Decoding Market Complexity Through Quantitative Analysis
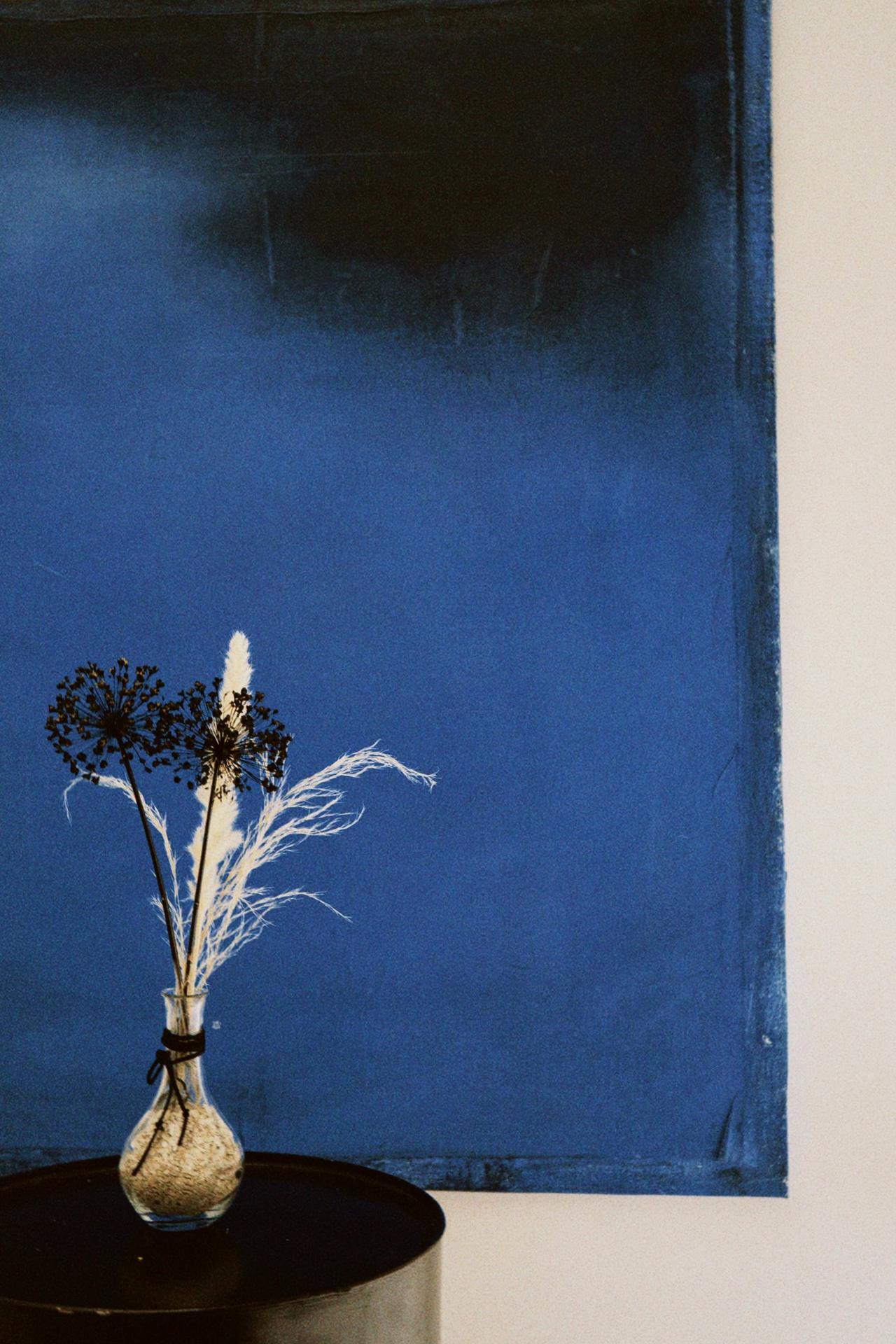
Investment analysis methods are essential tools for investors and financial analysts to understand and navigate the intricacies of the financial markets. Quantitative analysis, in particular, plays a vital role in deciphering the complexities of the market by applying mathematical and statistical techniques. This article explores the various facets of quantitative analysis in investment decisions.
The Role of Quantitative Analysis in Investment
Quantitative analysis uses mathematical and statistical modeling to understand and predict financial behavior. This analytical approach is grounded in the belief that accurate mathematical models can decipher market trends and investor behaviors.
Data-Driven Decision Making
Quantitative analysis allows investors to make decisions based on hard data rather than intuition. By analyzing historical data, investors can identify trends, patterns, and anomalies that would not be visible otherwise. This method helps in reducing biases and emotions in investment decisions.
Risk Quantification
One of the key aspects of quantitative analysis is the ability to quantify risk. By using statistical models, investors can estimate the probability of different outcomes, allowing for a more nuanced understanding of the risks involved in various investment opportunities.
Financial Modeling Techniques
Financial modeling is a cornerstone of quantitative analysis, enabling analysts to create abstract representations of financial situations.
Discounted Cash Flow (DCF) Models
DCF models are used to estimate the value of an investment based on its expected future cash flows. These models are central to equity valuation and help investors determine whether a stock is over or undervalued based on present values of future cash flows.
Monte Carlo Simulations
Monte Carlo simulations are used to model the probability of different outcomes in a process that cannot easily be predicted due to the intervention of random variables. This method is particularly useful in assessing risk and uncertainty in financial forecasting and decision making.
Statistical Analysis in Market Predictions
Statistical analysis is used to interpret data and identify patterns and trends. It plays a critical role in predicting market movements and assessing investment strategies.
Regression Analysis
Regression analysis helps in understanding the relationships between different financial variables. It is used extensively in predicting stock prices, analyzing economic trends, and assessing the impact of various factors on investment returns.
Time Series Analysis
Time series analysis involves analyzing a series of data points ordered in time. In financial markets, this method is used to analyze historical data such as stock prices or interest rates to predict future trends.
Algorithmic Trading Strategies
Algorithmic trading involves using algorithms to make trade decisions, submit orders, and manage those orders after submission. This method is heavily reliant on quantitative analysis.
High-Frequency Trading (HFT)
High-frequency trading is a type of algorithmic trading that processes a large number of orders at very high speeds. These strategies use complex algorithms to analyze multiple markets and execute orders based on market conditions.
Quantitative Trading Models
Quantitative trading models are developed to identify trading opportunities. They can be based on a variety of strategies, including statistical arbitrage, trend following, and mean reversion.
Risk Management and Quantitative Analysis
Effective risk management is crucial in investment decision-making. Quantitative analysis offers robust tools for managing and mitigating risk.
Value at Risk (VaR)
Value at Risk is a widely used risk management tool in finance. It provides an estimate of the maximum potential loss over a specified time period for a given confidence interval.
Stress Testing
Stress testing involves simulating extreme market conditions to assess the resilience of an investment portfolio. It helps in understanding the potential impact of adverse market conditions on investment performance.
Ethical Considerations in Quantitative Analysis
While quantitative analysis provides powerful tools for investment analysis, it also raises ethical considerations that must be addressed.
Data Integrity and Privacy
The reliance on extensive data raises concerns about data integrity and privacy. Ensuring the accuracy of data and respecting privacy constraints are crucial in maintaining the ethical standards of quantitative analysis.
Over-reliance on Models
There is a risk of over-reliance on mathematical models in investment decisions. Models are based on historical data and assumptions, and they may not always accurately predict future events. Balancing model-based insights with qualitative analysis is important for well-rounded investment decisions.
In conclusion, quantitative analysis offers a structured and data-driven approach to understanding and predicting financial market behavior. From financial modeling and statistical analysis to algorithmic trading and risk management, it encompasses a broad range of techniques that are essential for modern investment strategies. However, it’s important to balance the quantitative insights with qualitative judgment and to be mindful of the ethical considerations surrounding data use and model reliance. By integrating quantitative analysis effectively into their decision-making process, investors and analysts can navigate the complexities of the markets with greater precision and insight, leading to more informed and potentially successful investment outcomes.
Excited by What You've Read?
There's more where that came from! Sign up now to receive personalized financial insights tailored to your interests.
Stay ahead of the curve - effortlessly.