often show that EGARCH models outperform GARCH models in capturing asymmetric volatility effects. This makes EGARCH particularly valuable for assets with significant leverage effects, such as oil and gas.
Risk Management Implications
In risk management, the choice between GARCH and EGARCH can affect the estimation of Value at Risk (VaR) and other risk metrics. EGARCH’s ability to account for asymmetric responses in volatility can lead to more accurate risk assessments and better-informed decision-making.
Mathjax: Forecasting Volatility Metrics
To evaluate the performance of volatility forecasts, metrics such as Mean Squared Error (MSE) can be used:
$$ \text{MSE} = \frac{1}{N} \sum_{t=1}^N (\hat{\sigma}^2_t - \sigma^2_t)^2 $$where $\hat{\sigma}^2_t$ is the forecasted volatility, $\sigma^2_t$ is the actual volatility, and $N$ is the number of observations.
References
- “Forecasting Volatilities of Oil and Gas Assets: A Comparison of GARCH and EGARCH Models” – Provides a detailed analysis of the effectiveness of different volatility models.
- “Advanced Volatility Forecasting Techniques” – A comprehensive review of methods used in forecasting financial volatility.
This comparison highlights the importance of selecting the appropriate model for accurate volatility forecasting and effective risk management in financial markets.
Excited by What You've Read?
There's more where that came from! Sign up now to receive personalized financial insights tailored to your interests.
Stay ahead of the curve - effortlessly.