Forecasting Housing Prices Based On Historical Data Is An Example Of Anomaly Detection
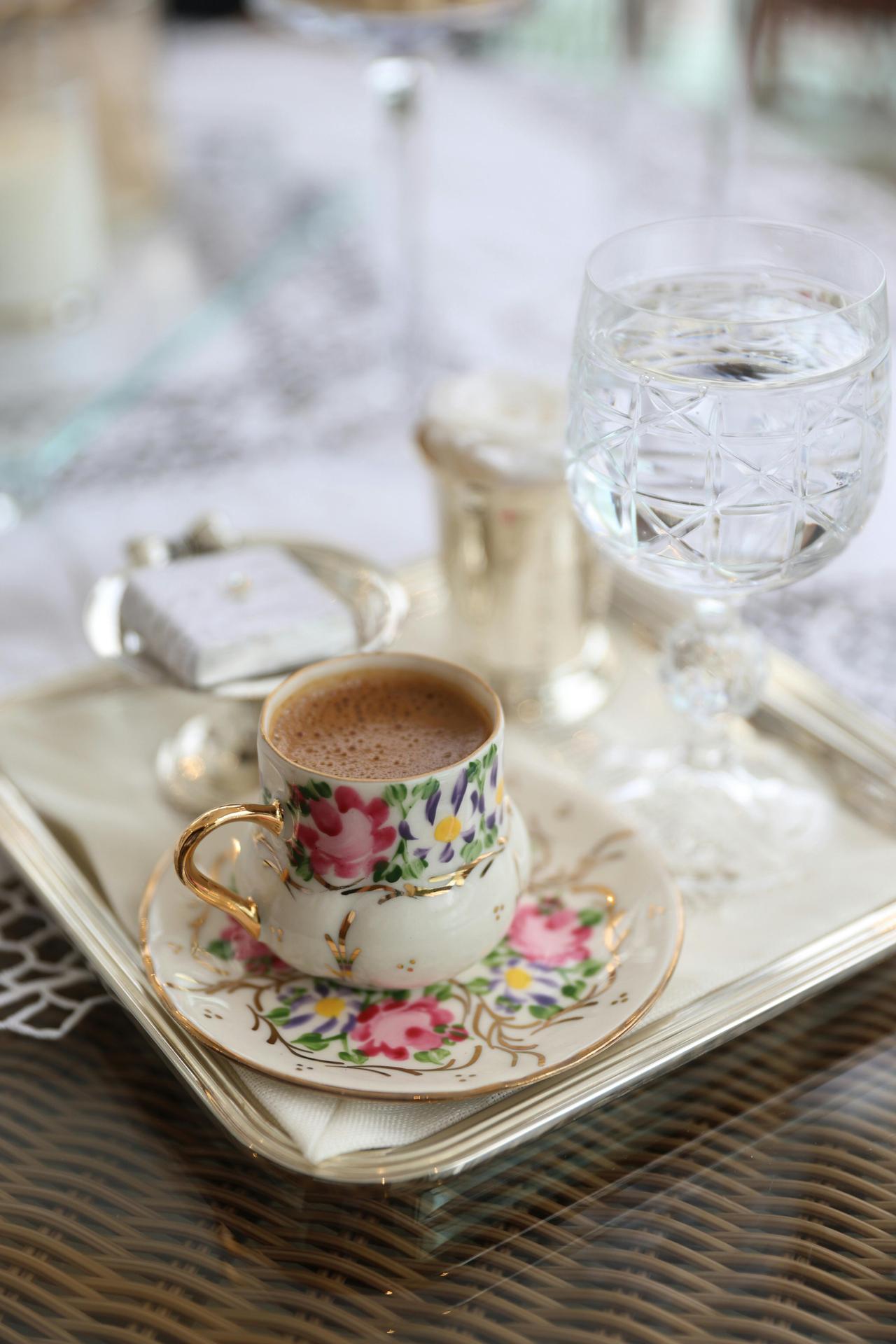
Forecasting housing prices involves analyzing historical data to predict future trends in the real estate market. This process typically employs statistical and machine learning techniques to model past price movements and make future predictions. When we say “forecasting housing prices based on historical data is an example of anomaly detection,” we are referring to the use of these forecasting techniques to identify unusual patterns or deviations from the norm within the housing market.
In this context, anomaly detection is a crucial component. Anomalies, or outliers, in housing price data might include sudden spikes or drops in property values that do not fit the historical trend. By applying forecasting models, analysts can predict expected price trends based on past data. When actual prices deviate significantly from these predictions, it signals a potential anomaly. For instance, if a property in a particular neighborhood shows a drastic price increase that is inconsistent with historical trends and other local data, this could indicate an anomaly. Such deviations may be caused by various factors, including changes in the local economy, new developments, or shifts in demand and supply.
Using historical data for anomaly detection in housing prices helps investors, real estate agents, and policymakers understand underlying market dynamics and respond appropriately. For example, detecting anomalies can prompt further investigation into whether a sudden price change is due to an emerging market trend or an isolated event. Additionally, identifying these anomalies can improve the accuracy of forecasting models by highlighting areas where historical data may not fully capture current market conditions.
In summary, “forecasting housing prices based on historical data is an example of anomaly detection” illustrates how historical data analysis can be used not only to predict future trends but also to identify significant deviations from expected price patterns, aiding in more informed decision-making within the housing market.
Forecasting involves predicting future values based on historical data and analytical models. In various fields, such as finance, economics, and real estate, forecasting helps in making informed decisions by analyzing past trends and patterns. The accuracy of forecasts depends on the quality of historical data and the suitability of the forecasting model used. By understanding historical patterns, analysts can provide insights into future trends and potential anomalies.
Forecasting Housing Prices with Historical Data
Forecasting housing prices using historical data is a common application of predictive modeling. This process involves analyzing past housing market trends, including price changes, supply and demand dynamics, and economic indicators. Models such as time series analysis and regression analysis are used to predict future housing prices based on historical trends. Accurate forecasting helps buyers, sellers, and investors make informed decisions in the real estate market.
Anomaly Detection in Price Forecasting
Anomaly detection is crucial in forecasting as it helps identify unusual patterns or outliers that may indicate significant changes or errors in the data. In the context of housing price forecasting, anomaly detection can reveal unexpected fluctuations or deviations from historical trends. Techniques such as statistical analysis, machine learning algorithms, and visualization tools are employed to detect anomalies and improve the reliability of forecasts.
Comparison of Forecasting Models
Here is a table comparing different forecasting models used in housing price predictions:
Model | Description | Advantages | Disadvantages |
---|---|---|---|
Time Series Analysis | Uses historical data points to predict future values | Captures trends and seasonality | May not account for external factors |
Regression Analysis | Models the relationship between price and other variables | Can include various influencing factors | Assumes linear relationships |
Machine Learning | Utilizes algorithms to predict prices based on complex patterns | Can handle non-linear relationships | Requires extensive data and tuning |
Quote: “Effective forecasting of housing prices requires a robust model that can incorporate historical trends and detect anomalies to provide accurate predictions.”
Mathematical Foundations of Forecasting Models
To illustrate the concepts of forecasting models, consider the following mathematical representations:
- Time Series Forecasting:
where \(Y_t\) represents the forecasted value at time \(t\), \(\alpha\) and \(\beta\) are parameters, and \(\epsilon_t\) is the error term.
- Regression Analysis:
where \(P\) is the housing price, \(X_1\) and \(X_2\) are predictor variables, \(\alpha\), \(\beta\), and \(\gamma\) are coefficients, and \(\epsilon\) is the error term.
These formulas help in understanding how historical data is used to predict future values and how anomalies are detected to enhance forecasting accuracy.
Excited by What You've Read?
There's more where that came from! Sign up now to receive personalized financial insights tailored to your interests.
Stay ahead of the curve - effortlessly.