Data Mining In Course Management Systems Moodle Case Study And Tutorial
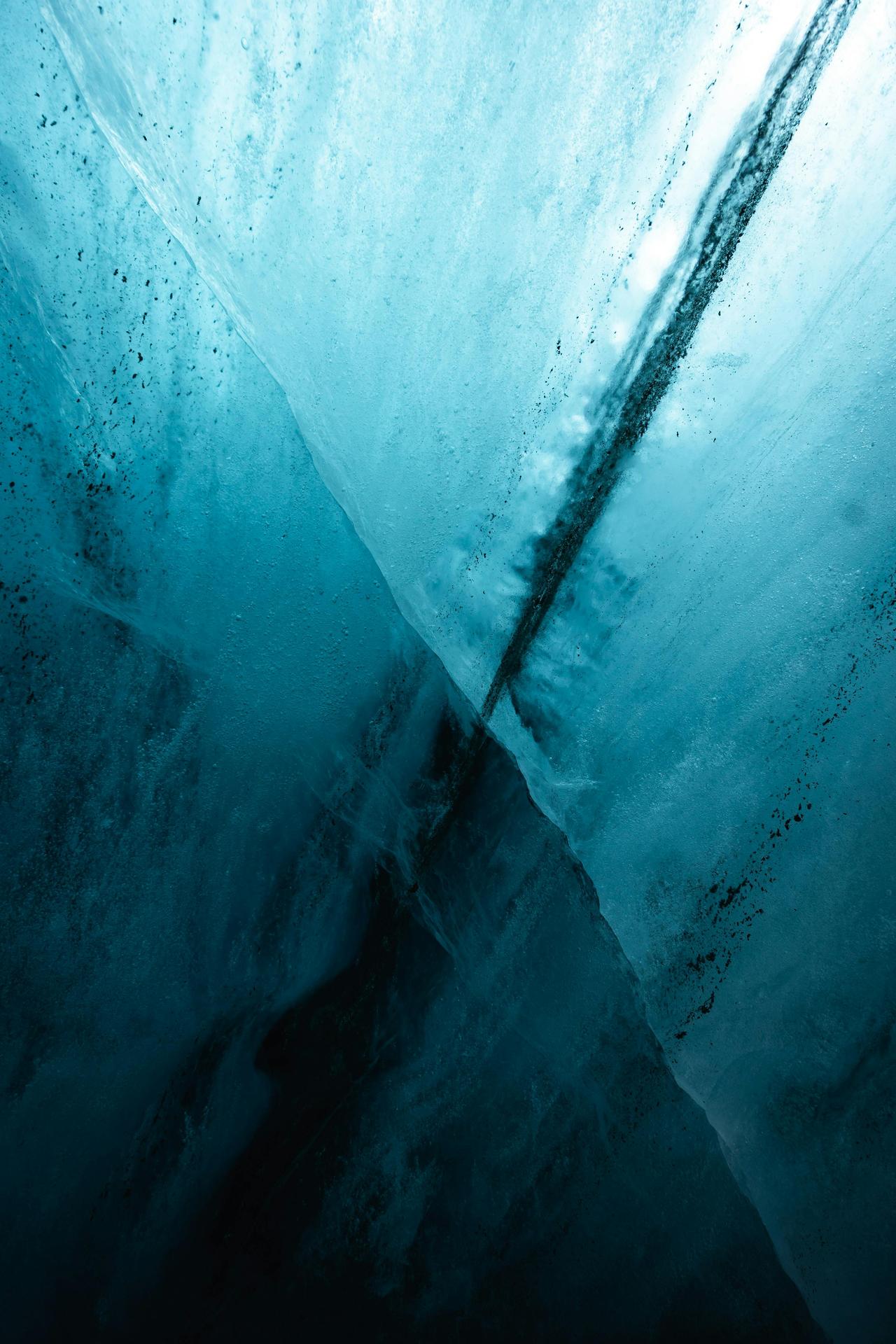
Data mining in course management systems, particularly with platforms like Moodle, is an increasingly important area of study and application. The term “data mining in course management systems Moodle case study and tutorial” refers to the exploration and analysis of data collected from Moodle, a widely used open-source learning management system. This analysis aims to uncover patterns and insights that can improve educational outcomes and enhance the management of courses.
Moodle generates a wealth of data through its various functionalities, including student interactions, assignment submissions, quiz results, and forum discussions. By applying data mining techniques to this data, educators and administrators can identify trends, track student performance, and tailor educational content to better meet the needs of learners. For instance, data mining can reveal which topics students struggle with most, enabling instructors to adjust their teaching strategies accordingly.
A case study involving data mining in Moodle might explore specific instances where data analysis led to significant improvements in course design or student engagement. For example, a case study could detail how mining quiz results and forum activity identified key areas where students needed additional support, leading to targeted interventions that improved overall course effectiveness.
Tutorials on data mining in Moodle guide users through the process of setting up data mining tools, running analyses, and interpreting results. These tutorials often include practical steps for extracting data from Moodle, applying various data mining algorithms, and using visualization tools to present findings. Through these resources, educators and administrators can gain the skills needed to leverage data mining for actionable insights, ultimately enhancing the learning experience and operational efficiency within Moodle-based courses.
Data mining involves the extraction of useful patterns and knowledge from large datasets. It uses algorithms and statistical models to uncover hidden trends, relationships, and insights within data. This process is pivotal in many fields, including business, healthcare, and education, to make informed decisions and predictions.
Data Mining Applications in Education
Course Management Systems and Data Mining
In the context of course management systems like Moodle, data mining techniques are employed to analyze student interactions and performance data. By examining log files, assignment submissions, and forum discussions, educators can identify trends and areas where students may need additional support. Data mining helps in customizing the learning experience and improving educational outcomes.
“Using data mining in course management systems can reveal insights into student engagement and performance, allowing for more targeted interventions.”
Moodle Case Study: Data Mining Insights
A case study on Moodle data mining reveals how data-driven analysis can enhance teaching strategies. For example, analyzing student grades and participation patterns can help instructors adjust course materials and teaching methods to better meet students’ needs. Such insights enable more effective learning environments and improved student retention.
Techniques and Tools for Data Mining
Common Data Mining Techniques
Several techniques are commonly used in data mining, including clustering, classification, and association rule mining. Clustering groups similar data points together, classification assigns data points to predefined categories, and association rule mining identifies relationships between variables. These methods are applied to educational data to uncover useful patterns.
Tools for Data Mining in Moodle
Various tools are available for data mining in Moodle, including plugins and external software. These tools facilitate the extraction and analysis of data from Moodle’s database, providing valuable insights into student behavior and course effectiveness. Popular tools include Moodle’s built-in analytics features and third-party data mining applications.
Challenges and Considerations
Data Privacy and Security
When mining educational data, it’s crucial to address privacy and security concerns. Ensuring that student data is anonymized and protected from unauthorized access is essential to maintain trust and comply with regulations. Data mining practices must adhere to ethical standards and legal requirements.
Data Quality and Accuracy
The quality and accuracy of the data being analyzed can significantly impact the results of data mining. Inaccurate or incomplete data can lead to misleading insights and poor decision-making. Ensuring data integrity and employing robust data cleaning methods are critical steps in the data mining process.
Future Directions and Innovations
Advances in Data Mining Technologies
Advancements in data mining technologies, such as machine learning and artificial intelligence, are driving new possibilities for analyzing educational data. These technologies enable more sophisticated analyses and predictions, enhancing the ability to personalize education and improve student outcomes.
Integrating Data Mining with Learning Analytics
Integrating data mining with learning analytics provides a comprehensive approach to understanding and improving educational processes. By combining data mining techniques with analytics tools, educators can gain deeper insights into student learning patterns and make data-driven decisions to enhance educational practices.
Excited by What You've Read?
There's more where that came from! Sign up now to receive personalized financial insights tailored to your interests.
Stay ahead of the curve - effortlessly.